DATA-DRIVEN PREDICTIVE MAINTENANCE AND OPTIMAL PLAN* (40 HOURS)
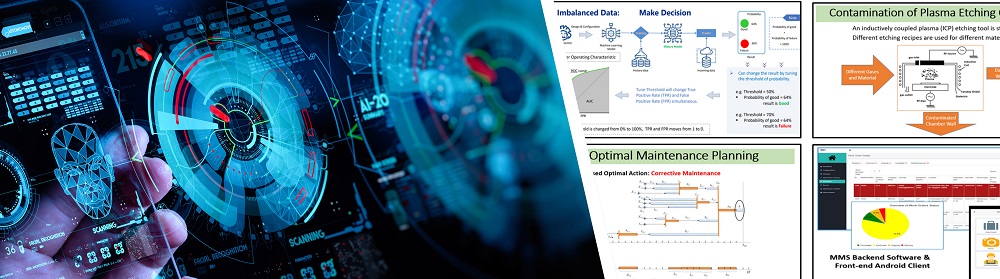
- This programme is in collaboration with the Sectoral AI Centre of Excellence for Manufacturing (AIMfg).
Introduction
Effective management of maintenance in industries is a major concern to reduce the cost and ensure the reliable operation of
high-value equipment/machines. With modern complex complicated equipment and time pressure from production, a data-driven decision-making approach with timely support from relevant data is crucial for generating optimal plans and cost-effective maintenance.
However, data is normally diverse and scattered around everywhere in the shopfloor, and needs to be collated with their time-stamp first. To get insights from data for improving efficiency and quality of maintenance, profound data analysis knowledge, techniques and skills are needed.
about the programme
This course aims to provide participants with knowledge, techniques and skills in data collection, analysis for predictive maintenance and optimal maintenance planning. Data from machine sensors, operation management systems and maintenance activities are analyzed during the training sessions. The latest technology, for example, machine learning-based predictive engines and maintenance planning systems, will be introduced to participants for applications on industrial cases and gain hands-on experience.
At the end of the course, participants can:
- Understand the basic concepts and issues in data analysis
- Identify imbalanced data classes
- Perform data processing effectively
- Understand the concept of data-driven predictive maintenance
- Apply data analysis techniques for predictive maintenance modelling
- Leverage the predictive model for optimal maintenance planning
Who should attend
This course is recommended for operations and maintenance managers and engineers, equipment designers, process managers and engineers, software analysts, machine, line or cell system integrators, project managers and other professionals in all industries where there is a need for machine health condition analysis including precision engineering, electronics, aerospace, marine engineering, renewable energy, MedTech, and remanufacturing.
Contact Us
- For technical enquiries, please contact:
Dr Ian CHAN,
Email: hlchan@SIMTech.a-star.edu.sg
Ms Su Myat Phyoe ,
Email: su_myat_phyoe@SIMTech.a-star.edu.sg
- For general enquiries, please contact:
Mr Kevin ZHENG,
Email: kevin_zheng@SIMTech.a-star.edu.sg
Registration
- Please register for this course through our Course Registration Form for Public Classes.
- For the first question, please select "Modular Programmes".
- Applicants will be placed on our waiting list if the course does not have an upcoming scheduled intake.
- Once the next intake is confirmed to commence, SIMTech will contact the applicants to share the class information.
Schedule
Module | Skills Course Reference Number | Next Intake(s)'s Training Period
(Click on the dates to view its schedules) | Registration Status |
| TGS-2020504648 | The next intake's schedule is still in the planning stage. |
Note: SIMTech and ARTC reserve the right to change the class/schedule/course fee or any details about the course without prior notice to the participants.
Announcement:
- From 1 Oct 2023, attendance-taking for SkillsFuture Singapore (SSG)'s funded courses must be done digitally via the Singpass App. More information may be viewed here.




- View the full list of modular programmes offered by SIMTech and ARTC.
A*STAR celebrates International Women's Day
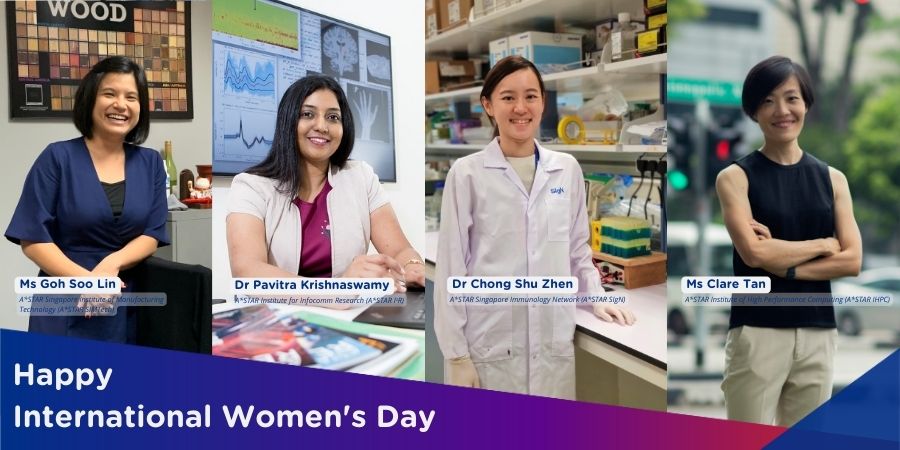
From groundbreaking discoveries to cutting-edge research, our researchers are empowering the next generation of female science, technology, engineering and mathematics (STEM) leaders.
Get inspired by our #WomeninSTEM