Conformal Time-series Forecasting
[CFAR Outstanding PhD Student Seminar Series]
Conformal Time-series Forecasting by Ms Kamilė Stankevičiūtė
2 Sep 2022 | 4.00pm (Singapore Time)
Current approaches for (multi-horizon) time-series forecasting using recurrent neural networks (RNNs) focus on issuing point estimates, which are insufficient for informing decision-making in critical application domains wherein uncertainty estimates are also required. Most existing methods (such as Bayesian recurrent neural networks, quantile regression models, latent variable models with deep state-space architectures, and post-hoc uncertainty estimates) share at least one of the two major drawbacks: (1) they require substantial modifications to the underlying model architecture, and (2) they provide no theoretical guarantees on frequentist coverage, with exceptions being computationally intractable.
In this talk, Ms Kamilė Stankevičiūtė from the University of Cambridge will present a conformal forecasting architecture which extends inductive conformal prediction (ICP) to the multi-horizon time-series forecasting problem, providing a lightweight algorithm for computing forecast uncertainty intervals with frequentist coverage guarantees.
SPEAKER
Conformal Time-series Forecasting by Ms Kamilė Stankevičiūtė
2 Sep 2022 | 4.00pm (Singapore Time)
Current approaches for (multi-horizon) time-series forecasting using recurrent neural networks (RNNs) focus on issuing point estimates, which are insufficient for informing decision-making in critical application domains wherein uncertainty estimates are also required. Most existing methods (such as Bayesian recurrent neural networks, quantile regression models, latent variable models with deep state-space architectures, and post-hoc uncertainty estimates) share at least one of the two major drawbacks: (1) they require substantial modifications to the underlying model architecture, and (2) they provide no theoretical guarantees on frequentist coverage, with exceptions being computationally intractable.
In this talk, Ms Kamilė Stankevičiūtė from the University of Cambridge will present a conformal forecasting architecture which extends inductive conformal prediction (ICP) to the multi-horizon time-series forecasting problem, providing a lightweight algorithm for computing forecast uncertainty intervals with frequentist coverage guarantees.
SPEAKER
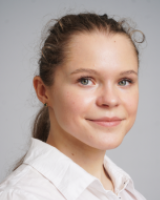
Ms Kamilė Stankevičiūtė
PhD student, Machine Learning for Medicine
Department of Applied Mathematics and Theoretical Physics
University of Cambridge
PhD student, Machine Learning for Medicine
Department of Applied Mathematics and Theoretical Physics
University of Cambridge
Ms Kamilė Stankevičiūtė received her BA in Computer Science from the University of Cambridge and an MSc in the same field from the University of Oxford, completing three software engineering internships at Google through the summers in between. She is currently in the first year of her PhD as part of the van der Schaar lab (University of Cambridge), where she is working on the applications of machine learning to healthcare.
A*STAR celebrates International Women's Day
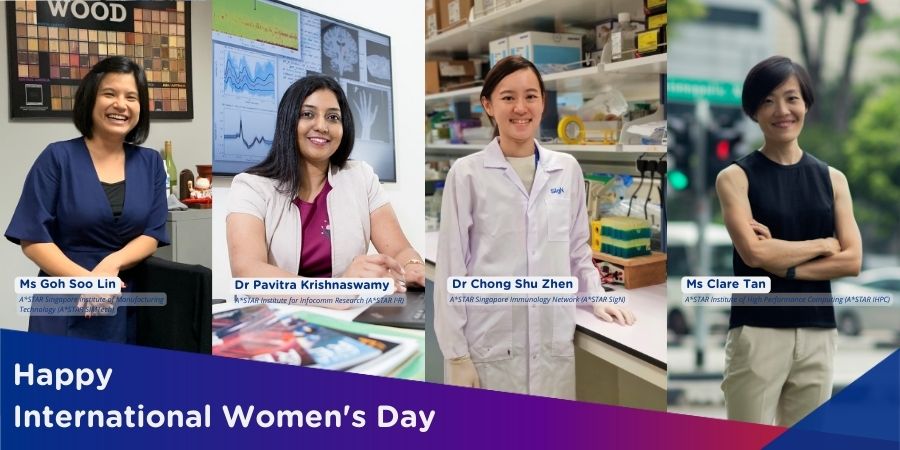
From groundbreaking discoveries to cutting-edge research, our researchers are empowering the next generation of female science, technology, engineering and mathematics (STEM) leaders.
Get inspired by our #WomeninSTEM