Computer Vision
Our researchers push the boundaries of computer vision while staying attuned to Singapore’s evolving technological needs. Through AI-powered image and video analysis, object recognition, and scene understanding, we develop solutions that enhance efficiency, safety, and automation across various industries such as healthcare and medical imaging, smart cities and urban mobility, security and surveillance etc.
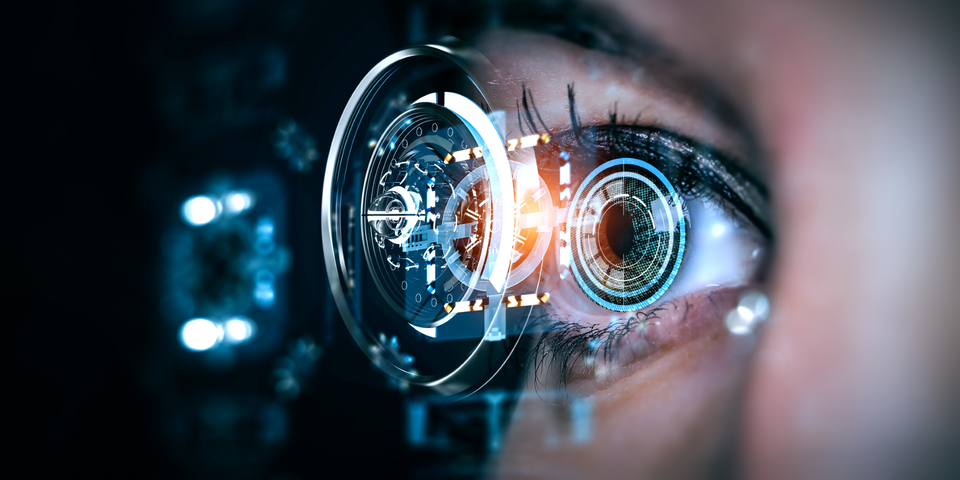
Key Researchers
- Lim Joo Hwee, A*STAR Institute for Infocomm Research (A*STAR I2R)
- Dr Zhang Mengmi, A*STAR Institute for Infocomm Research (A*STAR I2R)
- Dr Cheng Jun, A*STAR Institute for Infocomm Research (A*STAR I2R)
- Dr Lee Hwee Kuan, A*STAR Bioinformatics Institute (A*STAR BII)
- Dr Yu Weimiao, A*STAR Bioinformatics Institute (A*STAR BII)
Key Projects
A*STAR celebrates International Women's Day
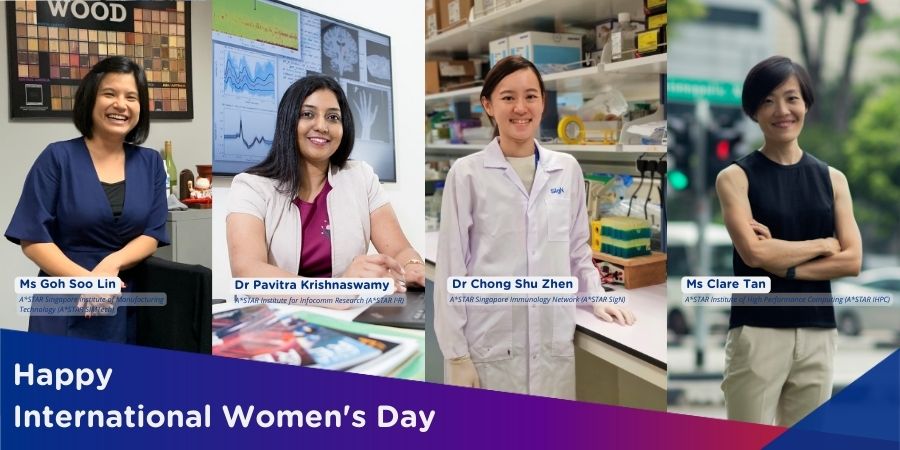
From groundbreaking discoveries to cutting-edge research, our researchers are empowering the next generation of female science, technology, engineering and mathematics (STEM) leaders.
Get inspired by our #WomeninSTEM