News
Features
5 Papers Accepted at IJCAI 2025
26 May 2025
The 34th International Joint Conference on Artificial Intelligence (IJCAI) is scheduled to take place in Montreal, Canada, from 16 – 22 August.
Since its inception in 1969, IJCAI has served as a platform for an exchange of groundbreaking advancements and achievements within the global AI community.
Congratulations to the following scientists from A*STAR Centre for Frontier AI Research (A*STAR CFAR) whose papers have been accepted at IJCAI 2025:
- Prof Ivor Tsang, Director, Distinguished Principal Scientist
- Prof Ong Yew Soon, Chief Artificial Intelligence (AI) Scientist and Advisor
- Dr Pan Yuangang, Senior Scientist
- Dr Yin Haiyan, Senior Scientist
- Dr Qian Hangwei, Scientist
- Dr Shi Yaxin, Scientist
- Dr Yao Yinghua, Scientist
- Mr Neoh Tzeh Yuan, Research Engineer
List of accepted papers:
1. | Not in My Backyard! Temporal Voting Over Public Chore Edith Elkind, Tzeh Yuan Neoh, Nicholas Teh We study a temporal voting model where voters have dynamic preferences over a set of public chores; projects that benefit society, but impose individual costs on those affected by their implementation. |
2. | Grounding Open-Domain Knowledge from LLMs to Real-World Reinforcement Learning Tasks: A Survey Haiyan Yin, Hangwei Qian, Yaxin Shi, Ivor Tsang, Yew-Soon Ong This paper introduces a comprehensive taxonomy for grounding large language models (LLMs) in reinforcement learning (RL) systems, enabling agents to perform reasoning, planning, and decision-making in dynamic real-world settings. By critically examining both training-free and fine-tuning paradigms across modalities, it offers strategic insights and a unified framework to advance the design of adaptive, knowledge-enhanced RL agents. |
3. | Instructing Text-to-Image Diffusion Models via Classifier-Guided Semantic Optimisation Yuanyuan Chang, Tao Qin, Yinghua Yao, Mengmeng Wang, Ivor Tsang, Guang Dai We propose a novel approach to text-to-image diffusion model editing that eliminates the need for manual text prompts. By optimising semantic embeddings guided by attribute classifiers, our method enables precise, disentangled edits without training or fine-tuning the diffusion model, enhancing control over image generation by aligning embeddings with desired attribute semantics. |
4. | Generative Co-Design of Antibody Sequences and Structures via Black-Box Guidance in a Shared Latent Space Yinghua Yao, Yuangang Pan, Xixian Chen We introduce LEAD, a latent space co-design framework that jointly optimises antibody sequence and structure to improve developability properties efficiently. By operating in a shared latent space with black-box guidance, LEAD outperforms existing methods while halving the number of required queries. |
5. | Evolvable Conditional Diffusion Wei Zhao, Ooi Chin Chun, Wong Jian Cheng, Gupta Abhishek, Chiu Pao Hsiung, Toh Sheares, and Ong Yew Soon This paper presents an evolvable conditional diffusion method such that black-box, non-differentiable multi-physics models – common in domains like computational fluid dynamics and electromagnetics, can be effectively used for guiding the generative process. |
Learn more about IJCAI 2025.
A*STAR celebrates International Women's Day
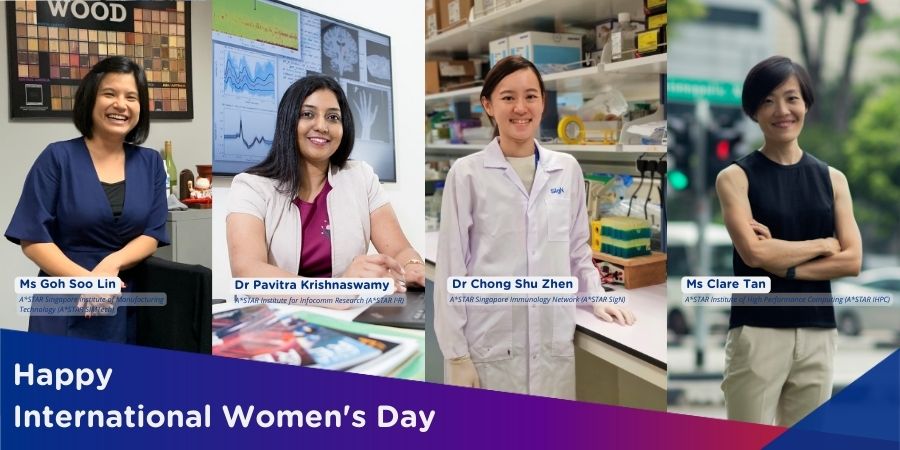
From groundbreaking discoveries to cutting-edge research, our researchers are empowering the next generation of female science, technology, engineering and mathematics (STEM) leaders.
Get inspired by our #WomeninSTEM