Research Pillars
- AI for Science
- Theory and Optimisation in AI
- Artificial General Intelligence
- Sustainable AI
- Resilient & Safe AI
Resilient & Safe AI
AI solutions are increasingly being deployed for real-world applications, to support human decision-making or even to make automated decisions. However, research has shown that AI models are trained highly specific to the datasets and not perform as well on novel unseen inputs. More precisely, AI models may fail when inputs are subject to shifts in the data distributions, for instance, due to adversarial attacks (such as adversarial examples and data poisoning) or changes in the deployment environment. These failures can sometimes cause catastrophic consequences, especially in safety-critical applications like self-driving vehicles and automated disease diagnosis. We therefore aim to develop AI models that are robust to such shifts (safe) and failing which, recover from these shifts (resilient).
In addition, Deepfake technology fabricates realistic images, audio, and videos, making it difficult for AI systems to distinguish between genuine and fake content, increasing the complexity of detection and defense. The misuse of Deepfakes could mislead or manipulate AI systems, compromising their decision-making safety and reliability. Moreover, the privacy and ethical issues posed by Deepfakes challenge the fairness and transparency of AI, highlighting the need for more robust safeguards and detection mechanisms to ensure the security of these systems.
Ensuring the safety of an AI system involves understanding how it works and the decision it makes. This allows us to then build in appropriate fail-safe mechanisms and fix issues when they arise. Having an interpretable and explainable AI system would help build trust in its predictions.
In summary, we focus on the following research areas:
- Adversarial Attacks & Defences: Ensure that AI models are resistant to various forms of intentional attacks.
- Robustness to Data Shifts: Aim to make AI models robust to shifts in data distribution between their deployment and training environment.
- Continual Learning: Enable models to adapt and recover from shifts in the data distribution.
- Deepfake Generation for Enhanced AI Defense: Develop advanced generation techniques to boost AI’s defense capabilities against manipulative content.
- Explainable and Interpretable AI: Understand and interpret how and why a prediction is made by an AI model to help build safeguards and engender trust.
Safety Issues in AI
Fig 2. Backdoor attacks in face recognition
Fig 3. Adversarial attacks in medical diagnosis
Fig 4. Nvidia DAVE-2 self-driving car platform
Fig 5. Biases in face recognition algorithms
Fig 6. A video generated by our in-house deepfake technology
Fig 7. Resilient & Safe AI and its applications
Glossary:
- Fig 1: PointBA: Towards Backdoor Attacks in 3D Point Cloud
- Fig 2.: Targeted backdoor attacks on deep learning systems using data poisoning
- Fig 3.: Adversarial attacks on medical machine learning
- Fig 4.: DeepXplore: Automated whitebox testing of deep learning systems
- Fig 5.: Najibi, A. (2020, October 24). Racial Discrimination in Face Recognition Technology. SITN.
A*STAR celebrates International Women's Day
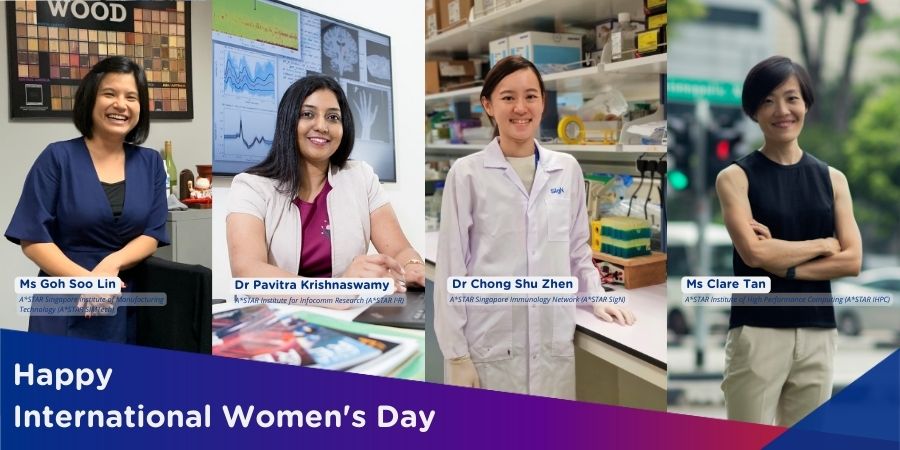
From groundbreaking discoveries to cutting-edge research, our researchers are empowering the next generation of female science, technology, engineering and mathematics (STEM) leaders.
Get inspired by our #WomeninSTEM