NEWS & ARTICLES
Overcoming drift with Metrological Traceability in Senor Networks
Author(s):
Dr CUI Shan, Scientist III, Acoustics & Vibration Laboratory
Senor Drift Challenges
In our online world, a growing number of devices like kitchen appliances, cars and machines now come with sensors that are connected to the Internet. These devices are part of a rapidly growing network of connected objects that collect and exchange data using embedded sensors, called the Internet of Things (IoT).
While IoT is mainly employed for consumer usage, industrial IoT (IIoT) is used more for purposes like manufacturing and energy management. The number of connected IoT devices in the world is expected to grow significantly, from 7.6 billion to 24.1 billion, between 2019 and 2030, according to Transforma Insights.
In IoT and IIoT deployments, a wide range of sensors are used—such as air quality sensors, temperature and vibration sensors. These sensors are useful for environmental condition monitoring and control, machine condition monitoring and more. IoT is a key technology that enables smart nation, smart manufacturing, smart energy management and more.
Even so, all sensors are affected by drift, where there are gradual changes in their responses, which in turn affects their accuracy and reliability. While the accuracy of readings from sensitive electronic sensors often deteriorates over time, the frequent replacement of sensors is often not practical as it may be costly to do so or they may be hard to access. As such, data generated from sensors that have drifted may lead to bad decisions and incorrect feedback controls, which could be very costly to rectify eventually. For example, CO2 sensors are used for demand-controlled ventilation in buildings to achieve energy saving in ventilation. If the controlling CO2 sensor drifts to a reading much higher than it is in reality, then energy may be expended by the ventilation system attempting to correct the situation indicated by the erroneous data leading to energy wastage instead of energy saving.
“As sensor networks become more pervasive, it is very difficult to ensure that all sensors are giving reliable and trustworthy data, especially after one to three years of deployment,” said Dr Cui Shan, Scientist at the National Metrology Centre (NMC), Singapore’s national measurement authority. “It’s vital to ensure the accuracy of measurements and confidence in the sensing data as they have a direct impact on test results, product quality or process efficiency. The errors from noise or drift in sensor readings would impact the reliability of the subsequent data analytics and artificial intelligence models,” said Dr Cui Shan.
Addressing sensor drift
The traditional approach to reducing inaccurate measurements caused by sensor drift is to undertake a regular and time-consuming preventive maintenance calibration programme.
The team at NMC has worked to enable data-driven IoT sensor data quality assurance by minimising laboratory-based calibration of the measurement tools for better productivity and to lower sensor maintenance cost. The goal is to ensure measurement traceability to the International System of Units (SI).
Combining data analytics with metrological understanding, NMC has developed a self-diagnosis and self-healing scheme for sensor networks that monitors and diagnoses sensor data reliability.
The technology provides self-awareness of the sensor’s health using metrological principles. The autonomous sensor drift identification and compensation is achieved through machine learning with measurement traceability back to physical measurement standards to ensure accurate and reliable sensor data. As a result, sensor drift is automatically corrected and unhealthy sensors are identified and ruled out from the control loop. This can replace most lab-based calibration, which is laborious, time-consuming, and interrupts the production or work process.
The self-monitoring and self-healing features are especially important in IoT networks that use large numbers of sensors as it would not be feasible to carry out periodic calibrations in the laboratory for all these sensors. The technology is suitable for sensor networks, IoT and IIoT deployments for smart nation projects, smart factories and smart buildings.
“Going forward, we plan to collaborate with industry partners to turn the technology into products and to look for more use cases,” said Dr Cui Shan.
“For organisations that use sensor feedback control systems, it is important to understand the risks and avoid a situation where sensor drifts and failures go undetected, as doing so can help save time, reduce costs and avoid operational challenges.”
A*STAR celebrates International Women's Day
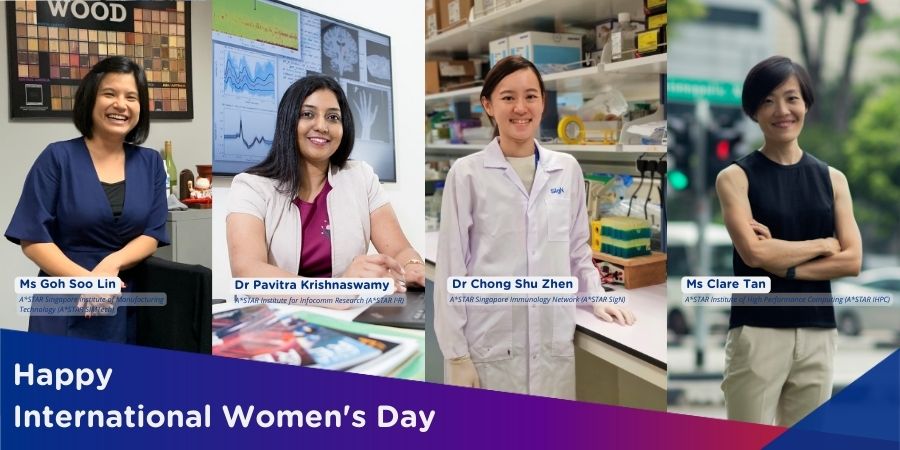
From groundbreaking discoveries to cutting-edge research, our researchers are empowering the next generation of female science, technology, engineering and mathematics (STEM) leaders.
Get inspired by our #WomeninSTEM