News
10 Papers Accepted at CVPR 2025
Held from 11th – 15th June 2025 at Nashville, Tennessee, the IEEE/CVF Computer Vision and Pattern Recognition Conference (CVPR) is a premier event that covers advances in computer vision, pattern recognition, large language models (LLMs), artificial intelligence (AI), machine learning and more. The annual conference will bring together students, researchers and industry professionals to explore and discuss the latest breakthroughs shaping the field.
Congratulations to the following scientists from A*STAR Centre for Frontier AI Research (A*STAR CFAR) on having their papers accepted:
- Prof Ivor Tsang, Director, A*STAR CFAR
- Prof Ong Yew Soon, Chief Artificial Intelligence (AI) Scientist and Advisor
- Dr Joey Zhou, Deputy Director, A*STAR CFAR and Principal Scientist
- Dr Foo Chuan Sheng, Principal Scientist
- Dr Lim Joo Hwee, Principal Scientist
- Dr Zhang Mengmi, Principal Scientist
- Dr Guo Qing, Senior Scientist
- Dr Li Chen, Senior Scientist
- Dr Qian Hangwei, Scientist
- Dr Zhang Jie, Scientist
List of accepted papers:
1. | Gazing at Rewards: Eye Movements as a Lens into Human and AI Decision-Making in Hybrid Visual Foraging Bo Wang, Dingwei Tan, Yen-Ling Kuo, Zhaowei Sun, Jeremy M. Wolfe, Tat-Jen Cham, Mengmi Zhang This paper is the first to introduce visual foraging problem in computer vision and the first to propose a model trained via reinforcement learning (RL) for this task. It demonstrates alignment with humans and has been publicised in MIT technology review. |
2. | SceneTAP: Scene-Coherent Typographic Adversarial Planner against Vision-Language Models in Real-World Environments Yue Cao, Yun Xing, Jie Zhang, Di Lin, Tianwei Zhang, Ivor Tsang, Yang Liu, Qing Guo We built the first LLM-agent-driven typographic physical attack against powerful large vision-language models including ChatGPT4o. |
3. | ChatGen: Automatic Text-to-Image Generation From FreeStyle Chatting Chengyou Jia, Changliang Xia, Zhuohang Dang, Weijia Wu, Hangwei Qian, Minnan Luo This paper aims to automate tedious steps during prompting, and to allow users to simply describe their needs with freestyle chatting. A benchmark ChatGenBench is designed for Automatic Text-to-Image Generation, and a multi-stage progressive strategy named ChatGen-Evo is proposed. |
4. | ProjAttacker: A Configurable Physical Adversarial Attack for Face Recognition via Projector Yuanwei Liu, Hui Wei, Chengyu Jia, Ruqi Xiao, Weijian Ruan, Xingxing Wei, Joey Tianyi Zhou, Zheng Wang Previous physical adversarial attacks have shown that carefully crafted perturbations can deceive face recognition systems, revealing critical security vulnerabilities. However, these attacks often struggle to impersonate multiple targets and frequently fail to bypass liveness detection. Thus, we propose a novel physical adversarial attack using a projector and explore the superposition of projected and natural light to create adversarial facial images. |
5. | Deterministic-to-Stochastic Diverse Latent Feature Mapping for Human Motion Synthesis Hua Yu, Weiming Liu, Gui Xu, Yaqing Hou, Yew-Soon Ong, Qiang Zhang This paper proposes a Deterministic-to-Stochastic Diverse Latent Feature Mapping approach for human motion synthesis. The human motion reconstruction stage learns the latent space distribution of human motions while the diverse motion generation phase build connections between Gaussian distribution to the latent space distribution of human motions, thus generating both diversity and accuracy in the generated human motions. |
6. | Learning with Noisy Triplet Correspondence for Composed Image Retrieval Shuxian Li, Changhao He, XitingLiu, Joey Tianyi Zhou, Xi Peng, Peng Hu We propose a Task-oriented Modification Enhancement framework (TME) to learn robustly from noisy triplets, which comprises three key modules - Robust Fusion Query (RFQ), Pseudo Text Enhancement (PTE), and Task-Oriented Prompt (TOP). |
7. | AVF-MAE++: Scaling Affective Video Facial Masked Autoencoders via Efficient Audio-Visual Self-Supervised Learning Xuecheng Wu, Heli Sun, Yifan Wang, Jiayu Nie, Jie Zhang, Yabing Wang, Junxiao Xue, Liang He We introduce AVF-MAE++, a series audio-visual MAE designed to explore the impact of scaling on AVFA with a focus on advanced correlation modelling. |
8. | Reconstructing Close Human Interaction with Appearance and Proxemics Reasoning Buzhen Huang, Chen Li, Chongyang Xu, Dongyue Lu, Jinnan Chen, Yangang Wang, Gim Hee Lee In this work, we find that human appearance can provide a straightforward cue to address these obstacles. Based on this observation, we propose a dual-branch optimisation framework to reconstruct accurate interactive motions with plausible body contacts constrained by human appearances, social proxemics, and physical laws. |
9. | CADCrafter: Generating Computer-Aided Design Models from Unconstrained Images Cheng Chen, Jiacheng Wei, Tianrun Chen, Chi Zhang, Xiaofeng Yang, Shangzhan Zhang, Bingchen Yang, Chuan-Sheng Foo, Guosheng Lin, Qixing Huang, Fayao Liu This work describes CADCrafter, an image to parametric CAD model generation framework that trains a latent diffusion network solely on synthetic textureless CAD data while testing on real-world images. It also introduces a real-world dataset RealCAD comprising multi-view images and corresponding CAD command sequence pairs. It enables easy and efficient reverse engineering of CAD models from real-world images, with applications in manufacturing, design and simulation. |
10. | Visual Prompting for One-shot Controllable Video Editing without Inversion Zhengbo Zhang, Yuxi Zhou, Duo Peng, Joo Hwee Lim, Zhigang Tu, De Wen Soh, Lin Geng Foo Our method improves One-shot Controllable Video Editing (OCVE) by eliminating DDIM inversion and introducing a novel visual prompting approach. We further enhance content and temporal consistency in edited videos through Content Consistency Sampling (CCS) and Temporal-content Consistency Sampling (TCS), achieving superior results validated by extensive experiments. |
Learn more about CVPR 2025.
A*STAR celebrates International Women's Day
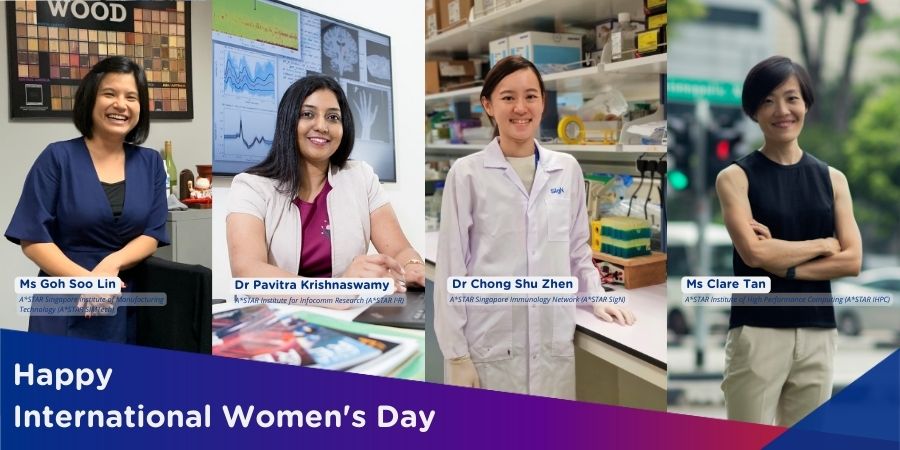
From groundbreaking discoveries to cutting-edge research, our researchers are empowering the next generation of female science, technology, engineering and mathematics (STEM) leaders.
Get inspired by our #WomeninSTEM