Research Pillars
- AI for Science
- Theory and Optimisation in AI
- Artificial General Intelligence
- Sustainable AI
- Resilient & Safe AI
Sustainable AI
While AI has attained remarkable achievements across multiple application domains, there remains significant concerns about the sustainability of AI. The quest for improved accuracy on large-scale problems is driving the use of increasingly deeper neural networks, which in turn increases energy consumption and climate-changing carbon emissions. For example, Strubell et al. estimated that training a particular state-of-the-art deep learning model resulted in 626,000 pounds of carbon dioxide emissions.
More broadly, decades of advances in scientific computing have clearly demonstrated the advantages of modelling and simulation across many domains. However, energy consumption will soon become a “hard feasibility constraint” for such computational modelling, and AI in HPC would be needed to reduce the energy cost of computation in the face of trends such as a declining Moore’s Law.
Thus, there is a rising and urgent need towards Sustainable AI for both AI and industry. To build and strengthen CFAR’s sustainable AI capabilities by significantly improving existing research, the team would focus on the following two paradigms, as previously described by van Wynsberghe:
- Sustainability of AI: To reduce carbon emissions and huge computing power consumption for AI models.
- AI for Sustainability and Sustainable Computing: To leverage AI for addressing environmental and climate problems and ameliorating the accelerating trend towards high-performance computing in modelling and simulation.
Specifically, for sustainability of AI, we will study how to reduce carbon emissions and huge computing power consumption via developing advanced AI technology in following areas:
For AI for sustainability, we will leverage AI for addressing pressing environmental problems, and ameliorating the growing carbon footprint from the increasing trend towards HPC in modelling and simulation. Digital modelling and simulation are of great importance to promoting sustainability and mitigating climate change.
AI can play an important role in both (i) enabling more accurate modelling, and (ii) reducing the cost of computing by reducing time-to-solution or reducing the need for high-resolution models, hence improving sustainability. In addition, this will also leverage on resource/data-efficient AI developments as per sustainability of AI to ensure that the application of AI to these challenges do not end up being as energy/resource consuming.
A*STAR celebrates International Women's Day
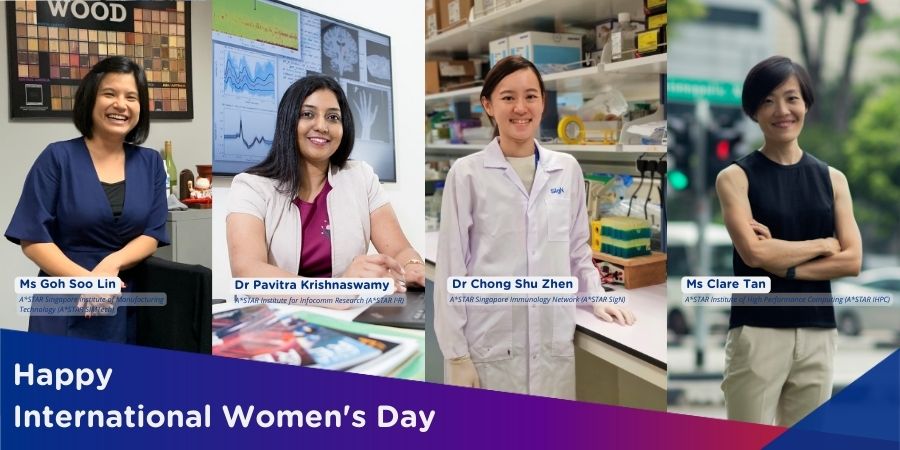
From groundbreaking discoveries to cutting-edge research, our researchers are empowering the next generation of female science, technology, engineering and mathematics (STEM) leaders.
Get inspired by our #WomeninSTEM