Learned Models for Efficient Simulation and Design
[CFAR Rising Star Lecture Series]
Learned Models for Efficient Simulation and Design by Dr Kimberly Stachenfeld
Simulation is important for numerous applications in science and engineering, and there has been increasing interest in using machine learning to produce more efficient learned simulators, distill complex dynamics into a differentiable model, or learn simulators from real world data. In the first part of this talk, Dr Kimberly Stachenfeld from DeepMind will describe her group’s approach to using deep learning for learned physics simulators, with particular focus on work that uses learned models for efficient, accurate, stable simulation of fluids and other complex physical dynamics in particle-, mesh-, and grid-based domains.
In the latter part of the talk, Dr Stachenfeld will discuss her group’s recent work using learned simulators for inverse design, where they combined their graph-based learned simulators with gradient-based optimisation in order to optimise designs in a variety of complex physics tasks. These include challenges faced when designing objects in 2D and 3D to direct fluids in complex ways, as well as optimising the shape of an airfoil. The group found that the learned models can not only support design optimisation across 100s of timesteps, these models can in some cases, permit designs that lead to dynamics that are apparently quite different from the training data. Dr Stachenfeld will then conclude the talk with a discussion on some of the future directions for learned simulation.
Learned Models for Efficient Simulation and Design by Dr Kimberly Stachenfeld
12 Oct 2022 | 4.00pm (Singapore Time)
Simulation is important for numerous applications in science and engineering, and there has been increasing interest in using machine learning to produce more efficient learned simulators, distill complex dynamics into a differentiable model, or learn simulators from real world data. In the first part of this talk, Dr Kimberly Stachenfeld from DeepMind will describe her group’s approach to using deep learning for learned physics simulators, with particular focus on work that uses learned models for efficient, accurate, stable simulation of fluids and other complex physical dynamics in particle-, mesh-, and grid-based domains.
In the latter part of the talk, Dr Stachenfeld will discuss her group’s recent work using learned simulators for inverse design, where they combined their graph-based learned simulators with gradient-based optimisation in order to optimise designs in a variety of complex physics tasks. These include challenges faced when designing objects in 2D and 3D to direct fluids in complex ways, as well as optimising the shape of an airfoil. The group found that the learned models can not only support design optimisation across 100s of timesteps, these models can in some cases, permit designs that lead to dynamics that are apparently quite different from the training data. Dr Stachenfeld will then conclude the talk with a discussion on some of the future directions for learned simulation.
SPEAKER
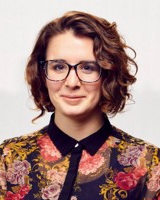
Dr Kimberly Stachenfeld
Research Scientist
DeepMind
Research Scientist
DeepMind
Dr Kimberly Stachenfeld is a Research Scientist at DeepMind. She received her PhD in Computational Neuroscience from Princeton University in 2018. Before that, she received her Bachelors from Tufts University in 2013, where she majored in Chemical & Biological Engineering and Mathematics. Her research is at the interface of Neuroscience and Machine Learning, focusing on graph-based computations for efficient learning and planning in brains and in machines.
A*STAR celebrates International Women's Day
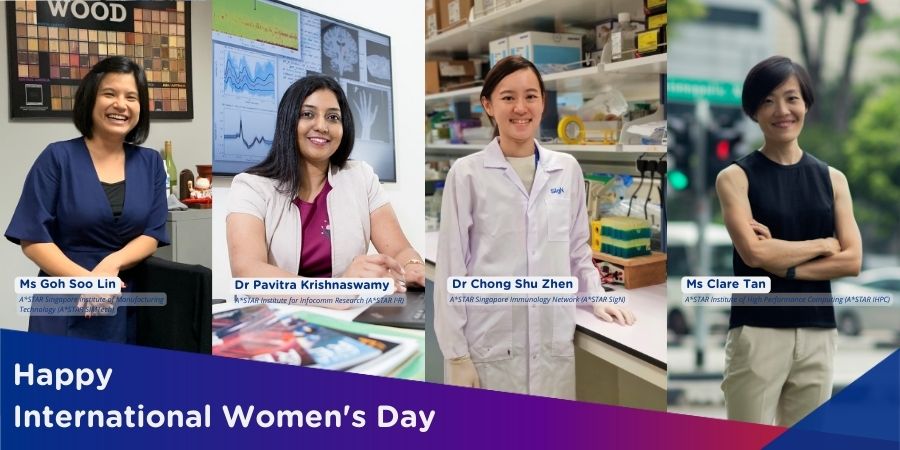
From groundbreaking discoveries to cutting-edge research, our researchers are empowering the next generation of female science, technology, engineering and mathematics (STEM) leaders.
Get inspired by our #WomeninSTEM