AI-powered Single-cell and Spatial Omics Analysis for Precision Medicine
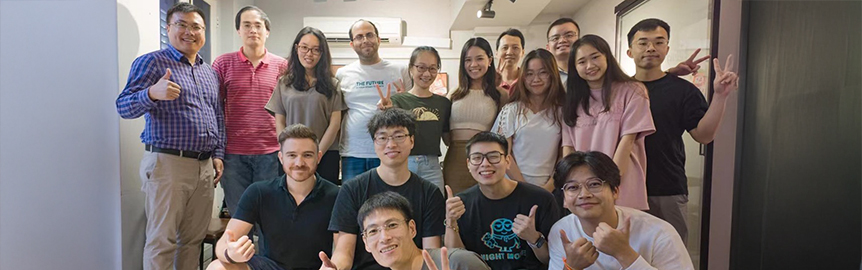
CHEN JINMIAO
![]() | CHEN Jinmiao Senior Principal Scientist Email: chen_jinmiao@bii.a-star.edu.sg Research Group: Single-Cell Multi/Spatial-Omics and Precision Technology |
Dr. Jinmiao Chen, a Senior Principal Scientist I at the Bioinformatics Institute (BII), leads cutting-edge research focused on AI-powered single-cell and spatial omics analysis. Her work is instrumental in advancing precision medicine by developing tools and methodologies that make complex biological data more accessible and interpretable for scientists worldwide.
Research Focus
Dr. Chen’s research centers around the integration of artificial intelligence with omics technologies to decipher cellular mechanisms at unprecedented resolution. She has made significant strides in the development of computational tools and omics databases that address the challenges posed by the complexity, scalability, and accessibility of single-cell and spatial omics data.
Achievements and Recognition
Dr. Chen’s contributions have earned her numerous accolades, including being named an EMBO Global Investigator (2024-2027), a Highly Cited Researcher by Clarivate Analytics (2020-2023), and an A*STAR Fellow (2023-2026). Her work is also recognized in the SG 100 Women in Tech initiative.
Collaboration and Impact
Dr. Chen's work stands at the forefront of integrating AI with biological research, and her contributions are poised to make a lasting impact on the field of precision medicine, offering new tools and insights that are accessible to a wide range of researchers.
Beyond her publications and tool development, Dr. Chen is dedicated to sharing her findings and tools with the broader scientific community. The DISCO Data Hub and the ezSingleCell platform established by her lab offer open-access resources for single-cell and spatial omics. These platforms facilitate collaboration, data sharing, and analysis across institutions worldwide. Their global reach ensures that valuable data and tools are accessible to researchers everywhere, accelerating the pace of discovery.
Group Members
Scientist | ANG Kok Siong |
Scientist | LI Mengwei |
Scientist | XU Hang |
Scientist | LONG Yahui |
Scientist | KARIOTIS Sokratis |
Research Officer | SETHI Raman |
Research Officer | ZHONG Chengwei |
Research Officer | YE Shuchen |
PhD Student | LEE Nicole |
PhD Student | TAN Jia Chi |
PhD Student | CHUAH Samuel |
A*STAR celebrates International Women's Day
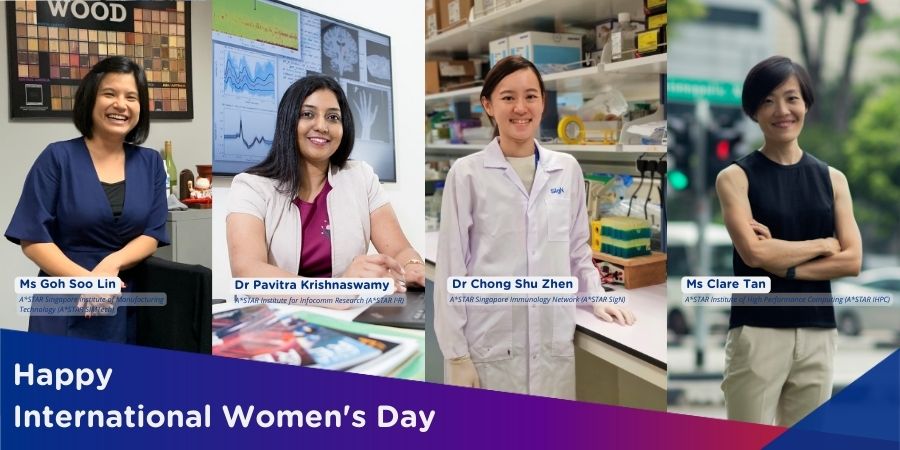
From groundbreaking discoveries to cutting-edge research, our researchers are empowering the next generation of female science, technology, engineering and mathematics (STEM) leaders.
Get inspired by our #WomeninSTEM