Paving the way for Human-Robot collaboration
Imagine a world where robots work seamlessly alongside humans, just like the futuristic visions of "Star Wars," "The Jetsons," "Big Hero 6," and "Astro Boy." In this utopian vision, artificial intelligence has evolved to a point where robots are not just tools but integral members of society, assisting us in our daily tasks, enriching our lives, and contributing to our well-being. While this might seem like science fiction, the reality is that we are taking significant strides towards achieving this dream.
The way to achieve this dream begins with the pursuit of Artificial General Intelligence (AGI), a level of intelligence that matches or exceeds human capabilities across a wide range of tasks. At A*STAR’s Institute for Infocomm Research (I²R), a team of dedicated scientists have embarked on a journey to make AGI a reality, starting with the most fundamental of tasks in the kitchen.
From left to right: Lin Dongyun, Sun Ying, Cheng Yi, Fang Fen, Xu Qianli
A*STAR I²R tops international computer vision challenge 3 years in a row
For three consecutive years, the Institute for Infocomm Research (I²R) took part in the EPIC-KITCHENS Challenge. It is a unique challenge that compels researchers worldwide to work with entirely unscripted datasets. It comprises of over 100 hours of HD recordings capturing all daily activities in the kitchen, 20 million image frames, 90,000 distinct actions, and 20,000 unique narrations across multiple languages.
I²R emerged 1st place for the Unsupervised Domain Adaptation (UDA) for Action Recognition category, from 2021 to 2023 and topped the newly launched Hand Object Segmentation (HOS) category in 2023.
Other contenders include global universities and research institutes.
AI Challenges for the team
You may wonder how winning the EPIC-KITCHENS challenge helps in creating an AGI or even enables robots to work with humans.
For the robots to assist us in tasks, they must first identify and understand objects and people in images and videos. Known as Computer vision, it traditionally requires learning through scripted datasets such as an image or video that has been designed and created specifically for training computer vision models, where they focus on a specific task, such as action recognition (what is the person doing?) or object recognition (what is the object and where is it?). However, a groundbreaking shift in the world of computer vision research has emerged with the EPIC-KITCHENS challenge where the team works with unscripted datasets.
AI models perform optimally when tested in domains similar to their training data eg: it will perform best if it is tested on videos that are shot in the same factory, with the same lighting condition, same camera setting and same filming style. However, in the real world, environments change, and AI models struggle to adapt. To address this challenge, AI models must be retrained with new, labeled data from the new environment. This process is both labour- intensive and time-consuming.
The UDA challenge posed a double challenge by requiring AI to adapt to different environments with unlabeled data. This endeavor is a pivotal step towards realising the elusive Artificial General Intelligence (AGI) that can adapt to various contexts without the need for extensive resources.
Overcoming these AI Challenges
I²R's continuous success in the EPIC-KITCHENS challenge can be attributed to their innovative approach. In 2021, they adopted a hand-centric approach that first detected hands in video frames to narrow down the area of focus and followed by focusing on the spatiotemporal appearances of hand regions (critical for identifying specific actions) to enhance recognition accuracy.
In 2022, the team incorporated verb-noun pairing knowledge from the data, improving the system's ability to recognise actions. For example, the team taught the system that "stir" does not typically pair with "oven". This adds a level of common sense to the model that we stir ingredients in a bowl or pot, not an oven.
In 2023, they embraced Large Language Models (LLMs), expanding their knowledge base on verb-noun pairing not included in the training data. They further extended their expertise to hand-object interaction – a core technique related to action recognition and undertook the hand-object segmentation (HOS) challenge in the same event.
Leveraging the state-of-the-art technology “Segment Anything Model (SAM)” and their expertise in hand detection, the team secure the leading position on the leaderboard.
The road ahead
The accomplishments in action recognition are part of the ongoing Human-Robot Collaborative AI program, aiming to enable robots to work seamlessly alongside humans. The team now seeks to generalise action recognition algorithms, making them more accessible for various applications such as public security and construction to enhance work safety practices.
• Cheng, Y., Xu, Z., Fang, F., Lin, D., Fan, H., Wong, Y., ... & Kankanhalli, M. (2023). A Study on Differentiable Logic and LLMs for EPIC-KITCHENS-100 Unsupervised Domain Adaptation Challenge for Action Recognition 2023. arXiv preprint arXiv:2307.06569.
• Cheng, Y., Lin, D., Fang, F., Woon, H. X., Xu, Q., & Sun, Y. (2023). Team VI-I2R Technical Report on EPIC-KITCHENS-100 Unsupervised Domain Adaptation Challenge for Action Recognition 2022. arXiv preprint arXiv:2301.12436.
• Cheng, Y., Fang, F., & Sun, Y. (2022). Team vi-i2r technical report on epic-kitchens-100 unsupervised domain adaptation challenge for action recognition 2021. arXiv preprint arXiv:2206.02573.
• Fang, F., Cheng, Y., Sun, Y., & Xu, Q. (2023). Team I2R-VI-FF Technical Report on EPIC-KITCHENS VISOR Hand Object Segmentation Challenge 2023. arXiv preprint arXiv: 2310.20120.
A*STAR celebrates International Women's Day
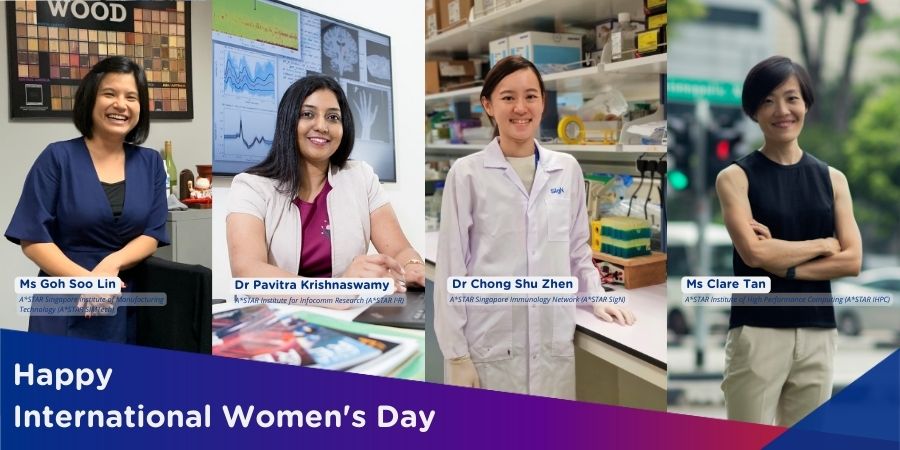
From groundbreaking discoveries to cutting-edge research, our researchers are empowering the next generation of female science, technology, engineering and mathematics (STEM) leaders.
Get inspired by our #WomeninSTEM