Ensembling over Classifiers: a Bias-Variance Perspective
[CFAR Rising Star Lecture]
Ensembling over Classifiers: a Bias-Variance Perspective by Dr Zelda Mariet
24 Mar 2022 | 8:30am (Singapore Time)
The bias-variance decomposition is a foundational tool to understand the behavior of machine learning algorithms. Albeit well-known for the squared Euclidean loss, the bias-variance decomposition takes a more opaque form in the general case. This has severely limited the theoretical analyses of models for which the Euclidean squared loss is ill-suited, including the entirety of models used for classification tasks.
In this talk, Dr Zelda Mariet from Google Brain, will show that a simple dual reparameterisation of the bias-tradeoff drastically simplifies its analysis. Based on this reparameterisation, she will characterise the behaviour of bias and variance terms for a broad class of losses, aiming to provide a unified understanding of how different sources of randomness affect machine learning algorithms.
In particular, Dr Zelda will use the bias-variance tradeoff to explain why the behaviour of ensembles of classification models breaks away from conventional wisdom, and how we can recover standard behaviours under alternate ensembling techniques.
Ensembling over Classifiers: a Bias-Variance Perspective by Dr Zelda Mariet
24 Mar 2022 | 8:30am (Singapore Time)
The bias-variance decomposition is a foundational tool to understand the behavior of machine learning algorithms. Albeit well-known for the squared Euclidean loss, the bias-variance decomposition takes a more opaque form in the general case. This has severely limited the theoretical analyses of models for which the Euclidean squared loss is ill-suited, including the entirety of models used for classification tasks.
In this talk, Dr Zelda Mariet from Google Brain, will show that a simple dual reparameterisation of the bias-tradeoff drastically simplifies its analysis. Based on this reparameterisation, she will characterise the behaviour of bias and variance terms for a broad class of losses, aiming to provide a unified understanding of how different sources of randomness affect machine learning algorithms.
In particular, Dr Zelda will use the bias-variance tradeoff to explain why the behaviour of ensembles of classification models breaks away from conventional wisdom, and how we can recover standard behaviours under alternate ensembling techniques.
SPEAKER
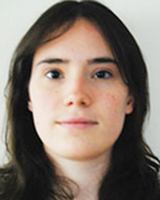
Dr Zelda Mariet
PhD, Massachusetts Institute of Technology (MIT)
Researcher, Google Brain
PhD, Massachusetts Institute of Technology (MIT)
Researcher, Google Brain
Zelda Mariet received her PhD from Massachusetts Institute of Technology (MIT) in 2019 for her work on the theory and applications of negatively dependent measures to machine learning. As a researcher at Google Brain, her current research focuses on the mathematical analysis of how diversity in ensembles of deep learning models can improve robustness to dataset shift. Recently, she has been focusing on dataset shifts specific to biochemistry applications, including protein design and drug discovery.
A*STAR celebrates International Women's Day
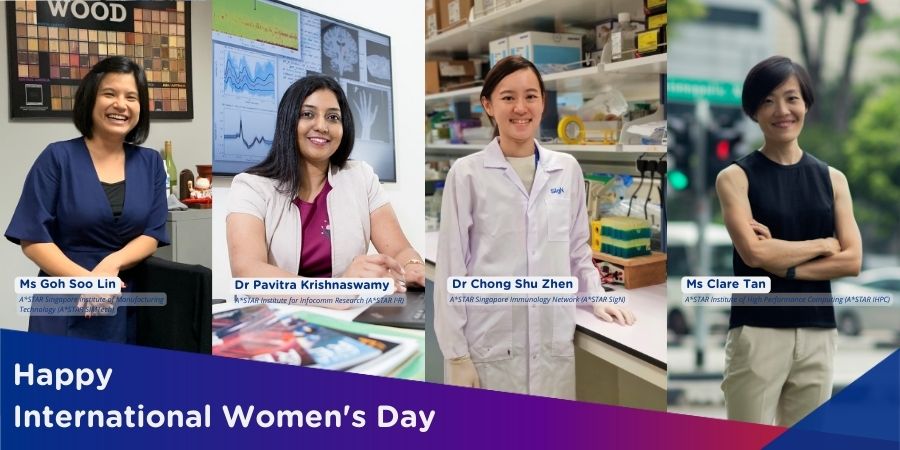
From groundbreaking discoveries to cutting-edge research, our researchers are empowering the next generation of female science, technology, engineering and mathematics (STEM) leaders.
Get inspired by our #WomeninSTEM