Optimisation Theory for Neural Networks and Implicit Bias Towards Flat Minima
[CFAR Rising Star Lecture Series]
Optimisation Theory for Neural Networks and Implicit Bias Towards Flat Minima by Prof Atsushi Nitanda
The gradient-based method for training neural networks on over-parameterised neural networks converges to the optimal solution which perfectly fits a given training dataset and generalises a highly non-convex loss landscape. This phenomenon is a result of the development of the neural tangent kernel and mean field theories. These theories translate the optimisation dynamics into functions spaces and exploit the convexity of the objective with respect to its function.
In this presentation, Prof Atsushi Nitanda will briefly introduce his team’s results on both theories. He will also present his on-going work on the implicit bias of the stochastic gradient descent and its averaging variant, the key to understanding why deep learning models work so well.
Optimisation Theory for Neural Networks and Implicit Bias Towards Flat Minima by Prof Atsushi Nitanda
8 Feb 2023 | 11.00am (Singapore Time)
The gradient-based method for training neural networks on over-parameterised neural networks converges to the optimal solution which perfectly fits a given training dataset and generalises a highly non-convex loss landscape. This phenomenon is a result of the development of the neural tangent kernel and mean field theories. These theories translate the optimisation dynamics into functions spaces and exploit the convexity of the objective with respect to its function.
In this presentation, Prof Atsushi Nitanda will briefly introduce his team’s results on both theories. He will also present his on-going work on the implicit bias of the stochastic gradient descent and its averaging variant, the key to understanding why deep learning models work so well.
SPEAKER
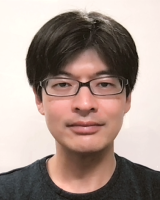
Prof Atsushi Nitanda
Associate Professor
Kyushu Institute of Technology
Associate Professor
Kyushu Institute of Technology
Prof Atsushi Nitanda is an Associate Professor at the Kyushu Institute of Technology. Prior to his current position, he was an Assistant Professor at the University of Tokyo, where he received his Ph.D. in Information Science and Technology in 2018. Previously, he worked at NTT DATA Mathematical Systems Inc. (MSI) as a researcher after receiving his master’s degree in Mathematical Sciences from the University of Tokyo in 2009. Prof Nitanda’s research interests include stochastic optimisation, kernel method and deep learning. In 2021, he received the Outstanding Paper Award at ICLR and the Dean’s Awards from the University of Tokyo in 2018 and 2009.
A*STAR celebrates International Women's Day
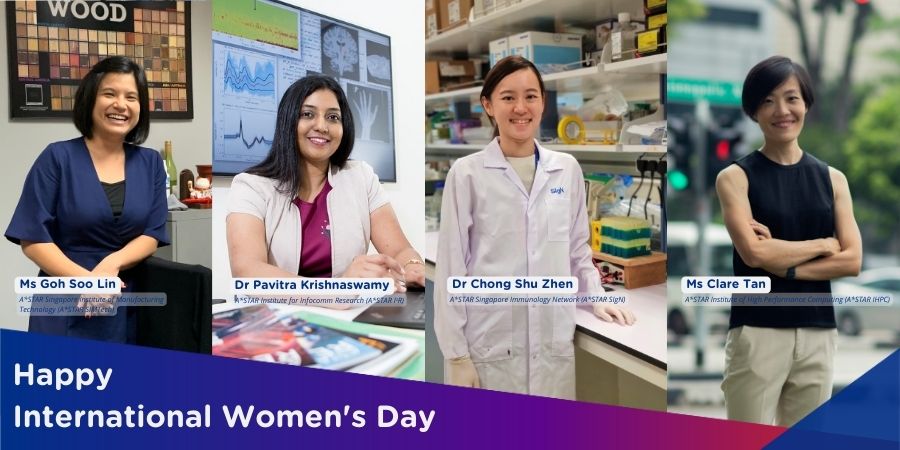
From groundbreaking discoveries to cutting-edge research, our researchers are empowering the next generation of female science, technology, engineering and mathematics (STEM) leaders.
Get inspired by our #WomeninSTEM