Effective management of maintenance in industries is a major concern to reduce the cost and ensure the reliable operation of
high-value equipment/machines. With modern complex complicated equipment and time pressure from production, a data-driven decision-making approach with timely support from relevant data is crucial for generating optimal plans and cost-effective maintenance.
However, data is normally diverse and scattered around everywhere in the shopfloor, and needs to be collated with their time-stamp first. To get insights from data for improving efficiency and quality of maintenance, profound data analysis knowledge, techniques and skills are needed.
about the programme
This course aims to provide participants with knowledge, techniques and skills in data collection, analysis for predictive maintenance and optimal maintenance planning. Data from machine sensors, operation management systems and maintenance activities are analyzed during the training sessions. The latest technology, for example, machine learning-based predictive engines and maintenance planning systems, will be introduced to participants for applications on industrial cases and gain hands-on experience.
At the end of the course, participants can:
- Understand the basic concepts and issues in data analysis
- Identify imbalanced data classes
- Perform data processing effectively
- Understand the concept of data-driven predictive maintenance
- Apply data analysis techniques for predictive maintenance modelling
- Leverage the predictive model for optimal maintenance planning
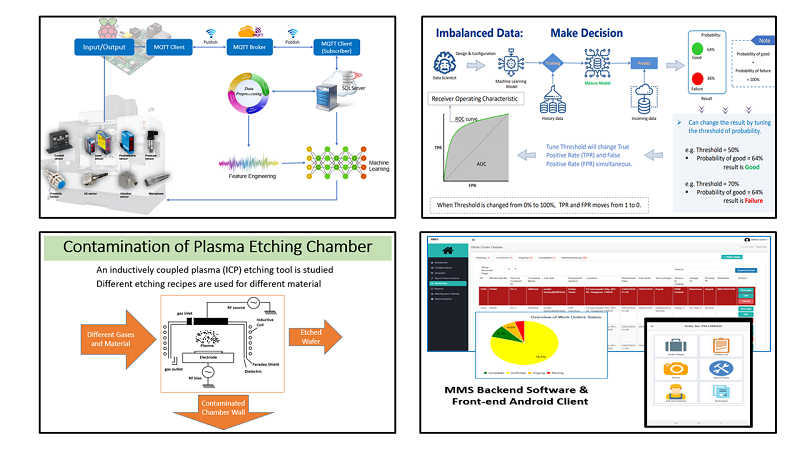
Who should attend
This course is recommended for operations and maintenance managers and engineers, equipment designers, process managers and engineers, software analysts, machine, line or cell system integrators, project managers and other professionals in all industries where there is a need for machine health condition analysis including precision engineering, electronics, aerospace, marine engineering, renewable energy, MedTech, and remanufacturing.