Embedding Physical Knowledge into Deep Learning Models for Efficient and Robust Learning
[CFAR Outstanding PhD Student Seminar Series]
Embedding Physical Knowledge into Deep Learning Models for Efficient and Robust Learning by Dr. Filipe de Avila Belbute-Peres
4 Nov 2022 | 9.00am (Singapore Time)
The recent successes of deep learning methods have motivated their employment in diverse domains, including physical tasks such as predicting the motion of objects or the behaviour of fluids. However, in order to learn properly, deep learning methods require large amounts of data, which can be costly in certain domains. Moreover, they often do not generalise properly to domains not previously seen in the training data.
By embedding traditional physics models into deep learning architectures, Dr. Filipe de Avila Belbute-Peres from Carnegie Mellon University will introduce ways to address these issues that allow for more efficient and robust learning.
Embedding Physical Knowledge into Deep Learning Models for Efficient and Robust Learning by Dr. Filipe de Avila Belbute-Peres
4 Nov 2022 | 9.00am (Singapore Time)
The recent successes of deep learning methods have motivated their employment in diverse domains, including physical tasks such as predicting the motion of objects or the behaviour of fluids. However, in order to learn properly, deep learning methods require large amounts of data, which can be costly in certain domains. Moreover, they often do not generalise properly to domains not previously seen in the training data.
By embedding traditional physics models into deep learning architectures, Dr. Filipe de Avila Belbute-Peres from Carnegie Mellon University will introduce ways to address these issues that allow for more efficient and robust learning.
SPEAKER
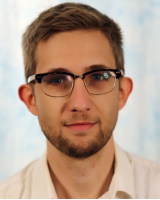
Dr. Filipe de Avila Belbute-Peres
PhD Student
Carnegie Mellon University
PhD Student
Carnegie Mellon University
Dr. Filipe de Avila Belbute-Peres is a PhD student in Computer Science at Carnegie Mellon University (CMU), where he studies approaches to combine physics knowledge and deep learning methods under the supervision of Prof. Zico Kolter. He also interned in Google and Bosch, where he worked with Dr. Fei Sha, Dr. Yifan Chen and Dr. Thomas Economon.
A*STAR celebrates International Women's Day
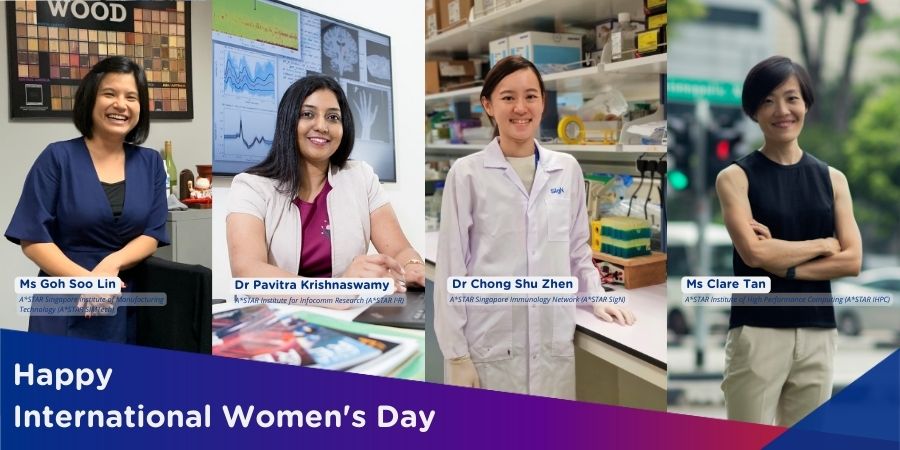
From groundbreaking discoveries to cutting-edge research, our researchers are empowering the next generation of female science, technology, engineering and mathematics (STEM) leaders.
Get inspired by our #WomeninSTEM