Intelligent Digital and Molecular Pathology
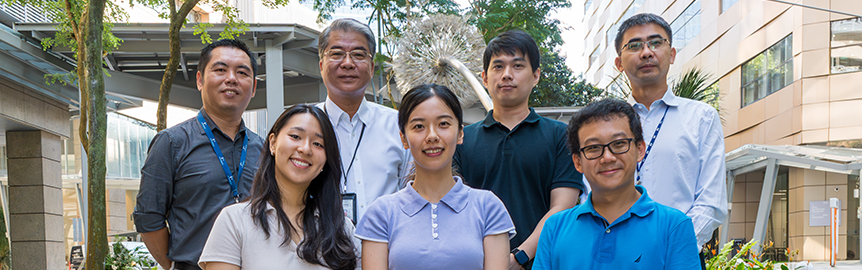
YU Weimiao
![]() | YU WeiMiao Principal Scientist Email: yu_weimiao@bii.a-star.edu.sg Research Group: Intelligent Digital and Molecular Pathology |
Dr. Yu Weimiao, an expert in bioimage informatics and computational digital pathology, leads two pioneering groups as a joint Principal Investigator at BII and IMCB. After his Ph.D. in image processing and machine vision from the National University of Singapore, Dr. Yu has been with A*STAR since 2007, advancing to lead the Computational Digital Pathology Lab at BII and the Computational & Molecular Pathology Lab at IMCB. His research, published in top peer reviewed journals like Nature Cell Biology, Nature Materials, Nature Communications, Bioinformatics, focuses on AI-driven computational biomedical image analysis and quantitative imaging informatics.
Dr. Yu’s teams, with over 18 years of experience, excel in developing robust cellular image analysis and molecular diagnosis solutions for clinical application. They integrate diverse methodologies, including signal processing, machine learning, and AI, to create accurate and efficient digital pathology algorithms. Their collaborations with hospitals, biotech, and pharmaceutical companies enhance their expertise in medical algorithm development and validation, aimed at improving patient care. His previous research outcomes were successfully commercialized and made an impact in the medical diagnosis and drug discovery.
An entrepreneur as well, Dr. Yu co-founded A!maginostic Pte. Ltd. to advance the application of AI in clinical diagnostics. He collaborates closely with Singapore General Hospital, National University Hospital, and Tan Tock Seng Hospital, ensuring a seamless R&D pipeline. His world-class joint lab for immunodiagnosis at the tissue level provides intelligent, quantitative imaging analysis solutions, including ImmunoHistoChemistry, Hematoxylin & Eosin, and Multiplex Fluorescence stained images. This integrated platform enables comprehensive profiling of patient immune signatures, aiding in diagnosis, prognosis, and drug response studies.
THE JOINT LAB OF EXCELLENCE IS PARKED AT A*STAR RESEARCH SUPPORT CENTER:
https://www.rsc.a-star.edu.sg/technologyplatforms/scientific-side-menu/scientific-information/histopathology/model-based-digital-pathology-services
Group Members
Senior Scientist | ONG Kok Haur |
Scientist | YUAN Chengxiang |
Scientist | LU Haoda |
Senior Research Officer | HUO Xinmi |
Senior Research Officer | LI Longjie |
Research Officer | PENG Aisha |
PhD Student | YOO Sehwan |
Visiting Scientist | CHEN Wanyuan |
A*STAR celebrates International Women's Day
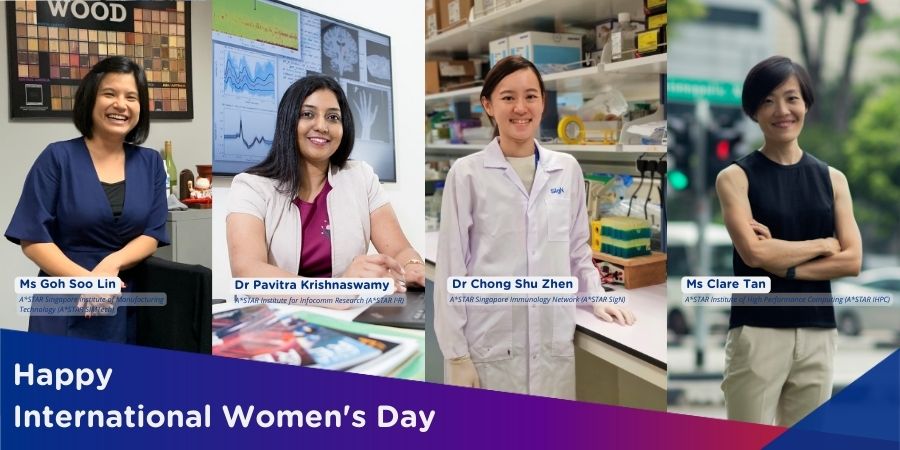
From groundbreaking discoveries to cutting-edge research, our researchers are empowering the next generation of female science, technology, engineering and mathematics (STEM) leaders.
Get inspired by our #WomeninSTEM