News
Runner-up Award at CVPR 2024 Workshop Challenge
Congratulations to Dr Guo Qing and his team members, Nhat Chung (SINGA Awardee), Sensen Gao (SIPGA Awardee), and Dr Ying Yang (Post-doc Scientist) for emerging as the Second-Place Winner of the Computer Vision and Pattern Recognition (CVPR) 2024 Workshop Challenge: Black-box Adversarial Attacks on Vision Foundation Models.
Organised by the 4th Workshop of Adversarial Machine Learning on Computer Vision: Robustness of Foundation Models, the challenge aims to encourage the development of innovative approaches that investigate the impact of vulnerabilities on safety-critical Artificial Intelligence (AI) systems, particularly the Computer Vision (CV) systems for Autonomous Vehicles (AV). The challenge explores research ideas that leverage AI techniques to investigate different ways to exploit vulnerabilities in CV systems for AVs that utilise Vision-Language Foundation Models (VLFMs) for reasoning and control.
The team’s project titled "Towards Transferable Attacks Against Vision-LLMs in Autonomous Driving with Typography” aims to develop a comprehensive attack framework to evaluate the vulnerabilities of VLFMs that were previously considered useful for AVs.
VLFMs are increasingly incorporated into AV systems for their advanced capabilities in visual-language reasoning, supporting perception with prediction, planning, and control mechanisms. However, findings from the project revealed their susceptibilities to adversarial attacks that could compromise their reliability and safety. By employing typographic attacks against AV systems relying on the decision-making capabilities of VLFMs, the project explores the risks in AV systems, transferability of practical threats, their influences on decision-making autonomy, and practical ways in which these attacks could be physically presented.
To achieve these objectives, the team first developed a framework that automatically generates false answers that could mislead the reasoning of VLFMs. Next, they studied a linguistic augmentation scheme to facilitate attacks at image-level and region-level reasoning, extending it to target multiple reasoning tasks simultaneously. Based on the new discoveries, the team discussed ways these attacks could occur during physical traffic scenarios in a follow-up paper. Through empirical study, the team evaluated the effectiveness, transferability, and realisability of typographic attacks in traffic scenes.
These findings underscore the significant impact of typographic attacks on existing VLFMs, raising community awareness on vulnerabilities when incorporating these models into AV systems.
Learn more about the CVPR 2024 Workshop Challenge.
A*STAR celebrates International Women's Day
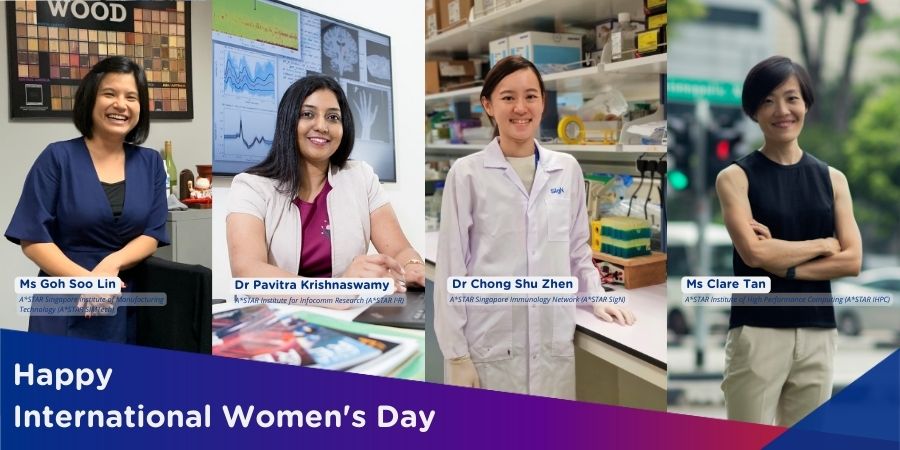
From groundbreaking discoveries to cutting-edge research, our researchers are empowering the next generation of female science, technology, engineering and mathematics (STEM) leaders.
Get inspired by our #WomeninSTEM