Incorporating Diversity and Consistency in Semi-supervised Learning
[CFAR Outstanding PhD Student Seminar Series]
Incorporating Diversity and Consistency in Semi-supervised Learning (Hybrid event) by Mr Arun Raja
9 Sep 2022 | 2.00pm (Singapore Time)
Deep neural networks have achieved tremendous success across several domains, ranging from computer vision, natural language processing, to audio analysis. However, training neural networks to perform well typically requires a large amount of labelled data. In many cases, this requirement for a large, labelled dataset presents a challenge, as the annotation process can be labour-intensive and thus expensive, especially when specialised expertise is required. To address this challenge, semi-supervised learning methods that could achieve similarly high performance with less labelled data by using unlabelled data have been developed.
Several semi-supervised learning methods in the regression setting harness the principle of consistency which reinforce the output consistency of the network under input perturbations. To ensure good performance, enforcing consistency alone may not be sufficient and could result in model collapsing or confirmation bias issues. In addition, the accuracy of the base learners play an important condition in the ensemble of learners and the key component that could make or break an ensemble is the diversity (or disagreement) among its individual regressors.
In this talk, Mr Arun Raja will address how he and his team at A*STAR’s Institute for Infocomm Research (I2R) have developed a novel technique, named Diverse and Consistent Multi-view Networks or DiCoM based on the multi-view learning framework for semi-supervised regression. The talk will cover the related work and the motivation behind developing the technique and its specifics. He will also discuss the experimental results across various data modalities such as tabular, visual and time-series along with possible future research directions.
Incorporating Diversity and Consistency in Semi-supervised Learning (Hybrid event) by Mr Arun Raja
9 Sep 2022 | 2.00pm (Singapore Time)
Deep neural networks have achieved tremendous success across several domains, ranging from computer vision, natural language processing, to audio analysis. However, training neural networks to perform well typically requires a large amount of labelled data. In many cases, this requirement for a large, labelled dataset presents a challenge, as the annotation process can be labour-intensive and thus expensive, especially when specialised expertise is required. To address this challenge, semi-supervised learning methods that could achieve similarly high performance with less labelled data by using unlabelled data have been developed.
Several semi-supervised learning methods in the regression setting harness the principle of consistency which reinforce the output consistency of the network under input perturbations. To ensure good performance, enforcing consistency alone may not be sufficient and could result in model collapsing or confirmation bias issues. In addition, the accuracy of the base learners play an important condition in the ensemble of learners and the key component that could make or break an ensemble is the diversity (or disagreement) among its individual regressors.
In this talk, Mr Arun Raja will address how he and his team at A*STAR’s Institute for Infocomm Research (I2R) have developed a novel technique, named Diverse and Consistent Multi-view Networks or DiCoM based on the multi-view learning framework for semi-supervised regression. The talk will cover the related work and the motivation behind developing the technique and its specifics. He will also discuss the experimental results across various data modalities such as tabular, visual and time-series along with possible future research directions.
SPEAKER
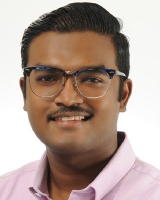
Mr Arun Raja
BSc. (Hons), Artificial Intelligence and Computer Science
Machine Learning Research Engineer
Institute for Infocomm Research (I2R), A*STAR
BSc. (Hons), Artificial Intelligence and Computer Science
Machine Learning Research Engineer
Institute for Infocomm Research (I2R), A*STAR
Mr Arun Raja graduated with a BSc. (Hons) in Artificial Intelligence and Computer Science in 2021 from the School of Informatics, University of Edinburgh, under the National Science Scholarship (Bachelors). He was recently awarded the National Science Scholarship (PhD). He will be pursuing his PhD at the University of Oxford in October 2022, specialising in developing Machine Learning methods for biomedical applications. He is currently a Machine Learning Research Engineer in the Data-Efficient Learning group at A*STAR’s Institute for Infocomm Research (I2R).
A*STAR celebrates International Women's Day
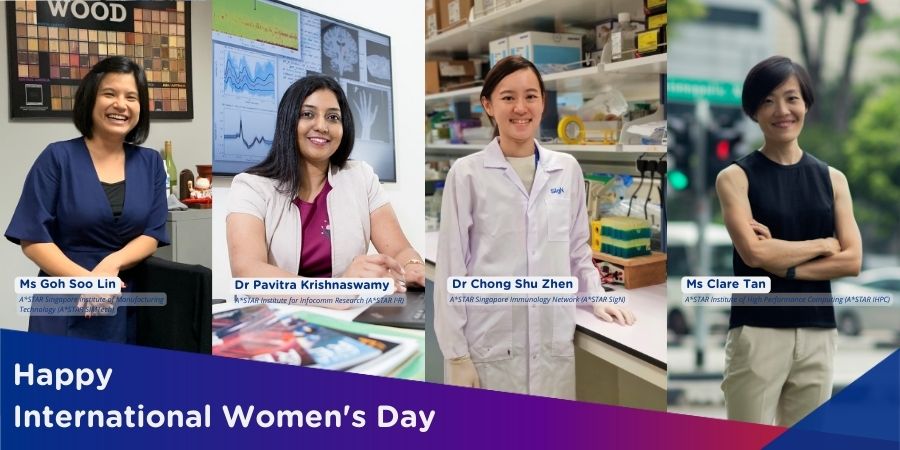
From groundbreaking discoveries to cutting-edge research, our researchers are empowering the next generation of female science, technology, engineering and mathematics (STEM) leaders.
Get inspired by our #WomeninSTEM