I²R Research Highlights
A bird’s eye view for improved diagnosis
An algorithm paves the way for accurate, rapid diagnosis of closed-angle glaucoma
Closed-angle glaucoma is when the optic nerve is damaged because the aqueous fluid in the eye does not drain properly. This is because the gap between the cornea and the iris, known as the anterior chamber angle, where fluid normally flows through the eye, has narrowed significantly or become blocked. The resulting build-up of pressure within the eye, which can happen rapidly, can cause irreversible blindness.
Current diagnosis of closed-angle glaucoma involves analyzing ‘anterior segment optical coherence tomography’ (AS-OCT) images, which are cross-sectional pictures of the eye’s anterior chambers. This takes considerable time and expertise.
"Our algorithm compares each individual image with a library of exemplar images, before producing a set of clinical parameters from each image that clinicians can use to validate diagnosis," explains Huazhu Fu at A*STAR’s Institute for Infocomm Research, who led the project alongside Mani Baskaran at Singapore Eye Research Institute, with scientists from China and the UK.
Fu’s team created a reference dataset of labelled images taken by different AS-OCT machines of several thousand individual eyes, both with and without clinically-diagnosed glaucoma. Their algorithm then uses the dataset to analyse newly-uploaded images.
"Although the eye structure shown in every AS-OCT image is different, local regions within each eye are similar. When our algorithm receives a new eye image, it searches the annotated dataset for 'best-fit' images that are the closest match in terms of eye structure and anterior chamber angle, before transferring the labels from these selected library images to the new image," Fu explains.
The algorithm then applies a smoothing technique to refine the structural labeling and ensure it is accurate for each unique eye shape. This allows very precise clinical measurements of the anterior chamber angle and other diagnostic features.
During tests, the algorithm was able to segment and measure one AS-OCT image in ten seconds and does not depend on specialist training, providing a potentially invaluable tool for clinicians.
The team are expanding their trials to larger datasets and aim to incorporate machine-learning techniques into the algorithm to further improve accuracy and performance.
The A*STAR-affiliated researchers contributing to this research are from the Healthcare & Medtech division of Institute for Infocomm Research.
Extracted from:
A*STAR Research - A bird’s eye view for improved diagnosis
A*STAR celebrates International Women's Day
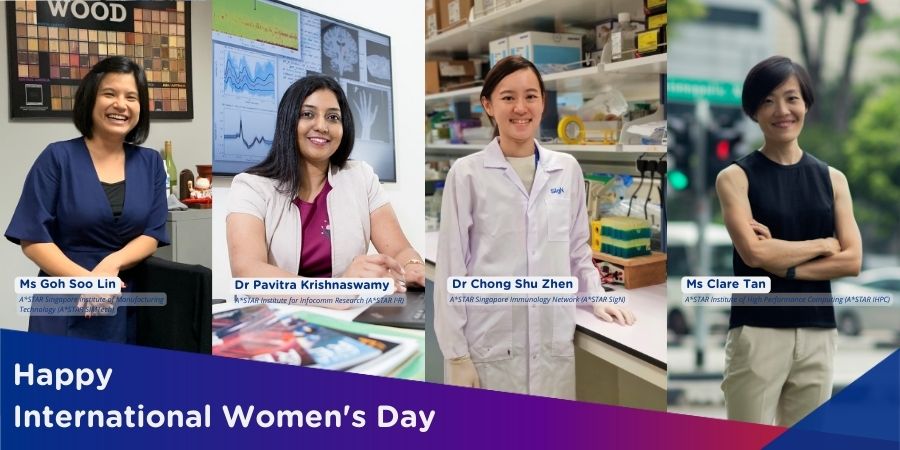
From groundbreaking discoveries to cutting-edge research, our researchers are empowering the next generation of female science, technology, engineering and mathematics (STEM) leaders.
Get inspired by our #WomeninSTEM