Computational Biology & Omics Lab
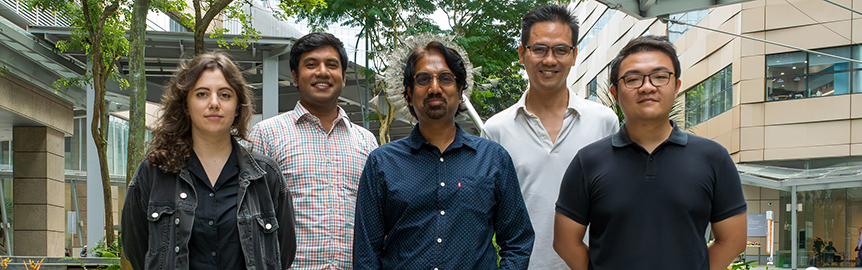
SELVARAJOO Kumar
![]() | SELVARAJOO Kumar Senior Principal Investigator Email: kumar_selvarajoo@bii.a-star.edu.sg Research Group: Computational Biology & Omics Lab Lab Website: https://www.cbio-kumar.org/home Research Gate: https://www.researchgate.net/profile/Kumar-Selvarajoo |
Dr. Kumar Selvarajoo is heading the Computational Biology & Omics laboratory at BII, A*STAR. He is also an adjunct Associate Professor at the Yong Loo Lin School of Medicine, National University of Singapore and the School of Biological Sciences, Nanyang Technological University. Prior, he was an Associate Professor in Systems Biology at the Institute for Advanced Biosciences, Keio University, Japan. He serves the editorial board of BMC Biology (SpringerNature), Genomics (Elsevier), Frontiers in Immunology (Frontiers) and Biotechnology Notes (KeAi). He leads in Computational Biology, Systems Biology, Bioinformatics, Data Analytics and Statistical Genetics. In particular, he has used original ideas, utilizing fundamental physical and statistical laws, to investigate multi-dimensional datasets, deterministic and stochastic modelling of complex protein signaling and metabolic networks. He has authored over 82 scientific articles, largely as corresponding author, which includes a single-authored book on Immuno Systems Biology (Springer) and edited a Methods in Molecular Biology book series on Computational Biology and Machine Learning for Metabolic Engineering and Synthetic Biology (Humana Press). He has obtained several research grants and has been an international grant reviewer and PhD thesis examiner. He has also presented invited/keynote talks at numerous international conferences.
Research Interests
Computational Biology, Systems Biology, Bioinformatics, Data Analytics & Machine Learning, Genomics, Cancer & Immunology, Synthetic Biology, Synthetic Data, Multi-omics Analytics
Group Members
Senior Scientist | YEO Hock Chuan |
Scientist | RASHID Md Mamunur |
Research Officer | SIRBU Olga |
PhD Student | LEE Shi Mun |
PhD Student | PABIS Kamil Konrad |
PhD Student | SIM Wen Bo Clarence |
A*STAR celebrates International Women's Day
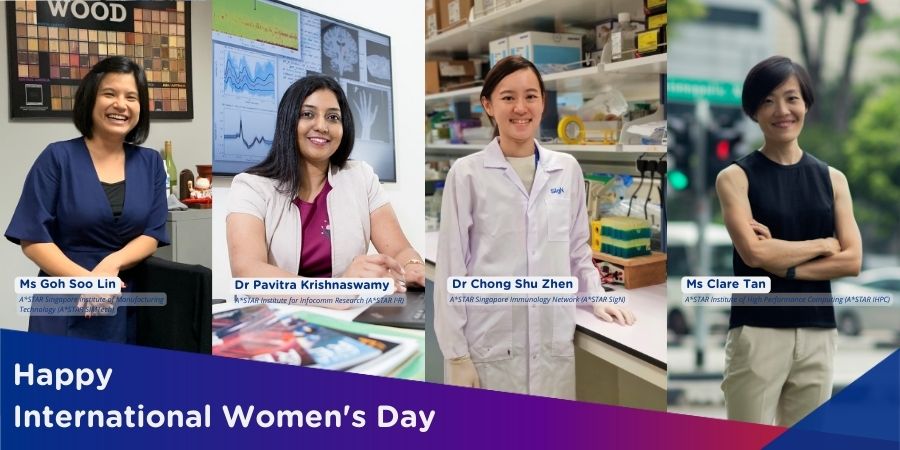
From groundbreaking discoveries to cutting-edge research, our researchers are empowering the next generation of female science, technology, engineering and mathematics (STEM) leaders.
Get inspired by our #WomeninSTEM