Few-Shot Adaptation of Pre-Trained Networks for Domain Shift
By: Wenyu Zhang, Li Shen, Wanyue Zhang and Chuan-Sheng Foo
While deep neural networks have demonstrated remarkable performance on a variety of tasks, their performance relies heavily on the assumption that training (source domain) and test (target domain) data distributions are the same. However, as real-world data collection can be difficult, time-consuming or expensive, it may not be feasible to adequately capture all potential variation in the training set, such that test samples may be subject to domain shift (also known as covariate shift).
In the recent work "Few-Shot Adaptation of Pre-Trained Networks for Domain Shift", the team propose a framework to adapt pre-trained source models at the batch normalization layers with only a few target samples. We evaluate on classification and semantic segmentation tasks, and the method improves source model performance with as few as one sample per class for classification tasks. Timely adaptation can help to prevent severe performance degradation when models are deployed.
A*STAR celebrates International Women's Day
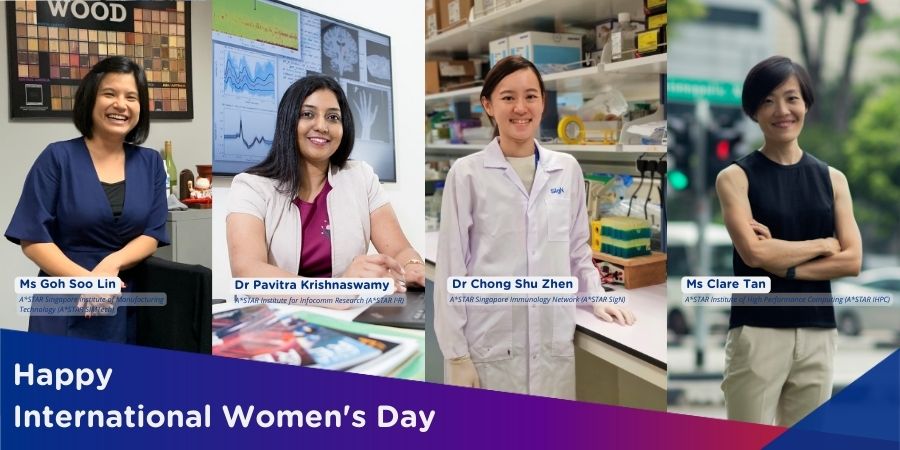
From groundbreaking discoveries to cutting-edge research, our researchers are empowering the next generation of female science, technology, engineering and mathematics (STEM) leaders.
Get inspired by our #WomeninSTEM