News
Recipient for AI Singapore Research Grant Call 2021
Self-Aware Continuous Learning Models
Learning continually, to accumulate knowledge, is one of the prominent features of human intelligence. Unlike this, deep learning-based AI models are mostly static under a strong assumption that all the distributions are known apriori and are pre-defined in the training data.
The project by Dr Li Xiaoli, Investigator at A*STAR’s Centre for Frontier AI Research (CFAR), aims to bridge the gap by developing Self-Aware Continuous Learning Models that are continuously:
- aware of the historical distributions,
- detect unknown novel distributions,
- estimate the complexity of the unknown distributions,
- choose relevant data to represent the unknown distributions, and
- adapt to represent new distribution without (catastrophically) forgetting any historical distribution.
It also improves AI models’ generalisation capability through representational adaptations with new data. The project will build AI models that learn throughout life, to enable critical applications that warrant dynamic and up-to-date decision making, in diverse application domains.
Memory-Efficient Online Continuous Object Recognition on Video Streams
The world is not stationary. To thrive in evolving environments, humans are capable of continual acquisition and transfer of new knowledge, from a continuous stream of highly correlated visual stimuli over multiple tasks, while retaining previously learned experiences.
In contrast to human learning, most AI systems focus on recognizing a pre-defined number of object classes. During training, these systems are often presented with a stationary pool of carefully shuffled, balanced, and homogenised images. In most cases, these systems struggle to recognise new objects when presented with a video stream of new object classes incrementally available over time. Meanwhile, they also fail to retain good performance on earlier learned classes without repeated passes over the data, known as catastrophic forgetting.
Dr Zhang Mengmi and Dr Sun Ying aim is to design a continual learning framework that recognises incrementally available object classes on video streams without repeated visits of the old data in a memory-efficient manner.
With multi-disciplinary approaches at the intersection of neuroscience, cognitive science, and AI, we hope to gain a better understanding of the following scientific questions and provide insights about replay mechanisms in biological and artificial intelligence:
- how AIs can make use of compositional structure and representations in the memory to learn to recognize new objects faster;
- what levels of abstract compositional representations from the old object classes are good for replay, how many times for replay and in what sequence to replay;
- how far our current AI continual learning systems are from human continual learning;
- how future generations of continual learning algorithms can be designed from inspirations of human continual learning behaviors; and
- how the strategies in AI systems assist human continual learning.
About AI Singapore Research Grant Call 2021
AI Singapore invites proposals in advanced fundamental AI research for the following themes:
- Theme One: Continuous Learning AI
- Theme Two: Resource Efficient AI
- Open AI Fundamental Research Theme
For more info, please refer to AI Singapore.
A*STAR celebrates International Women's Day
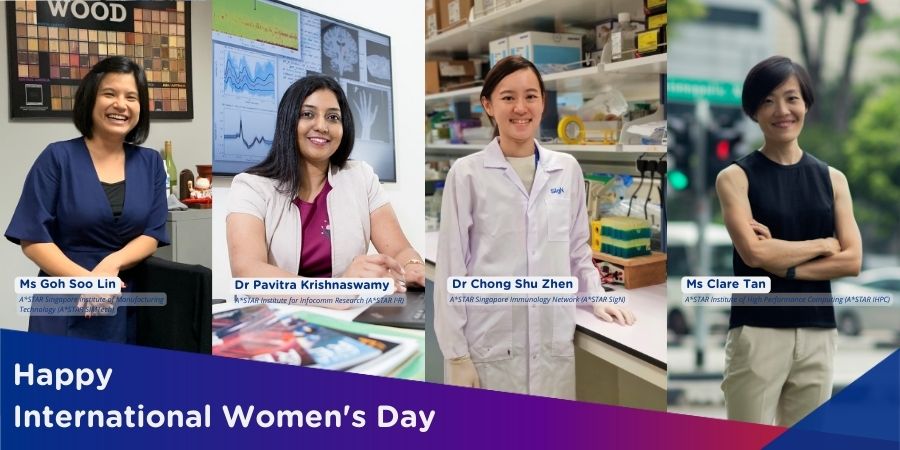
From groundbreaking discoveries to cutting-edge research, our researchers are empowering the next generation of female science, technology, engineering and mathematics (STEM) leaders.
Get inspired by our #WomeninSTEM