AI IN MANUFACTURING
Artificial Intelligence has been widely adopted by companies in the manufacturing sector. AI-powered applications in the manufacturing environment spans across remote maintenance, predictive and prescriptive maintenance, production and supply chain optimisation, and decision-support tools to streamline processes across the value chain.
AI tools also support research in areas related to manufacturing but can be applied to other sectors too – for example, material research, resource planning and optimisation, waste minimisation and process control. AI is also being used to increase the resilience of our manufacturing systems with simulation research to assist in “what-if” scenario analysis and managing uncertainties in an increasingly dynamic and complex manufacturing environment.
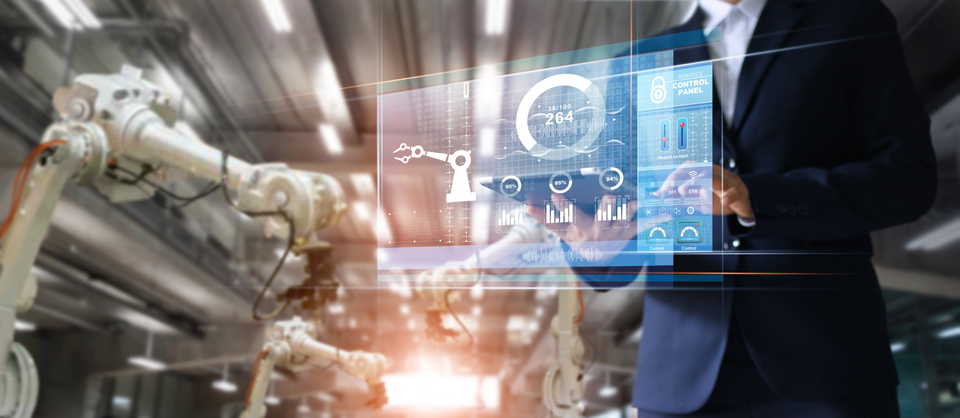
Key Researchers
Dr Wang Wei, A*STAR Singapore Institute of Manufacturing Technology (A*STAR SIMTech) Dr Tan Puay Siew, A*STAR Singapore Institute of Manufacturing Technology (A*STAR SIMTech)
Dr Joel Tay, A*STAR Singapore Institute of Manufacturing Technology (A*STAR SIMTech)
Dr Tran Van Tung, A*STAR Singapore Institute of Manufacturing Technology (A*STAR SIMTech) Dr Ernest Tan, A*STAR Advanced Remanufacturing and Technology Centre (A*STAR ARTC)
Dr Tan Teck Leong, A*STAR Institute of High Performance Computing (A*STAR IHPC) Dr Li Xiaoli, A*STAR Institute for Infocomm Research (A*STAR I2R)
Key Projects
A*STAR celebrates International Women's Day
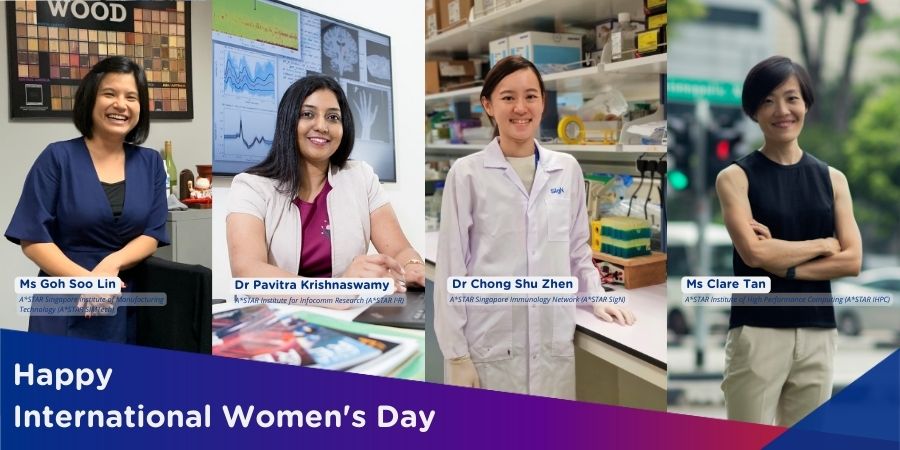
From groundbreaking discoveries to cutting-edge research, our researchers are empowering the next generation of female science, technology, engineering and mathematics (STEM) leaders.
Get inspired by our #WomeninSTEM