Research Pillars
CFAR is centred upon five key research pillars, namely:
- AI for Science: Harness AI to address and solve complex scientific challenges.
- Artificial General Intelligence: Investigate the development of general-purpose AI systems capable of solving any complex unseen problem.
- Resilient and Safe AI: Focus on developing robust and secure AI technologies to ensure safety and reliability.
- Sustainable AI: Commit to minimising the environmental impact of AI technologies.
- Theory and Optimisation in AI: Advance the theoretical foundations and improve the efficiency of AI algorithms.
These pillars reflect CFAR's commitment to driving innovation in AI across diverse sectors and pushing the boundaries of AI for impactful applications.
![]() |
A*STAR celebrates International Women's Day
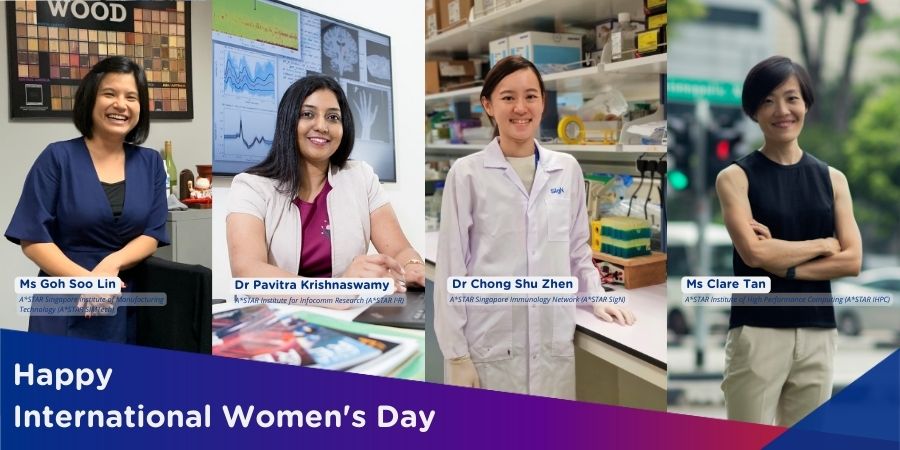
From groundbreaking discoveries to cutting-edge research, our researchers are empowering the next generation of female science, technology, engineering and mathematics (STEM) leaders.
Get inspired by our #WomeninSTEM