Grant Awards
Grant Awards
AI Singapore Grand Challenge in Robust AI
27 Jul 2023
Organised in collaboration with the Future Systems and Technology Directorate, MINDEF Singapore, and DSO National Laboratories, the Robust AI Grand Challenge aims to encourage the development of innovative approaches that address the vulnerabilities of Artificial Intelligence (AI) models in Computer Vision (CV) systems for Autonomous Vehicles (AV). It supports ground-breaking research ideas that adopt
AI techniques to address the following challenge: “How can we design robust CV systems for AVs that can recover at least 80% of their original accuracy after physical testing-time adversarial attacks?”
A project titled "Towards Building Unified Autonomous Vehicle Scene Representation for Physical AV Adversarial Attacks and Visual Robustness Enhancement (Stage 1a)” seeks to develop a unified framework to conduct physical
adversarial attacks and defenses with a novel unified autonomous vehicle scene representation. In real-world situations, the state-of-the-art visual perception models in autonomous vehicles (AV) fail when met with adversarially designed physical objects/environmental
conditions. The main reason is that they are trained with discretely-sampled samples and can hardly cover all possibilities in the real world.
An effective path towards robust visual perception models is to first develop physical attacks and corresponding defence methods, and then iterate. Although effective, existing physical attacks consider one or two physical factors but are unable to simulate
dynamic entities (i.e., moving cars or persons, street structures) and environment factors (i.e., weather variation and light variation) jointly. Meanwhile, most defence methods like denoising or adversarial training (AT) mainly rely on single-view
or single-modal information, hence neglecting the multi-view cameras and different modality sensors on the AV that contain rich complementary information. The above challenges in both attacks and defenses are caused by the lack of a continuous and
unified scene representation for the AV scenarios.
To address the limitations, we develop a unified representation for AV scenarios based on the neural implicit representation, which is a hot and relatively new research topic. By solving challenges and exploring the opportunities of the unified AV representation,
we can develop physical adversarial attacks considering different physical factors jointly, and also unlock novel defensive methods with multi-view cameras and multiple modalities naturally.
Congratulations to the team of researchers from A*STAR’s Centre for Frontier AI Research (CFAR), Nanyang Technological University (NTU), Singapore Management University (SMU), and National University of Singapore (NUS) for receiving the funding
for the AI Singapore Grand Challenge in Robust AI.
Principal Investigator
NTU: Prof Liu Yang
Co-Principal Investigators
CFAR: Dr Guo Qing
NTU: Asst Prof Zhang Tianwei, Assoc Prof Zhang Hanwang and
Asst Prof Lyu Chen
NUS: Prof Dong Jin Song
A*STAR celebrates International Women's Day
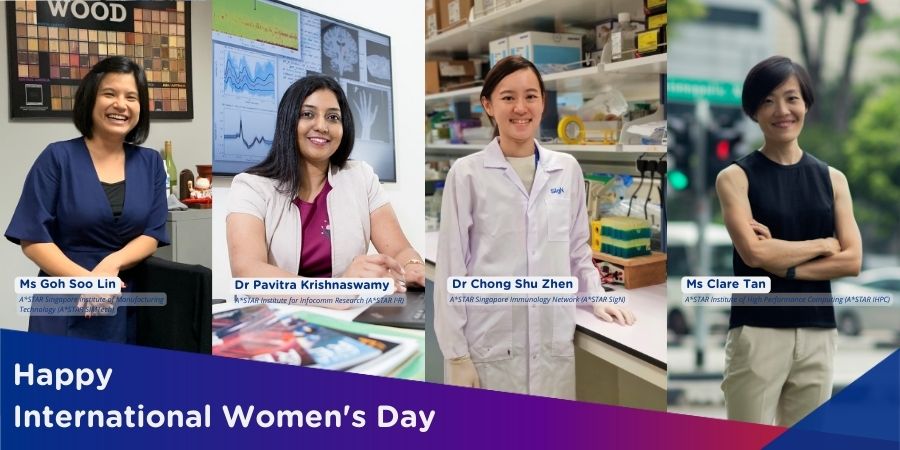
From groundbreaking discoveries to cutting-edge research, our researchers are empowering the next generation of female science, technology, engineering and mathematics (STEM) leaders.
Get inspired by our #WomeninSTEM