Effective and Efficient Continual Learning
[CFAR Outstanding PhD Student Seminar Series]
Effective and Efficient Continual Learning by Wang Zifeng
Continual Learning (CL) aims to develop models that mimic the human ability to learn continually without forgetting the knowledge acquired earlier. While traditional machine learning methods focus on learning with a certain dataset (i.e., task), CL methods adopt a single model to learn a sequence of tasks continually. In this talk, Wang Zifeng from Northeastern University will share how his team develops effective and efficient CL methods under different challenging and resource-limited settings. First, he will present the novel prompting-based paradigm for parameter-efficient CL. It leverages the idea of sparsity to achieve cost-effective CL that aims to train a more succinct memory system that is both data and memory efficient. A method was then introduced to dynamically prompt (Learning to Prompt, CVPR 2022) a pre-trained model to learn tasks sequentially under different task transitions, where prompts are small learnable parameters maintained in a memory space. To improve Learning to Prompt (L2P), his team proposed ‘DualPrompt’ (ECCV 2022) that decouples prompts into complementary “General” and “Expert” prompts to learn task-invariant and task-specific instructions, respectively. For the second segment, Zifeng will present Sparse Continual Learning (SparCL, NeurIPS 2022) - a novel framework that leverages sparsity to enable cost-effective continual learning on edge devices. He will discuss how SparCL could achieve both training acceleration and accuracy preservation through the synergy of three aspects: weight sparsity, data efficiency, and gradient sparsity. Lastly, he will conclude the effectiveness and efficiency of his team’s methods over the state-of-the-art methods on multiple CL benchmarks.
SPEAKER
Effective and Efficient Continual Learning by Wang Zifeng
19 Jul 2023 | 9.00am (Singapore Time)
Continual Learning (CL) aims to develop models that mimic the human ability to learn continually without forgetting the knowledge acquired earlier. While traditional machine learning methods focus on learning with a certain dataset (i.e., task), CL methods adopt a single model to learn a sequence of tasks continually. In this talk, Wang Zifeng from Northeastern University will share how his team develops effective and efficient CL methods under different challenging and resource-limited settings. First, he will present the novel prompting-based paradigm for parameter-efficient CL. It leverages the idea of sparsity to achieve cost-effective CL that aims to train a more succinct memory system that is both data and memory efficient. A method was then introduced to dynamically prompt (Learning to Prompt, CVPR 2022) a pre-trained model to learn tasks sequentially under different task transitions, where prompts are small learnable parameters maintained in a memory space. To improve Learning to Prompt (L2P), his team proposed ‘DualPrompt’ (ECCV 2022) that decouples prompts into complementary “General” and “Expert” prompts to learn task-invariant and task-specific instructions, respectively. For the second segment, Zifeng will present Sparse Continual Learning (SparCL, NeurIPS 2022) - a novel framework that leverages sparsity to enable cost-effective continual learning on edge devices. He will discuss how SparCL could achieve both training acceleration and accuracy preservation through the synergy of three aspects: weight sparsity, data efficiency, and gradient sparsity. Lastly, he will conclude the effectiveness and efficiency of his team’s methods over the state-of-the-art methods on multiple CL benchmarks.
SPEAKER
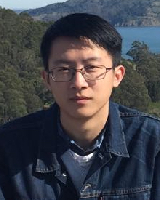
Wang Zifeng
Ph.D. Student
Northeastern University
Ph.D. Student
Northeastern University
Wang Zifeng is a Ph.D. student at Northeastern University. He received his B.S. degree in Electronic Engineering from Tsinghua University. His research interests include continual (lifelong) learning, data-efficient and parameter-efficient learning, large language models, and real-world machine learning applications. Zifeng will be joining Google as a research scientist after his graduation at the end of July.
A*STAR celebrates International Women's Day
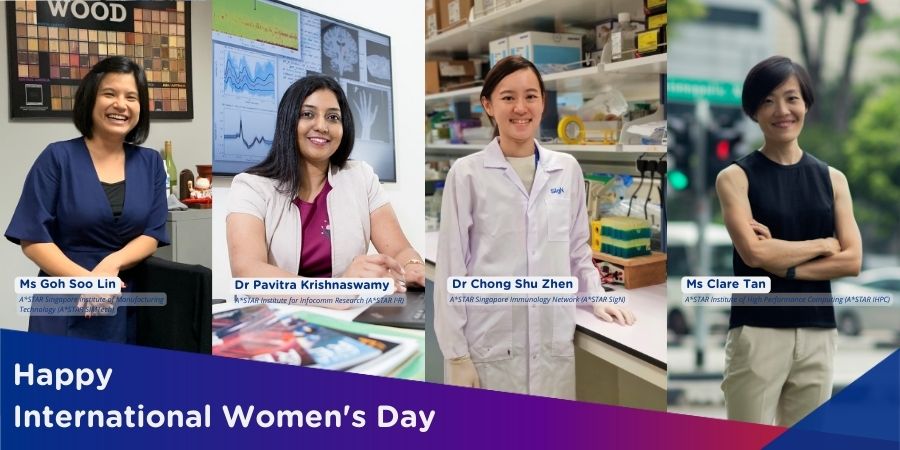
From groundbreaking discoveries to cutting-edge research, our researchers are empowering the next generation of female science, technology, engineering and mathematics (STEM) leaders.
Get inspired by our #WomeninSTEM