Learning Manifold Dimensions with Conditional Variational Autoencoders
[CFAR Rising Star Lecture Series]
Learning Manifold Dimensions with Conditional Variational Autoencoders (Hybrid event) by Ms Zheng Yijia
The variational autoencoder (VAE) and its conditional extension (CVAE) have been found to be capable of achieving state-of-the-art results across multiple domains, however, their precise behaviour is still not fully understood, particularly in the context of data (like images) that lies on or near a low-dimensional manifold. For example, while prior work has suggested that the globally optimal VAE solution could learn the correct manifold dimension, a necessary (but not sufficient) condition for producing samples from the true data distribution, this has never been rigorously proven. Moreover, it remains unclear how such considerations would change when various types of conditioning variables are introduced, or when the data support is extended to a union of manifolds.
In this talk, Zheng Yijia will share her insights on the latent space behaviour in VAE/CVAE models and demonstrate practical scenarios where the conditioning variables would allow the model to adaptively learn manifolds of varying dimensions across samples. She will then conclude the talk by introducing some common design choices for these models.
Learning Manifold Dimensions with Conditional Variational Autoencoders (Hybrid event) by Ms Zheng Yijia
15 Mar 2023 | 11.00am (Singapore Time)
The variational autoencoder (VAE) and its conditional extension (CVAE) have been found to be capable of achieving state-of-the-art results across multiple domains, however, their precise behaviour is still not fully understood, particularly in the context of data (like images) that lies on or near a low-dimensional manifold. For example, while prior work has suggested that the globally optimal VAE solution could learn the correct manifold dimension, a necessary (but not sufficient) condition for producing samples from the true data distribution, this has never been rigorously proven. Moreover, it remains unclear how such considerations would change when various types of conditioning variables are introduced, or when the data support is extended to a union of manifolds.
In this talk, Zheng Yijia will share her insights on the latent space behaviour in VAE/CVAE models and demonstrate practical scenarios where the conditioning variables would allow the model to adaptively learn manifolds of varying dimensions across samples. She will then conclude the talk by introducing some common design choices for these models.
SPEAKER
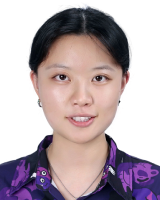
Zheng Yijia
PhD Student
Purdue University
PhD Student
Purdue University
Zheng Yijia is a first year PhD student in Statistics at Purdue University. She is currently doing an applied scientist internship with Amazon Web Services (AWS), under the supervision of Dr. David Wipf and Mr. Tong He. She completed her Bachelor’s degree in Data Science at Shanghai University of Finance and Economics in 2022, where she was supervised by Dr. Yixuan Qiu. Yijia’s current research interests are Deep Generative Models and Graph Machine Learning.
A*STAR celebrates International Women's Day
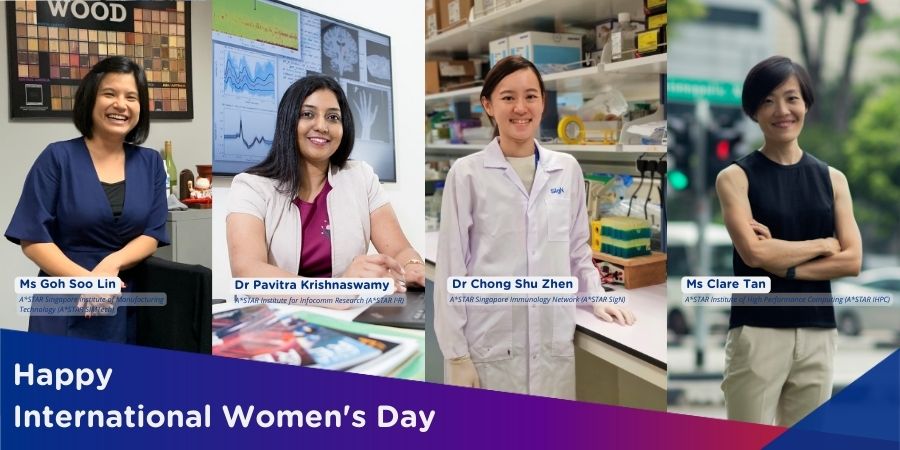
From groundbreaking discoveries to cutting-edge research, our researchers are empowering the next generation of female science, technology, engineering and mathematics (STEM) leaders.
Get inspired by our #WomeninSTEM