Data Management
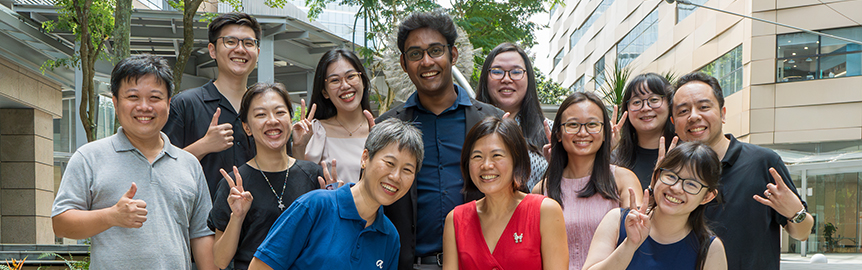
P Mukkesh Kumar
![]() | P Mukkesh KUMAR Lead Research Officer Email: mukkesh_kumar@bii.a-star.edu.sg Research Group: Data Management |
Dr P Mukkesh Kumar is the Head of Data Management Platform at A*STAR’s Bioinformatics Institute (BII) and Singapore Institute for Clinical Sciences (SICS), leading the Multi-modal Data Management, Data Curation & Data Stewardship, Healthcare Data Analytics & Reporting and Healthcare Software Development teams. Dr P Mukkesh Kumar is a PhD alumnus of the NUS Saw Swee Hock School of Public Health, he has developed a predictive care framework for diabetes & maternal health, combining coalitional game theory concepts with machine learning. Forging collaborations with the National University Hospital (NUH) in Value-based Healthcare Strategy, the Early Screening for Gestational Diabetes Mellitus in a Low Risk Population (EaGeR) pilot study conducted at NUH for the real world deployment of early pregnancy GDM predictor AI model. Working in close partnership with Singapore’s Ministry of Health (MOH), Dr P Mukkesh Kumar is developing the core OMOP data curation team at A*STAR to support MOH-TRUST Strategic Research Data Contributors. Dr P Mukkesh Kumar has been mentoring the Data Managers at US Boston Children’s Hospital/Harvard Medical School for multi-centre clinical research studies, building talent and capabilities in the global research ecosystem.
Group Member
Lead Research Officer | ERWIN Tantoso |
Senior Research Officer | ANG Li Ting |
Senior Research Officer | CHOH Mei Kit |
Senior Research Officer | CHOO Sok Hui Sue |
Senior Research Officer | HO Xin Ying Cindy |
Senior Research Officer | ISMAIL Bin Mohd |
Senior Research Officer | NG Mei Xi Maisie |
Senior Research Officer | TAN Shuen Lin |
Senior Research Officer | YE Yingxin Estella |
Research Officer | PNG Hang |
Research Officer | TAN Jing Yang |
A*STAR celebrates International Women's Day
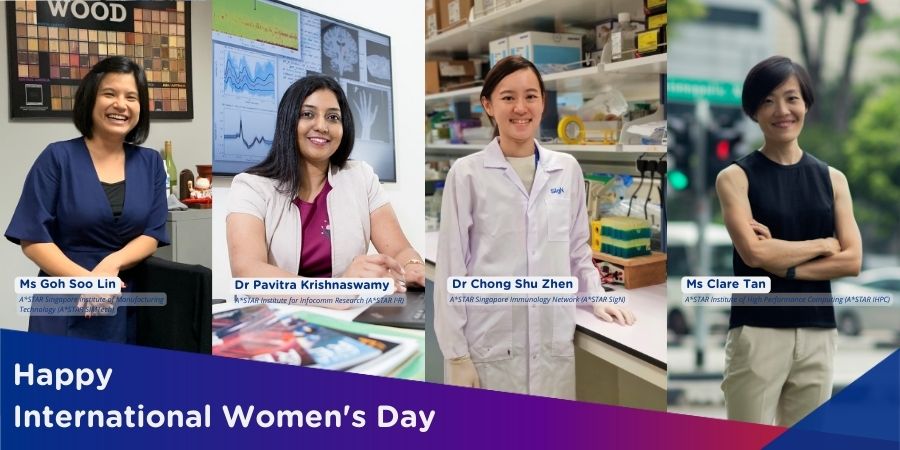
From groundbreaking discoveries to cutting-edge research, our researchers are empowering the next generation of female science, technology, engineering and mathematics (STEM) leaders.
Get inspired by our #WomeninSTEM