Densely Connected Attention Propagation for Reading Comprehension
03 Dec 2018
An algorithm that improves machine’s understanding of text for accurate real world applications
Being able to read a text does not necessary equates to the understanding of what it meant. This is similar in both human and machines.
Reading Comprehension is fundamental in the human world to fully enable us to correctly address the questions related to the text that we just read. In machines, the ability to better read and understand the text, the more accurate the response you will receive.
A*STAR I2R researchers proposed a new densely connected neural architecture for Reading Comprehension (DECAPROP) that uses Bidirectional Attention Connectors (BAC) as the central of our overall architecture.
It improves the information flow through the shortened signal path. DECAPROP also increases the number of interaction interfaces, i.e., matching in an asynchronous, cross-hierarchical fashion that lead to an improvement in performance. The modularity of this BAC also allows easier equipping to other models and domains thus enabling a wider usage of this model in reading comprehension applications.
When tested on four RC benchmark datasets that accurately reflect real world applications, our approach was able to outperform existing baselines by up to 2.6% to 14.2% in absolute F1 score.
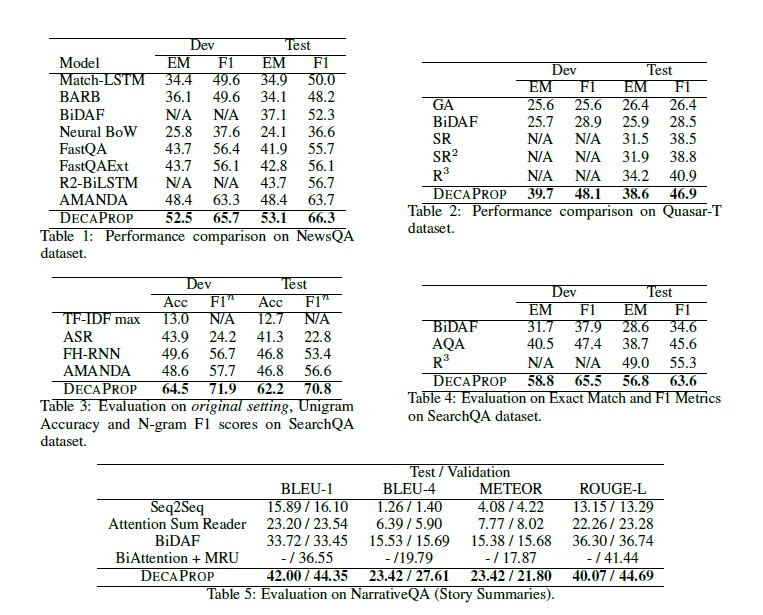
Derived from the research within Baidu I2R Research centre, this approach would potentially be adopted within the enhancement of Baidu search engine results.
The A*STAR-affiliated researchers contributing to this research are from the Aural & Language Intelligence department of Institute for Infocomm Research and Nanyang Technological University.
Paper can be found in:
Advances in Neural Information Processing Systems (NIPS) - Densely Connected Attention Propagation for Reading Comprehension, Yi Tay, Luu Anh Tuan, Siu Cheung Hui, Jian Su, December 2018