News
IEEE SmartCity 2022 Best Paper Award
-min.jpg?sfvrsn=e395e590_1)
The major challenge in this work was to effectively extract subtle and implicit features from WiFi signals, which often have a low signal-to-noise ratio. To address this challenge, Dr Li Bing and his team from A*STAR’s Centre for Frontier AI Research (CFAR) proposed the symmetry Transformer networks, a variant of Transformer networks that would facilitate better representation learning of nuanced features. Additionally, the team also developed a novel two-stage training method to overcome the limited availability of positive examples and low inter-environment transferability that could be caused by the Transformer networks. This involved using unsupervised representation learning which could help to reveal a transferability property, reducing the need for positive examples in training the resulting model.
Through empirical evaluation, the team has demonstrated the cost-effectiveness of their approach, while achieving superior performance compared to state-of-the-art models. Compared to the best previous methods, their model has achieved an average 14.69 absolute percentage point improvement in AUC-ROC (Area Under Curve - Receiver Operator Characteristic) score using only 1% annotated fall records.
The team won the IEEE Best Paper title "Cost-effective Elderly Fall Detection with Symmetry Transformer Networks" at the 20th IEEE International Conference on Smart City as part of 2022 IEEE Hyper-Intelligence Congress (HPCC/Smartcity/DSS/DependSys/ICESS/DIKW-2022) that was held in Chengdu, China from 18-21 December 2022.
The researchers contributing to this research include:
- CFAR: Li Bing, Joey Zhou Tianyi, Chen Zhenghua
- A*STAR's Institute for Infocomm Research (I2R): Cui Wei, Wu Min
- Sichuan University: Chen Yanru
- Shandong University of Science and Technology: Li Yuli
Li Bing's bio
![]() | Li Bing is a Research Scientist at A*STAR’s Centre for Frontier AI Research (CFAR), Singapore. Prior to joining CFAR, he was a Research Associate with the University of New South Wales, Australia. He received his Ph.D. degree from Northeastern University, China, in 2018. His research interests include intelligent information extraction, natural language processing, and machine learning. He was the awardee of the 2019 ACM China SIGMOD Chapter Doctoral Dissertation Award and served as PC member in various first-tier conferences, such as ICML, NeurIPS, AAAI, and ICLR. |
A*STAR celebrates International Women's Day
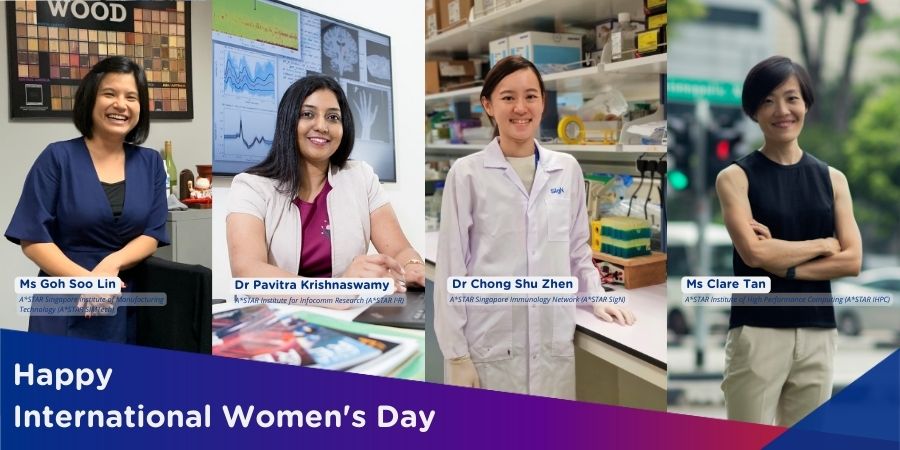
From groundbreaking discoveries to cutting-edge research, our researchers are empowering the next generation of female science, technology, engineering and mathematics (STEM) leaders.
Get inspired by our #WomeninSTEM