News
Features
18 Papers Accepted at ICLR 2025
04 Feb 2025
The International Conference on Learning Representations (ICLR) is the premier conference that gathers professionals who are dedicated to the advancement of the branch of artificial intelligence called representation learning; or generally referred to as 'Deep Learning'.
A total of 18 papers from A*STAR Centre for Frontier AI Research (A*STAR CFAR) have been accepted at ICLR 2025. Congratulations to the following scientists on this achievement:
- Prof Ivor Tsang, Director, A*STAR CFAR
- Prof Ong Yew Soon, Chief Artificial Intelligence (AI) Scientist and Advisor
- Dr Atsushi Nitanda, Principal Scientist
- Dr David Bossens, Senior Scientist
- Dr Du Jiawei, Senior Scientist
- Dr Foo Chuan Sheng, Principal Scientist
- Dr Guo Qing, Senior Scientist
- Dr He Yang, Scientist
- Dr Joey Zhou, Deputy Director, A*STAR CFAR and Principal Scientist
- Dr Li Jing, Scientist
- Dr Liu Yanzhu, Senior Scientist
- Dr Lyu Yueming, Scientist
- Dr Yu Xingrui, Scientist
- Dr Zhang Jie, Scientist
- Dr Zhang Xin, Scientist
Check out the poster sessions at ICLR from 24-28 Apr 2025, at Singapore EXPO.
List of accepted papers:
List of accepted papers:
1. | Direct Distributional Optimisation for Provable Alignment of Diffusion Models Ryotaro Kawata, Kazusato Oko, Atsushi Nitanda, Taiji Suzuki We introduce a novel alignment method for diffusion models from distribution optimisation perspectives while providing rigorous convergence guarantees. This framework is broadly applicable to general distribution optimisation problems, including alignment tasks in Reinforcement Learning with Human Feedback (RLHF), Direct Preference Optimisation (DPO), and Kahneman-Tversky Optimisation (KTO). |
2. | Heyang Zhao, Xingrui Yu, David Mark Bossens, Ivor Tsang, Quanquan Gu We propose a novel imitation learning algorithm namely Imitation Learning with Double Exploration (ILDE), which implements exploration in two aspects: (1) optimistic policy optimisation via an exploration bonus that rewards state-action pairs with high uncertainty to potentially improve the convergence to the expert policy, and (2) curiosity-driven exploration of the states that deviate from the demonstration trajectories to potentially yield beyond-expert performance. |
3. | Confidence Elicitation: A New Attack Vector for Large Language Models Brian Formento, Chuan-Sheng Foo, See-Kiong Ng We propose a new attack on LLMs based on asking it for its confidence in its predictions. It reflects a new attack vector and the risks of having calibrated probabilities in LLMs. |
4. | Broaden your SCOPE! Efficient Conversation Planning for LLMs with Semantic Space Zhiliang Chen, Xinyuan Niu, Chuan-Sheng Foo, Bryan Kian Hsiang Low Conversation planning typically uses many LLM queries for look-ahead simulation to select responses that maximize long-term rewards. By learning transition and reward models in text semantic space, we conversation plan without needing LLM queries. |
5. | Evidential Learning-based Certainty Estimation for Robust Dense Feature Matching Lile Cai, Chuan-Sheng Foo, Xun Xu, Zaiwang Gu, Jun Cheng, Xulei Yang Incorporates evidential deep learning to improve the robustness of dense feature matchers. |
6. | Robust-PIFu: Robust Pixel-aligned Implicit Function for 3D Human Digitalisation from a Single Image Kennard Chan, Fayao Liu, Guosheng Lin, Chuan-Sheng Foo, Weisi Lin Using Transfer Learning and Latent Diffusion Models to elevate the robustness of pixel-aligned implicit models in terms of dealing with occlusions and also to achieve SOTA performance. |
7. | On the Adversarial Risk of Test Time Adaptation: An Investigation into Realistic Test-Time Data Poisoning Yongyi Su, Yushu Li, Nanqing Liu, Kui Jia, Xulei Yang, Chuan-Sheng Foo, Xun Xu Re-investigates assumptions made in previous work on data poisoning during test time augmentation, proposes a new gray-box setting and provide a careful investigation in this, more realistic setting. |
8. | Easing Training Process of Rectified Flow Models Via Lengthening Inter-Path Distance Shifeng Xu, Yanzhu Liu, Adams Kong Recent research pinpoints that different diffusion methods and architectures trained on the same dataset produce similar results for the same input noise. By visualising the noise-sample pairs, we observe that the preferable paths are much well organized with significant fewer crossings comparing with the random paths. Inspired by this observation, we propose the Distance-Aware Noise-Sample Matching (DANSM) method to lengthen the inter-path distance for speeding up the model training. |
9. | Breaking Class Barriers: Efficient Dataset Distillation via Inter-Class Feature Compensator Xin Zhang, Jiawei Du, Ping Liu, Joey Tianyi Zhou This paper introduces Inter-class Feature Compensator (INFER), an innovative dataset distillation approach. INFER leverages a Universal Feature Compensator (UFC) to break the traditional class-specific data-label paradigm, enabling cross-class feature integration. It significantly improves distillation efficiency and generalisability by optimising synthetic data with label interpolation and reducing the use of soft labels to nearly zero. |
10. | Training-Free Dataset Pruning for Instance Segmentation Yalun Dai, Lingao Xiao, Ivor Tsang, Yang He Existing dataset pruning techniques primarily focus on classification tasks, limiting their applicability to more complex and practical tasks like instance segmentation. To address this, we propose a novel Training-Free Dataset Pruning (TFDP) method for instance segmentation. |
11. | VideoShield: Regulating Diffusion-based Video Generation Models via Watermarking Runyi Hu, Jie Zhang, Yiming Li, Jiwei Li, Qing Guo, Han Qiu, Tianwei Zhang We propose VideoShield, a watermarking framework for diffusion-based video generation models that embeds watermarks directly during generation, enabling tamper localisation both spatially and temporally without degrading video quality, and extending applicability to image generation. |
12. | Fast Direct: Query-Efficient Online Black-box Guidance for Diffusion-model Target Generation Kim Yong Tan, Yueming Lyu, Ivor Tsang, Yew-Soon Ong Guided diffusion models customize pre-trained diffusion models for downstream tasks but often rely on offline datasets or differentiable objectives, which limits real-world applications. We propose Fast Direct, a query-efficient, online algorithm supporting black-box objectives for tasks like human preference-based image generation or molecular design. |
13. | Sharpness-Aware Black-Box Optimisation Feiyang Ye, Yueming Lyu, Xuehao Wang, Masashi Sugiyama, Yu Zhang, Ivor Tsang Black-box optimisation often leads to suboptimal generalisation by directly minimising training loss. We propose SABO, a sharpness-aware algorithm reparameterising objectives with Gaussian distributions and iteratively optimizsng local maxima, improving generalisation. SABO’s convergence rate and generalisation bounds are theoretically proven. |
14. | Second-Order Fine-Tuning without Pain for LLMs: A Hessian Informed Zeroth-Order Optimiser Yanjun Zhao, Sizhe Dang, Haishan Ye, Guang Dai, Yi Qian,Ivor Tsang Fine-tuning LLMs with first-order optimisers is memory-intensive, while zeroth-order methods like MeZO face instability with heterogeneous curvatures. We propose HiZOO, a diagonal Hessian-informed optimiser, improving convergence and stability for fine-tuning LLMs with theoretical proof and visualized optimisation trajectories. |
15 | ProAdvPrompter: A Two-Stage Journey to Effective Adversarial Prompting for LLMs Hao Di, Tong He, Haishan Ye, Yinghui Huang, Xiangyu Chang, Guang Dai, Ivor Tsang With LLMs widely deployed, addressing vulnerabilities to jailbreaking attacks is crucial. We propose a novel two-stage adversarial prompter method, combining Exploration and Exploitation stages, to enhance performance while mitigating perplexity-based defenses effectively. |
16 | Trajectory-LLM: A Language-based Data Generator for Trajectory Prediction in Autonomous Driving Kairui Yang, Zihao Guo, Gengjie Lin, Haotian Dong, Zhao Huang, Yipeng Wu, Die Zuo, Jibin Peng, Ziyuan Zhong, Xin WANG, Qing Guo, Xiaosong Jia, Junchi Yan, Di Lin We introduce Trajectory-LLM (Traj-LLM), a new approach that takes brief descriptions of vehicular interactions as input and generates corresponding trajectories. |
17 | Generative Adversarial Ranking Nets (ICLR Journal Track) Yinghua Yao, Yuangang Pan, Jing Li, Ivor W. Tsang, Xin Yao The work proposes a novel generative adversarial learning paradigm that can learn the distribution of a user-specified subset of training data with theoretical guarantees. |
18 | Mentored Learning: Improving Generalisation and Convergence of Student Learner (ICLR Journal Track) Xiaofeng Cao, Yaming Guo, Heng Tao Shen, Ivor W. Tsang, James T. Kwok Student learners update hypotheses iteratively, which can lead to mistakes and costly convergence due to weak initialisation or inaccurate history. We propose “Mentored Learning,” where the learner's disagreement with the teacher is monitored. This method simplifies convergence analysis and improves generalisation bounds on error and label complexity. |
Find out more about ICLR 2025.
A*STAR celebrates International Women's Day
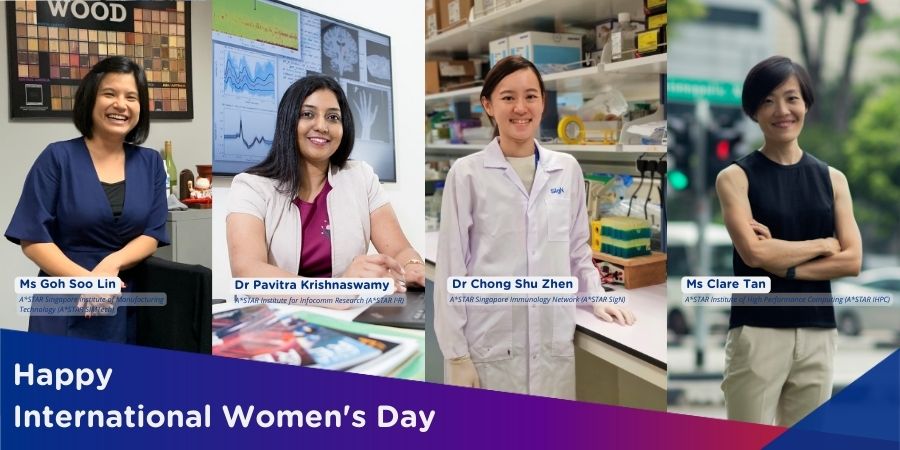
From groundbreaking discoveries to cutting-edge research, our researchers are empowering the next generation of female science, technology, engineering and mathematics (STEM) leaders.
Get inspired by our #WomeninSTEM