Distribution-Shift Robustness via Diversity and Non-Learning
[CFAR Outstanding PhD Student Seminar Series]
Distribution-Shift Robustness via Diversity and Non-Learning by Lee Yoonho
Real-world conditions are non-stationary, rather than static. When deployed in the wild, machine learning (ML) systems encounter distribution shifts, i.e., conditions that differ from what was seen during training. Conventional machine learning systems often struggle to extrapolate to new data distributions, consequently leading to severe failures.
In this talk, Lee Yoonho from Stanford University will present two methods that make ML models more robust to distribution shifts based on the principles of diversity (considering all explanations) and non-learning (not adapting more than necessary).
SPEAKER
Distribution-Shift Robustness via Diversity and Non-Learning by Lee Yoonho
17 Aug 2023 | 10.00am (Singapore Time)
Real-world conditions are non-stationary, rather than static. When deployed in the wild, machine learning (ML) systems encounter distribution shifts, i.e., conditions that differ from what was seen during training. Conventional machine learning systems often struggle to extrapolate to new data distributions, consequently leading to severe failures.
In this talk, Lee Yoonho from Stanford University will present two methods that make ML models more robust to distribution shifts based on the principles of diversity (considering all explanations) and non-learning (not adapting more than necessary).
SPEAKER
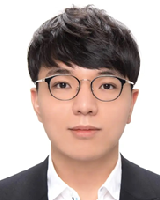
Lee Yoonho
Ph.D. Student
Stanford University
Ph.D. Student
Stanford University
Lee Yoonho is a second-year PhD student at Stanford University, under the supervision of Prof. Chelsea Finn. His research focuses on developing models capable of learning and decision-making in changing environments. He is also a Korea Foundation for Advanced Studies Doctoral Fellow.
A*STAR celebrates International Women's Day
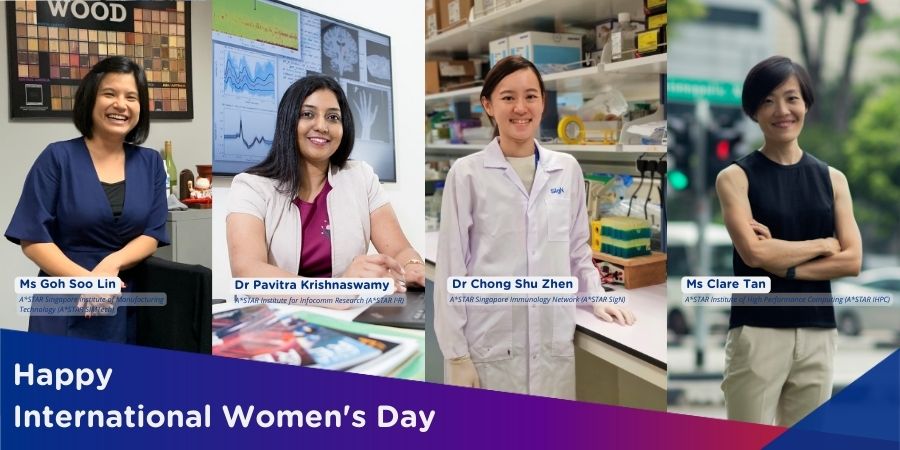
From groundbreaking discoveries to cutting-edge research, our researchers are empowering the next generation of female science, technology, engineering and mathematics (STEM) leaders.
Get inspired by our #WomeninSTEM