Coreference-Aware Dialogue Summarisation
The use of AI to summarise human conversations has gained research traction lately. With large amount of data circulating the digital space, there is a need to develop machine learning algorithms that automatically shorten longer texts and deliver fluently accurate summaries that convey the intended messages.
Researchers must navigate through the complexity of human speech and face challenges such as unstructured information exchanges, informal interaction between speakers, and dynamic role changes as dialogues evolve.
I2R’s Aural & Language team thus explored different approaches to incorporate coreference information in neural abstractive dialogue summarisation models to tackle these challenges. These approaches are :
- GNN-based coreference fusion - a graph-based method that can characterise the underlying structure and facilitate inter-connected relations.
- Coreference-guided attention- a parameter-efficient method that uses attentive re-weighting of contextualised representation.
- Coreference-informed Transformer- a parameter-free method with strategic head probing, selection and manipulation.
Their state-of-the-art approach of using Coreference information (such as “you” and “I”) for the summarising of human conversation were applied onto various neural architectures. It achieved a better ROUGE-1 score as compared to larger summarising models (refer to Table 2). The results on Automatic and human evaluation on factual correctness suggest that such coreference-aware models are better at tracing the information flow among interlocutors and associating accurate status and actions with the corresponding interlocutors and person mentions.
With the astounding results in their research, it opens new possibilities of exploiting coreference for other dialogue related tasks such as, helping AI better understand human language and how humans talk thus increasing the possible interactions between humans and robots. They also won the Best Paper Award at the 22nd Annual Meeting of the Special Interest Group on Discourse and Dialogue. Other submissions for this event include work related to Amazon's Alexa Challenge from Professor Christopher Manning (a highly cited researcher (h>100 according to Google Scholar) from Stanford University).
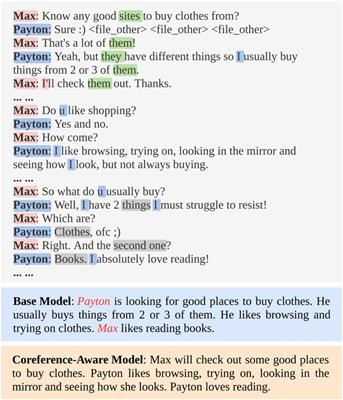
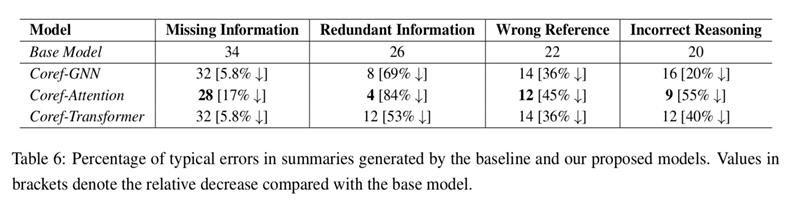
* The A*STAR I2R researchers contributing to this research are Zhengyuan Liu, Ke Shi, Nancy F. Chen
A*STAR celebrates International Women's Day
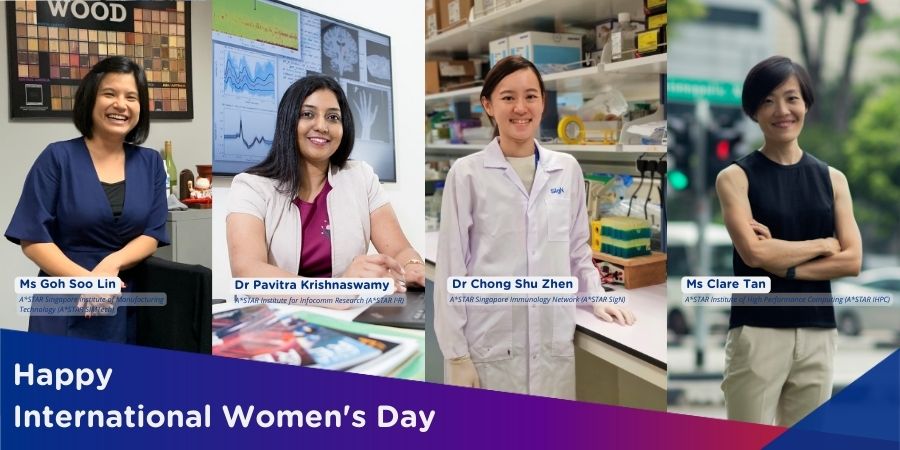
From groundbreaking discoveries to cutting-edge research, our researchers are empowering the next generation of female science, technology, engineering and mathematics (STEM) leaders.
Get inspired by our #WomeninSTEM