A*STAR NEWS
Balancing Privacy and Personalisation are Key to Take Large Language Models into the Future
By Professor Ivor Tsang
Director, Centre for Frontier AI Research (CFAR), A*STAR
It has only been a year since the debut of ChatGPT, and it has already significantly changed the ways people work, create and communicate. Together with other large language model (LLM) tools, it has played an important role in helping people understand what generative artificial intelligence (AI) is – namely algorithms that can generate or create new content by learning from patterns and structure of data they have been trained on.
OpenAI, the developer of ChatGPT, said that it has expanded its user base to over 100 million weekly active users, setting records for being the fastest-growing consumer application in history. People are certainly sitting up and taking notice of generative AI’s huge potential. Big Tech company Microsoft has cumulatively invested a reported $13 billion in OpenAI and joined the company’s board, while Google is using its own generative AI tools in Gmail, Docs and other widely used products.
Singapore is also looking to develop and deploy impactful AI solutions, including generative AI and LLMs tools. Earlier this month, Singapore unveiled its National AI Strategy (NAIS 2.0), an updated iteration of NAIS which was launched in 2019, initiating pivotal AI initiatives across industry, the government, and research sectors.
The strategy aims to strengthen key aspects in our communities, infrastructure, and environment for better support in AI development. Simultaneously, a S$70 million National Multimodal LLM Programme (NMLP) was announced by Singapore’s Infocomm Media Development Authority (IMDA) in collaboration with AI Singapore (AISG) and the Agency for Science, Technology and Research (A*STAR). The initiative aims to foster innovation in AI on both domestic and regional fronts, enhancing the nation's capabilities in AI research and development while nurturing AI talent.
LLMs are important as they can revolutionise many sectors. Finance professionals could employ them to conduct risk analyses and enhance fraud detection, since the algorithms are adept at detecting and learning from patterns. In the digital economy, LLMs can produce code and assist programmers in software development as well.
While LLMs hold immense potential and are likely to be used very widely, there are hurdles to surmount before wider adoption takes place. Specifically, we need to improve LLMs’ training for specialised fields and enhance data protection measures.
Balancing privacy and personalisation in LLMs
Privacy concerns are a significant issue in the use of LLMs. Sensitive or confidential information can be gathered by LLMs when users input details or pose queries, also called prompts. Moreover, many designers of LLMs rely on users’ prompts to guide and refine the behaviour of their models, particularly in generating results. This means that some LLMs could unintentionally store confidential information, keyed in by users, in databases used to train the LLM models, which could subsequently be revealed as responses to other users' prompts.
In May 2023, Samsung Electronics banned its staff from accessing tools like ChatGPT after finding that some of them had uploaded sensitive code. It noted that the data transmitted to such platforms is stored on external servers, thus difficult to retrieve and delete, and could be disclosed to other users.
To address the privacy concerns, A*STAR’s Institute for Infocomm Research (I²R) has been working on Privacy Preserving Technologies (PPTech) to enable Large Language Models (LLMs) to securely process sensitive datasets, ensuring a secure and privacy-compliant processing environment.
On the other hand, A*STAR’s Centre for Frontier AI Research (CFAR), set up in 2022, scientists are looking into ways to boost LLMs’ user privacy while personalising their output. For example, CFAR scientists are bridging the gap by fine-tuning LLMs with domain-specific prompts, so that they can handle domain-related questions while anonymising user interactions and prompts.
Fine-tuning can broaden LLMs’ appeal in other ways. When a company uses a generic chatbot to interact with customers, its ‘voice’ may seem impersonal or not reflect the business’s brand. Training it with examples of how the firm usually communicates with clients can help ensure it sounds on point. Part of CFAR’s work is in studying how to personalise LLMs’ responses while also balancing privacy concerns.
Advancing education with personalised LLMs
LLM provides great potential for personalisation, for example in educational applications. When children are learning to read aloud, they often need one-on-one just-in-time feedback from teachers, which can be tough for large classes. To bridge this gap, AI can be adopted to help coach students individually.
Dr Nancy Chen and her team at A*STAR’s Institute for Infocomm Research (I2R) have developed an AI tutoring system to promote self-learning. CommonTown, an EdTech company, has deployed the Malay and Tamil versions to schools. The English and Chinese versions have led to a commercial spin-off, nomopai, which support students to prepare for oral examinations and empower companies to improve sales training.
As students read aloud, the AI speech evaluation tutoring system gauges and scores their pronunciation, intonation and fluency. It also gives personalised feedback so that they can improve their speech delivery skills and increase their overall language proficiency. Teachers can also use the feedback from the AI system to tailor their teaching strategies and plans for each individual student.
The I2R researchers are now designing a multimodal AI tutor that will guide children in communicating in our mother tongue languages (Mandarin, Malay and Tamil) under the ‘AI in Education Grand Challenge’ organised by AI SG in partnership with the Ministry of Education (MOE). It will use visual inputs such as pictures to spur conversations with young pupils who are beginning their primary school journey. Such initiatives, along with others, contribute to Singapore's ongoing preservation of linguistic diversity and its bilingual advantage.
The next frontier: long-term sustainability of LLMs
Despite LLMs’ impressive achievements, there are concerns about their environmental sustainability. For LLM models to have greater accuracy based on a larger number of training parameter means more energy consumption by data centres and servers, and as a result increased carbon emission too.
CFAR scientists are exploring the next generation of LLMs with a focus on sustainability. They are studying novel techniques to develop methods to reduce the carbon footprint both during the training and deployment phases. For instance, by reducing the amount of data required to train models, or efficiently adapting existing models during the deployment stage using hardware that perform well while consuming very little power.
The next few years will no doubt see more developments in the technology powering generative AI and LLMs. Useful applications will expand, but so will concerns over privacy, personalisation and sustainability. We hope that what Singapore is doing in its AI strategies and R&D will contribute to tackling these hurdles and help generative AI and LLMs fulfill their promise of being transformational tools for how we live, work and create.
Was This Article Helpful ?
A*STAR celebrates International Women's Day
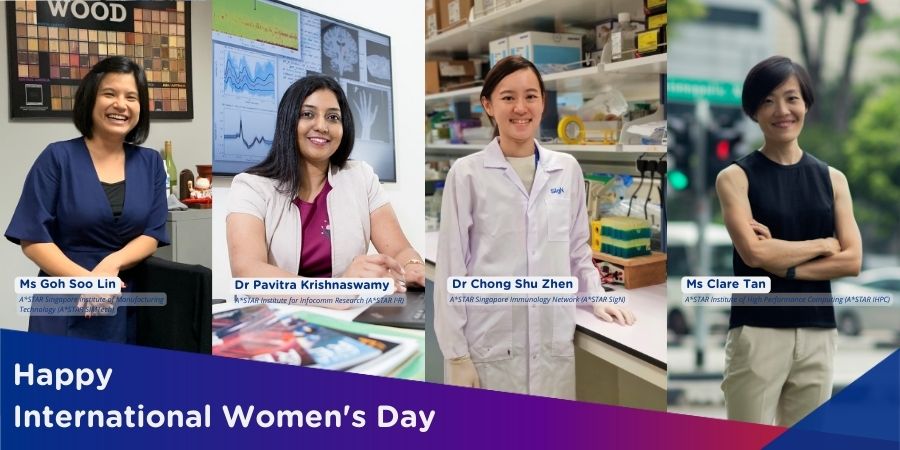
From groundbreaking discoveries to cutting-edge research, our researchers are empowering the next generation of female science, technology, engineering and mathematics (STEM) leaders.
Get inspired by our #WomeninSTEM