Structure-based Ligand Discovery and Design
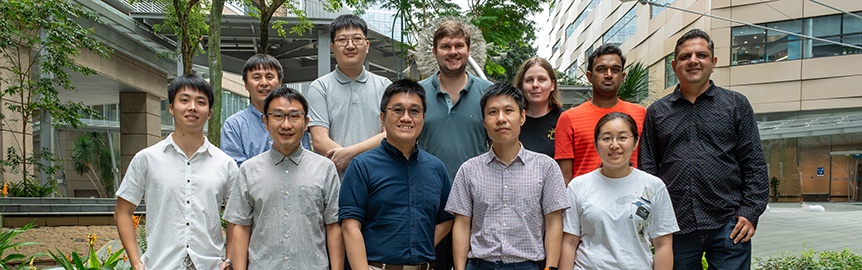
Fan Hao
![]() | FAN Hao Senior Principal Investigator Email: fanh@bii.a-star.edu.sg Research Group: Structure-based Ligand Discovery and Design |
Dr. Hao Fan received his undergraduate degree in Biological Sciences in University of Science and Technology of China (USTC). He obtained his PhD in Biophysical Chemistry in Dr. Alan Mark’s lab at University of Groningen (RUG). He worked as postdoctoral fellow followed by research scientist in both Dr. Andrej Sali’s lab and Dr. Brian Shoichet’s lab at University of California, San Francisco (UCSF). He was appointed Principal Investigator at the Bioinformatics Institute (BII), A*STAR since 2014. Currently he is Adjunct Associate Professor at Signature Research Programme in Cancer & Stem Cell Biology (“CSCB”) and Centre for Computational Biology (“CCB”), Office of Research in Duke-NUS Medical School (“Duke-NUS”).
Group Members
Senior Scientist | VERMA Ravi Kumar |
Senior Scientist (Collaborator) | LIN Fu |
Research Scientist (Adjunct) | KRISHNA DEEPAK R.N.V. |
Senior Scientist | JALADANKI Chaitany`a Kumar |
Senior Scientist | SUPEKAR Shreyas |
Post-Doctoral Research Fellow (Collaborator) | HARTONO Yossa Dwi |
Post-Doctoral Research Fellow (Collaborator) | BARBIERI Alessandro |
Scientist | WEI Wan |
Scientist | LIM Jun Yu Victor |
Research Officer | RAYAKAR Achal Ajeet |
PhD Student | TIKHONOVA Anastasiia |
PhD Student | BEHN Julian |
PhD Student | HAN Sungho Bosco |
PhD Student (co-supevisor) | WANG Kaichen |
PhD Student (co-supevisor) | PICUCCI Francesca Harriet |
A*STAR celebrates International Women's Day
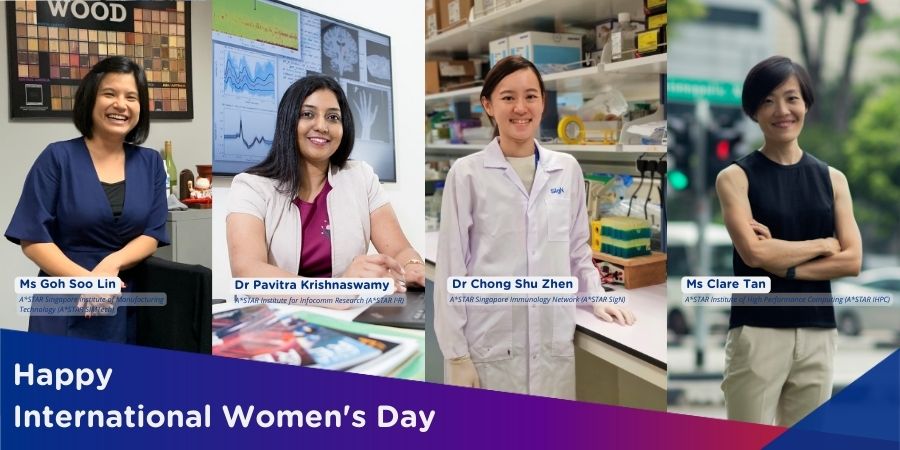
From groundbreaking discoveries to cutting-edge research, our researchers are empowering the next generation of female science, technology, engineering and mathematics (STEM) leaders.
Get inspired by our #WomeninSTEM