Artificial intelligence generated content (AIGC) has attracted a lot of research attention recently. The great success of the text-to-image generation models is partially due to the emergence of the diffusion models. Generally, diffusion models rely on the progressive noising and denoising steps based on the Gaussian distribution. However, in some scenarios, the distribution of the noise is non-Gaussian. In this talk, Dr Liu Jun will introduce two techniques capable of handling non-Gaussian-distribution noise. He will also share about their efficacy in 3D pose estimation and 3D mesh recovery tasks, showcasing the techniques’ state-of-the-art results in these domains.
A*STAR Centre for Frontier AI Research (A*STAR CFAR)
Officially opened in 2022, A*STAR Centre for Frontier AI Research (A*STAR CFAR) aims to advance use-inspired basic research in AI within the broader scientific community of A*STAR.
A*STAR CFAR will focus on the following strategically important fundamental research to develop next generation AI technologies:
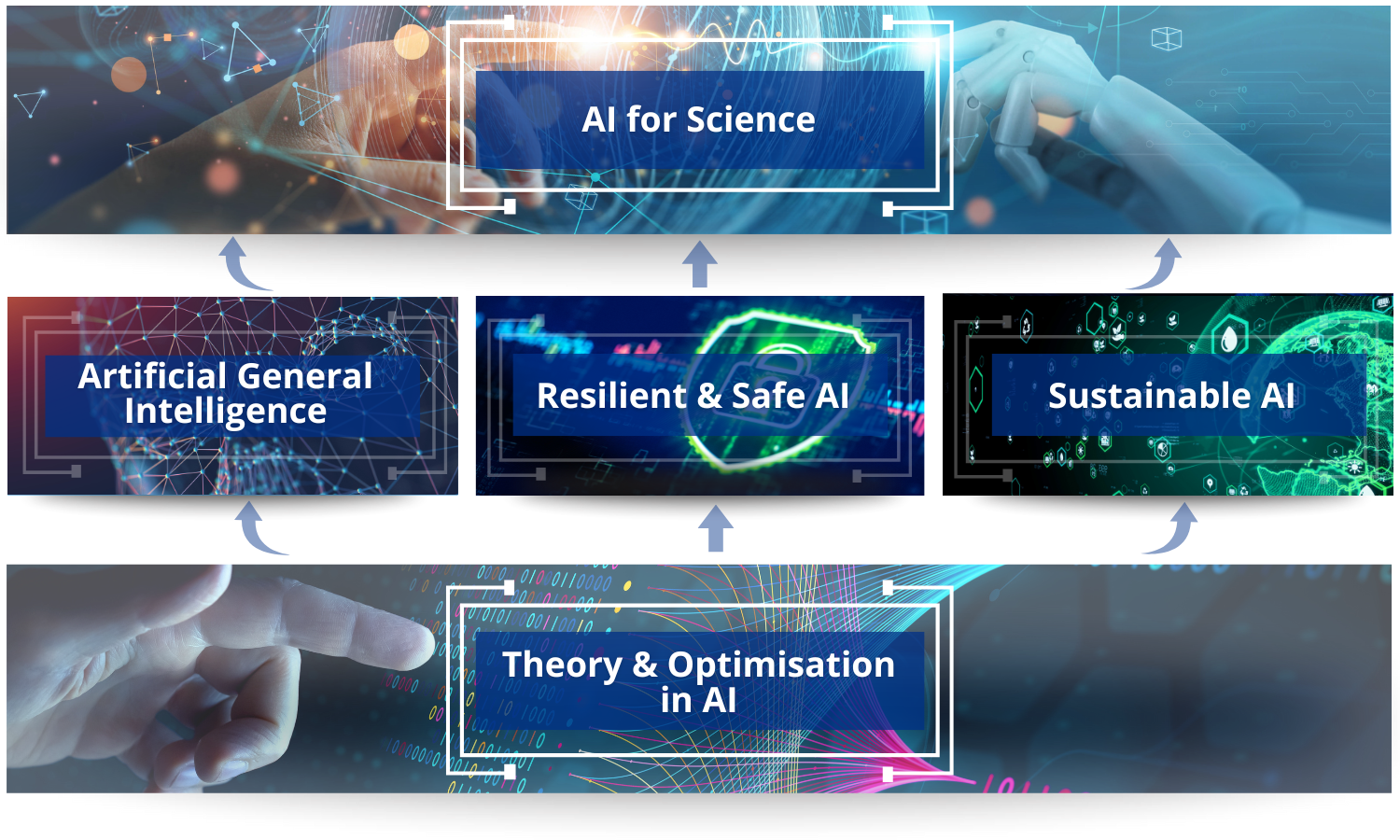
Publications
Upcoming Events
Friday, 02 February 2024
[CFAR Rising Star Lecture Series] Beyond Image Generation - Diffusion Models for 3D Pose and Mesh Recovery
Tuesday, 05 December 2023
[CFAR Rising Star Lecture Series] Improving and Evaluating Adversarial Robustness by Dr Pang Tianyu
In this talk, Dr Pang Tianyu will introduce his work on improving adversarial training from the perspectives of robustness-accuracy tradeoff and diffusion-based data augmentation. He will also demonstrate how to perform adversarial attacks against large vision-language models.
Thursday, 17 August 2023
[CFAR Outstanding PhD Student Seminar Series] Distribution-Shift Robustness via Diversity and Non-Learning by Lee Yoonho
Real-world conditions are non-stationary, rather than static. When deployed in the wild, machine learning (ML) systems encounter distribution shifts, i.e., conditions that differ from what was seen during training. Conventional machine learning systems often struggle to extrapolate to new data distributions, consequently leading to severe failures.In this talk, Lee Yoonho from Stanford University will present two methods that make ML models more robust to distribution shifts based on the principles of diversity (considering all explanations) and non-learning (not adapting more than necessary).
Friday, 04 August 2023
[CFAR Distinguished Professor Lecture Series] A Family of Pretrained AI Models for Target Discovery and Drug Design by Dr Song Le
How can we leverage large amounts of unsupervised data models to accelerate target discovery and drug design? In this talk, Dr. Song Le from BioMap and Mohamed bin Zayed University of AI (MBZUAI) will introduce the xTrimo family of large-scale and interrelated pre-trained models across a multiscale of biological processes, integrating a huge amount of data from protein sequences, structures, protein-protein interactions and single-cell transcriptomics data. These pretrained models could be used downstream to address many problems arising from target discovery and drug design. Dr. Song will then conclude the talk by illustrating examples, to show that xTrimo models may achieve State-Of-The-Art (SOTA) in drug target combination and antigen-antibody complex structure predictions.
Wednesday, 19 July 2023
[CFAR Outstanding PhD Student Seminar Series] Effective and Efficient Continual Learning by Wang Zifeng
Continual Learning (CL) aims to develop models that mimic the human ability to learn continually without forgetting the knowledge acquired earlier. While traditional machine learning methods focus on learning with a certain dataset (i.e., task), CL methods adopt a single model to learn a sequence of tasks continually. In this talk, Wang Zifeng from Northeastern University will share how his team develops effective and efficient CL methods under different challenging and resource-limited settings. First, he will present the novel prompting-based paradigm for parameter-efficient CL. It leverages the idea of sparsity to achieve cost-effective CL that aims to train a more succinct memory system that is both data and memory efficient. A method was then introduced to dynamically prompt (Learning to Prompt, CVPR 2022) a pre-trained model to learn tasks sequentially under different task transitions, where prompts are small learnable parameters maintained in a memory space. To improve Learning to Prompt (L2P), his team proposed ‘DualPrompt’ (ECCV 2022) that decouples prompts into complementary “General” and “Expert” prompts to learn task-invariant and task-specific instructions, respectively. For the second segment, Zifeng will present Sparse Continual Learning (SparCL, NeurIPS 2022) - a novel framework that leverages sparsity to enable cost-effective continual learning on edge devices. He will discuss how SparCL could achieve both training acceleration and accuracy preservation through the synergy of three aspects: weight sparsity, data efficiency, and gradient sparsity. Lastly, he will conclude the effectiveness and efficiency of his team’s methods over the state-of-the-art methods on multiple CL benchmarks.
Wednesday, 12 July 2023
[CFAR Rising Star Lecture Series] Neural Radiance Fields with Light Transport Occlusions by Prof Wan Renjie
Neural Radiance Fields (NeRF), a framework for Novel View Synthesis, has emerged as a crucial tool in a broad range of applications, including 3D rendering, robotic perception, and AIGC. It has gained popularity due to its capacity to meticulously render every detail of 3D scenes from 2D images, making it a sought-after representation for different medias in the forthcoming era. However, NeRF's training methodologies pose a challenge due to the difficulty in integrating external priors, which may lead to unwanted occlusions in the rendered outputs. In this talk, Prof Wan Renjie from the Hong Kong Baptist University will share the results from his exploration of NeRF alongside Light Transport Occlusions. Prof Wan will conclude the talk by showcasing an assortment of application instances related to 3D scene editing that were established his research findings.
Wednesday, 05 July 2023
[CFAR Rising Star Lecture Series] Reliable Visual Perception by Gu Jindong
Visual perception plays a crucial role across various domains, including autonomous driving, robotics and computer vision applications. In this talk, Gu Jindong from the University of Oxford will present his research on reliable visual perception, with specific focus on the robustness of vision models from multiple perspectives: data, model, task and learning paradigm. He will also emphasise the critical factors that impact vision robustness, including potential data perturbations (Natural Variations and Adversarial Attacks), different model architectures (CNN, ViT and CapsNet), the format of tasks (Classification, Segmentation, and Multi-tasks) and different learning paradigms (Standard Learning, Pre-training and Prompting).
Wednesday, 14 June 2023
[CFAR Outstanding PhD Student Seminar Series] Visual Knowledge and Reasoning with Large Language Models: Classification via Description and Visual Inference via Program Execution for Reasoning (ViperGPT) by Sachit Menon
While large language models (LLMs) have shown impressive capabilities in a wide range of natural language tasks, how could they help us solve computer vision tasks without being trained on visual data?In this talk, Sachit Menon from Columbia University will discuss two research works that leverage LLMs toward visual tasks: determine *what* information to use for a visual task (visual knowledge) and *how* to use that information (visual reasoning). The first work presents an alternative framework for classification with Vision-Language Models (VLMs), also known as classification by description. The VLMs will check for descriptive features rather than broad categories, i.e., to find a tiger, look for its stripes; its claws and more. He will also explain how these descriptive features could be obtained by a language model and achieve better performance with inherent interpretability. The second work will introduce ViperGPT, a framework that leverages code-generation models to compose vision-and-language models into subroutines to produce a result for any visual query. Sachit will conclude his talk by sharing how both approaches require no additional training and are able to obtain state-of-the-art results on various datasets, providing practical intermediate results, and enabling much more interpretability than previous models.
Wednesday, 31 May 2023
[CFAR Rising Star Lecture Series] Knowledge-Driven Multimodal Representation Learning by Assoc Prof Xie Weidi
In recent years, foundation models have shown tremendous success. In essence, these models trained on web data have shown to encode a large amount of human knowledge. For example, ChatGPT, GPT4 are able to freely chat with humans on most topics. In this talk, Associate Prof Xie Weidi from Shanghai Jiao Tong University will introduce some of his recent works on exploiting knowledge within the foundation models and expand the ability of existing computer vision systems towards open-vocabulary scenarios – for example, action recognition, object detection, segmentation, audio description for movies, etc. He will also discuss his recent research focus on AI4science, specifically on representation learning in the medical domain that requires large amount of human knowledge to be involved, particularly in medical image analysis, disease prediction, and clinical decision-making, etc.
Wednesday, 07 June 2023
[CFAR Distinguished Professor Lecture Series] Night Images: Challenges and Solutions from the Perspective of Visibility Enhancement and Object Detection by Prof Robby T. Tan
Nighttime conditions are largely associated with low light conditions, which constitute related but different degradation problems, such as low intensity values, high levels of noise (a low signal-to-noise ratio), low contrast, low colour saturation, blurry edges due to noise and low intensity, blur due to motion, etc. Noise is significantly present in low light images because the actual signals emitted by the scene are weak, causing the random noise generated by the camera to dominate the pixel intensity values. In some severe cases, noise levels are higher than the scene pixel intensity, making the recovery intractable. While the low light problem is dominant in nighttime conditions, there are other significant problems.
One of them is the imbalanced distribution of light, particularly when there are man-made lights present. In the areas nearby the light sources, the light can be strong, yet in some distant regions from the sources, the light is considerably weak. The imbalance of light distribution is usually manifested in visual effects like flare, glare and glow. In nighttime conditions, the presence of glow can be prominent, particularly when there are a considerable amount of atmospheric particles, in hazy or foggy scenes. The combination of glow and haze/fog can also degrade the visibility significantly since glow somehow occludes the scene behind.
In this talk, Prof Robby Tan from the National University of Singapore will discuss the challenges in nighttime particularly from the perspective of visibility enhancement and object detection.
Wednesday, 17 May 2023
[CFAR Distinguished Professor Lecture Series] Smart FinTech: AI and Data Science for Finance by Prof Cao Longbing
Smart FinTech has emerged as one of the most incredible application areas of new-generation artificial intelligence and data science (AIDS). Financial innovations, businesses and data are diversified and challenging, posing significant theoretical and practical settings and questions to AIDS research and applications. In this talk, Prof Cao Longbing from the University of Technology Sydney (UTS) will briefly review the areas of smart FinTech, the landscape of classic to modern AIDS research in finance and data-driven analytics and learning for open finance. He will then illustrate shallow to deep analytics and learning for cross-market applications including asset representation, financial crisis and portfolio investment, and banking services.
Friday, 12 May 2023
[CFAR Outstanding PhD Student Seminar Series] Understanding Modern Machine Learning Models Through the Lens of High-Dimensional Statistics by Denny Wu
Modern machine learning tasks are often high-dimensional, due to the large amount of data, features, and trainable parameters. Mathematical tools such as random matrix theory have been developed to precisely study simple learning models in the high-dimensional regime, and such precise analysis can reveal interesting phenomena that are also empirically observed in deep learning.
In this talk, Denny Wu from the University of Toronto will introduce two examples concluded by him and his research team. He will first talk about the selection of regularisation hyperparameters in the over-parameterised regime and how the team established a set of equations that rigorously describes the asymptotic generalisation error of the ridge regression estimator. These equations led to some surprising findings: (i) the optimal ridge penalty can be negative and (ii) regularisation can suppress “multiple descents” in the risk curve. He will then discuss practical implications such as the implicit bias of first- and second-order optimisers in neural network training. Next, Denny will go beyond linear models and characterise the benefit of gradient-based representation (feature) learning in neural networks. By studying the precise performance of kernel ridge regression on the trained features in a two-layer neural network, his team has proven that feature learning could result in a considerable advantage over the initial random features model, highlighting the role of learning rate scaling in the initial phase of gradient descent
Tuesday, 25 April 2023
[CFAR Distinguished Professor Lecture Series] See What You Hide and Hide What You See by Prof Wang Zheng
Deep learning (DL) models are now so powerful that they could understand multimedia content directly and accurately – that is seeing what you see. However, the world is not just about what we could see. We are unable to see what lies inside these information, unlike visual models, which have a strong ability to see all. In special situations where privacy is concerned , we do not want the model to see more than it should.Therefore, Prof Wang Zheng and his team started working on the topic of “See What You Hide and Hide What You See". In this talk, Prof Wang will share two pieces of his work – Hidden Follower Discovery (See What You Hide) and HOTCOLD blocks (Hide What You See).
Thursday, 20 April 2023
[CFAR Outstanding PhD Student Seminar Series] Reducing the CO2 Emission of Training Deep-learning Models: Towards Efficient Large-Scale Dataset Distillation and Pruning by Wang Kai
Ultra-large-scale datasets could help deep learning (DL) achieve many remarkable results in computer vision (CV) and natural language processing (NLP) areas. However, training on such datasets is costly and results in heavy CO2 emissions. Dataset distillation and pruning may reduce training costs by generating (selecting) small but informative datasets with little information loss while utilising fewer samples and achieving comparable results as the original dataset. In this talk, Wang Kai from the National University of Singapore (NUS) will introduce three recent works: (1) Designing efficient matching strategies to reduce the iterations of previous works, where the team proposed a novel matching strategy named Dataset Distillation by REpresentAtive Matching (DREAM) which only selects representative original images for matching. DREAM could also be plugged easily into popular dataset distillation frameworks and may reduce matching iterations by 10 times without performance drop. He will then explain his second work on (2) Using the generative model as information container, where they introduced a novel distillation scheme to Distill information of large train sets into generative Models (DiM), which uses a generative model instead of small and informative images to store information of the target dataset. This scheme minimises the differences in logits predicted by a models pool between real and generated images. At the deployment stage, the generative model synthesises various training samples from random noises on the fly. Lastly, Wang will discuss about iii) Learning to reduce the number of forward, where the team proposed InfoBatch, a novel framework aiming to achieve lossless training acceleration through unbiased dynamic data pruning. Specifically, InfoBatch randomly prunes a portion of less informative samples based on the loss distribution and rescales the gradients of the remaining samples.
Wednesday, 12 April 2023
[CFAR Outstanding PhD Student Seminar Series] The Ultimate Solution for L4 Autonomous Driving: Cooperative Perception by Xu Runsheng
Autonomous driving perception systems are faced with significant challenges, such as occlusion and sparse sensor observations at a distance. Cooperative perception presents a promising solution to these challenges as it utilises vehicle-to-everything (V2X) communication that enables autonomous vehicles to share visual information with each other.In this seminar, Xu Runsheng from the University of California, Los Angeles will explore the state-of-the-art technologies in cooperative perception and present his recent research on the topic, including three published papers: (1) V2X-ViT: Vehicle-to-Everything Cooperative Perception with Vision Transformer (ECCV2022), (2) CoBEVT: Cooperative Bird's Eye View Semantic Segmentation with Sparse Transformers (CoRL2022) and (3) V2V4Real: A Real-world Large-scale Dataset for Vehicle-to-Vehicle Cooperative Perception (CVPR2023 Highlight). He will also share insights into the current developments and future directions in autonomous driving perception.
Thursday, 23 March 2023
[CFAR Rising Star Lecture Series] Data-efficient Learning with Dataset Condensation by Dr Zhao Bo
In numerous fields, larger datasets are increasingly required to achieve state-of-the-art performance. Storing these datasets and training models on them has become significantly more expensive, especially when validating multiple model designs and hyper-parameters. To address this, Dr Zhao Bo and his team have proposed Dataset Condensation, a training set synthesis technique for data-efficient learning to condense a large dataset into a small set of informative synthetic samples for training deep neural networks from scratch. In this talk, Dr Zhao will present his team’s recent progress in dataset condensation research and demonstrate some promising results from the application of dataset condensation to continual learning, neural architecture search and privacy-preserving learning.
Wednesday, 15 March 2023
[CFAR Rising Star Lecture Series] Learning Manifold Dimensions with Conditional Variational Autoencoders by Ms Zheng Yijia
The variational autoencoder (VAE) and its conditional extension (CVAE) have been found to be capable of achieving state-of-the-art results across multiple domains, however, their precise behaviour is still not fully understood, particularly in the context of data (like images) that lies on or near a low-dimensional manifold. For example, while prior work has suggested that the globally optimal VAE solution could learn the correct manifold dimension, a necessary (but not sufficient) condition for producing samples from the true data distribution, this has never been rigorously proven. Moreover, it remains unclear how such considerations would change when various types of conditioning variables are introduced, or when the data support is extended to a union of manifolds. In this talk, Zheng Yijia will share her insights on the latent space behaviour in VAE/CVAE models and demonstrate practical scenarios where the conditioning variables would allow the model to adaptively learn manifolds of varying dimensions across samples. She will then conclude the talk by introducing some common design choices for these models.
Tuesday, 28 February 2023
[CFAR Distinguished Professor Lecture Series] Trustworthy Machine Learning (ML) for Healthcare by Prof Finale Doshi-Velez
In many healthcare settings, recommendations on new treatment policies based on data from the current practice only. Despite many potential benefits of using prior data to improve future treatments, it is particularly challenging to apply machine learning in healthcare due to the low data quality and high levels of missingness in health data. In this talk, Prof Finale Doshi-Velez will first describe some machine learning (ML) algorithms that her team has developed for healthcare settings, with applications to decision-making for HIV treatments and in the intensive care unit (ICU). Next, she will also share the work done to validate their algorithms. While statistical checks are necessary to improve methods for off-policy evaluation, they are not sufficient. Prof Doshi-Velez will then introduce the ways of how various kinds of transparency have been incorporated for human experts to apply their domain knowledge and identify potential concerns. She will conclude the talk by describing some of their user studies that demonstrate how ML recommendations could lead people astray and the measures to resolve these issues.
Tuesday, 21 February 2023
[CFAR Distinguished Professor Lecture Series] Unifying Continual Learning and OOD Detection by Prof Liu Bing
Continual learning (CL) learns a sequence of tasks incrementally. One of its main setting, class incremental learning (CIL) remains a challenging scenario. While it is well known that catastrophic forgetting (CF) is a major difficulty for CIL, inter-task class separation (ICS) is equally challenging. In this talk, Prof Liu Bing will first present a theoretical investigation on ways to solve the CIL problem and the key results achieved: (1) The necessary and sufficient conditions for good CIL are good within-task prediction and task-id prediction; and (2) the task-id prediction is correlated with out-of-distribution (OOD) detection. The theory unifies OOD detection and continual learning and proves that a good CIL method could also perform OOD detection well. Prof Liu will also discuss how some new CIL methods (designed based on the theory) could outperform existing CIL baselines by a large margin and also perform OOD detection well.
OOD (or novelty) detection and incremental learning of the identified novel items are two key functions to enable artificial intelligence (AI) agents to function and learn independently in the open world with unknowns. Prof Liu will conclude the talk by exploring ways to enable learning on the fly in the open world to achieve open-world continual learning.
Wednesday, 15 February 2023
[CFAR Outstanding PhD Student Seminar Series] On the Expressive Power of Geometric Graph Neural Networks by Chaitanya K. Joshi
The expressive power of Graph Neural Networks (GNNs) has been studied extensively through the Weisfeiler-Leman (WL) graph isomorphism test. However, standard GNNs and the WL framework are inapplicable for geometric graphs embedded in Euclidean space, such as biomolecules, materials, and other physical systems.
In this seminar, Chaitanya K. Joshi will share a recent paper titled “On the Expressive Power of Geometric Graph Neural Networks” where he and the team proposed a geometric version of the WL test (GWL) for discriminating geometric graphs while respecting the underlying physical symmetries: permutations, rotation, reflection, and translation. They used GWL to characterise the expressive power of geometric GNNs that are invariant or equivariant to physical symmetries in terms of distinguishing geometric graphs. He will conclude the talk by explaining how GWL unpacks the ways key design choices influence geometric GNN expressivity: (1) Invariant layers have limited expressivity as they cannot distinguish one-hop identical geometric graphs; (2) Equivariant layers distinguish a larger class of graphs by propagating geometric information beyond local neighbourhoods; (3) Higher order tensors and scalarisation enable maximally powerful geometric GNNs; and (4) GWL’s discrimination-based perspective is equivalent to universal approximation. Synthetic experiments supplementing their results are available at https://github.com/chaitjo/geometric-gnn-dojo.
Read the full paper: https://arxiv.org/abs/2301.09308.
A*STAR celebrates International Women's Day
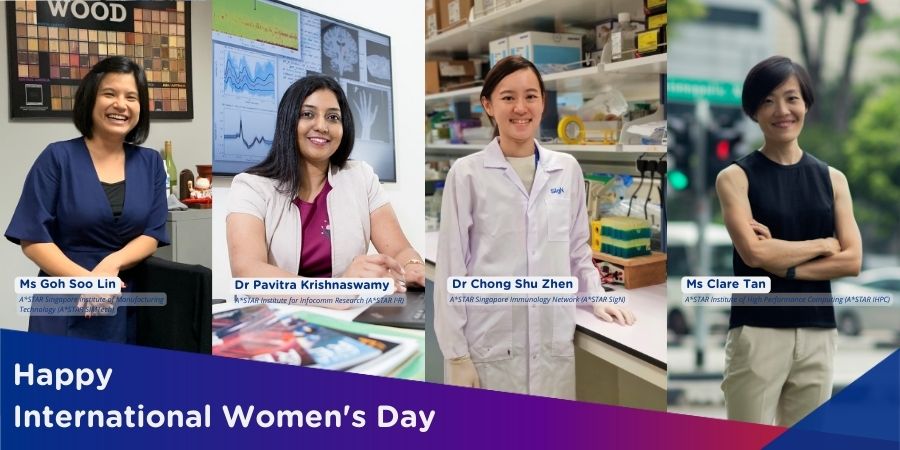
From groundbreaking discoveries to cutting-edge research, our researchers are empowering the next generation of female science, technology, engineering and mathematics (STEM) leaders.
Get inspired by our #WomeninSTEM