The A*STAR Career Development Fund (CDF) aims to support promising early-career researchers in developing their careers in A*STAR by providing project management experience and seed funding.
Congratulations to the following recipients for the A*STAR Career Development Fund (CDF) 2023:
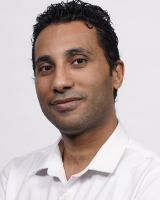
Mohamed Ragab Research Scientist | Label-Efficient and Resilient Federated Learning Approach for Time Series Applications
Dr Ragab’s work stands at the forefront of federated learning, offering innovative solutions to enhance data privacy and collaborative analysis in time series applications across diverse sectors such as manufacturing, healthcare,
and the Internet of Things. His project aims to streamline the sharing and utilisation of data, while maintaining stringent privacy standards, ultimately paving the way for more reliable and efficient data-driven insights. Findings from the study will address critical challenges in federated learning, particularly on issues arising from non-uniformly distributed data (non-IID) and the scarcity of labelled data in real-world scenarios. Dr Ragab’s
approach is instrumental for sectors like Singapore’s aviation industry, where data fragmentation from varying commercial and privacy interests is prevalent.
|
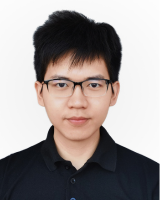 Du Jiawei Senior Research Engineer
|
Leverage Foundation Models for Modular Dataset Distillation
Existing methods unvaryingly capture knowledge in every stage from the entire dataset, leading to poor distillation performance and expensive training
cost. Dr Du’s proposal aims to improve the efficiency and effectiveness of dataset distillation. His work employs a divide-and-conquer strategy, selectively condensing knowledge from various training phases and utilising
foundation models to create new data from these condensed subsets. This involves synthesising smaller datasets for training deep neural networks (DNNs), with the aim of decreasing data storage and training expenses. These synthetic
datasets are designed to encapsulate the core knowledge of larger, real-world datasets, thereby achieving comparable performance. Dr Du’s work holds potential for significant reduction in computational demands and memory
requirements, while potentially enhancing performance. |
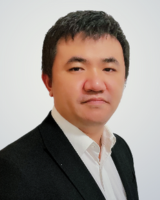 Guo Qing
Senior Scientist
|
Seeing the Unseen: Unlocking Trustworthy Active Visual Tracking via Bio-Vision-inspired Visual Implicit Representation
While active visual tracking (AVT) uses agents (e.g., mobile cameras in UAVs) to localise objects in real time with adjustable angles, its reliability remains a challenge due to real-world complexities. On the contrary, biological
vision adapts reliably to challenging conditions. To enhance the performance of AVT, Dr Guo aims to merge biological vision and agent mobility by creating a bio-vision inspired implicit representation (BViR). The BViR enables an
online framework that enhances reliability by reconstructing signals and selecting optimal view angles. In addition, a collaborative AVT will be built using BViR to accommodate multiple agents. With a reliable AVT, key intelligent
systems in Singapore, such as transportation, urban solutions and national defense can be further enhanced. |
Yin HaiyanSenior Scientist |
Towards Meta Continuous Reinforcement Learning Agents for Solving Text-based Games
Dr Yin’s project involves redefining the landscape of reinforcement learning (RL) paradigms in text-based domains.
She aims to formulate a sequential generative policy model bolstered by a decomposed latent space, with an emphasis on enabling efficient knowledge transfer while addressing the critical challenge of mitigating forgetting. This
new framework will lay a solid foundation for exploring multi-task learning and policy generalisation within the novel research trajectory of text-based RL. |