I²R Research Highlights
Adversarially learned anomaly detection
A novel Generative Adversarial Networks (GAN)-based anomaly detection method
Our Adversarially Learned Anomaly Detection (ALAD) is a novel Generative Adversarial Networks (GAN)-based anomaly detection that derives adversarially learned features for the anomaly detection task.
As compared with the only published GAN-based method, this method is able to achieve several hundred-fold faster anomaly detection by avoiding computationally expensive inference procedures.
On standard benchmark datasets – KDD99 and Arrhythmia, ALAD was compared against some of the best deep-learning based anomaly detection methods including AnoGAN, DSEBM and DAGMM. ALAD outperforms competing methods on the F1-score metric in the KDD99 dataset.
This enables faster anomaly detection in complex and high-dimensional data found in real world settings including cyber-security, manufacturing, fraud detection and medical imaging.
The A*STAR-affiliated researchers contributing to this research are from the Deep Learning 2.0 programme of Institute for Infocomm Research, École polytechnique and CentraleSupélPec.
Paper can be found in:
20th IEEE International Conference on Data Mining (ICDM), November 2018 - Adversarially learned anomaly detection, Houssam Zenati, Manon Romain, Chuan Sheng Foo, Bruno Lecouat, Vijay Ramaseshan Chandrasekhar
A*STAR celebrates International Women's Day
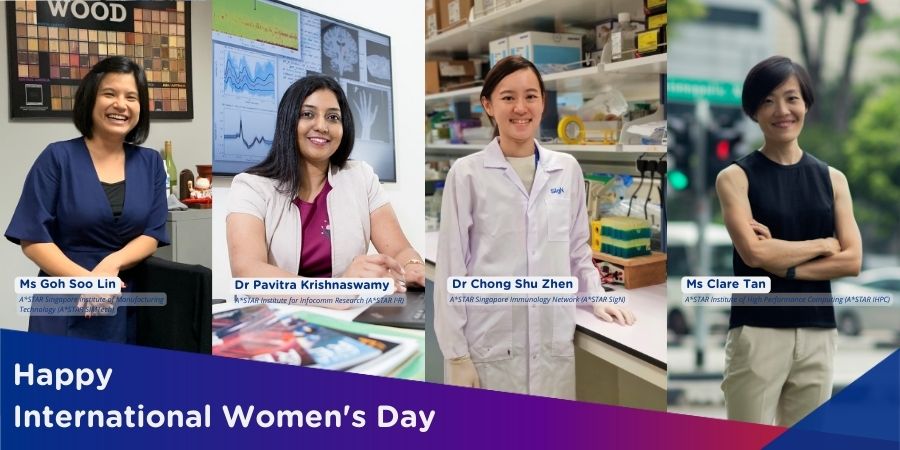
From groundbreaking discoveries to cutting-edge research, our researchers are empowering the next generation of female science, technology, engineering and mathematics (STEM) leaders.
Get inspired by our #WomeninSTEM