Health and Human Potential
- Health & Human Potential
- Manufacturing & Engineering
- Smart Nation & Digital Economy
- Transport & Connectivity
- Urban Solutions & Sustainability
AI Applications on Fundus Images
The eye is the window for human health and fundus images (i.e., colour photos of a person’s retina) contain a lot of information on a person’s well-being. Combining expert knowledge and Artificial Intelligence (AI) models, factual information can be extracted from the fundus images to diagnose an individual’s health conditions.
Working closely with the clinical team from the Singapore Eye Research Institute (SERI) under SingHealth, the IHPC team has developed AI algorithms and models for ophthalmology using multimodal data, including fundus photos, clinical risk factors, genetic data and OCT (Optical Coherence Tomography).
Applications on Vision Impairment
Current approaches in vision screening could only provide a high-level examination to opticians in the community. Hence a simple and efficient process is needed to identify individuals for prompt referral to tertiary eye care centres for vision loss related to potential eye diseases. The emergence of deep learning technology offers new opportunities to revolutionise this clinical referral pathway. Together, the team assesses the performance of a newly developed deep learning algorithm to detect disease-related visual impairment.
Applications on Myopic Macular Degeneration (MMD)
Myopic Macular Degeneration (MMD) is a type of macular degeneration that occurs in people with severe myopia. By 2050, almost 5 billion people globally are projected to have myopia, of whom 20% are likely to have high myopia with a clinically significant risk of sight-threatening complications such as MMD[1]. The diagnoses typically require a specialist’s assessment or measurement with multiple equipments. Deep learning approaches could be effective for risk stratification and identify individuals at the highest risk of visual loss.
Applications on Papilledema
Papilledema detection (generally known as optic-nerve edema from intracranial hypertension) and the ability to determine abnormal optic disk are essential in evaluating patients with headaches and other neurologic symptoms. Using artificial intelligence (AI) to detect papilledema and other optic-disk abnormalities from fundus photographs has not been well studied. IHPC team developed a deep-learning system that could aid in the diagnosis of optic-nerve abnormalities, particularly in papilledema detection from fundus photographs.
Publications
- Papilledema Detection (New England Journal of Medicine 2020, The Straits Times)
- Myopic Macular Degeneration (MMD) Detection (The Lancet Digital Health 2021)
- Visual Impairment Detection (The Lancet Digital Health 2021)
- Diabetic Macular Edema (DME) detection (MICCAI2019)
- Retinal Vessel Segmentation (OMIA, MICCAI2019)
Features
- Identify Visual impairment (VI), High myopia and MMD and optic nerve abnormality (papilledema) with high accuracy (i.e., at Area under the Curve, AUC from 0.94 to 0.98)
- Alert medical practitioners of the possibility of a severe brain condition
- Minimise the risk of overlook during an emergency assessment
- Allow early detection and treatment for slowing down or reversal of disease progression
The Science Behind
IHPC developed deep learning methodologies based on fundus images and trained multiple AI models for detection of multiple diseases.
a) Visual Impairment: In a proof-of-concept study, retinal fundus images with (complete data related to) best-corrected visual acuity or pinhole visual acuity were used. The team then developed a single-modality deep learning algorithm based on retinal photographs alone for the detection of any disease-related visual impairment.
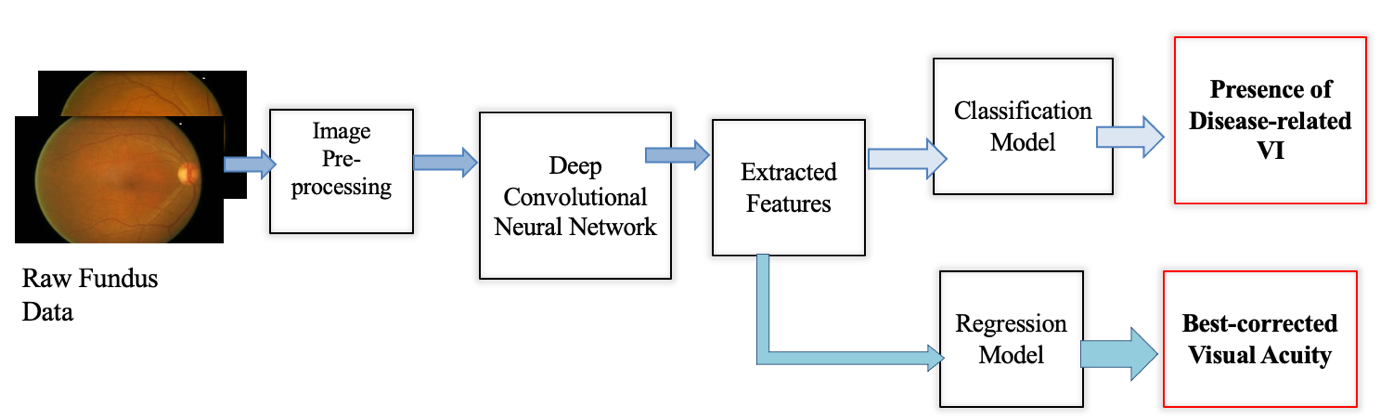
The architecture of AI model for Visual Impairment
b) MMD and High Myopia: Retinal images formed the input of the convolutional neural networks. The team derived the following outputs from three separate deep learning algorithms: (i) myopic macular degeneration, (ii) high myopia by spherical equivalent criteria, and (iii) high myopia by axial length criteria. The outputs of both model (ii) & (iii) were used as inputs to create a “combined high myopia model” for better detection performance.
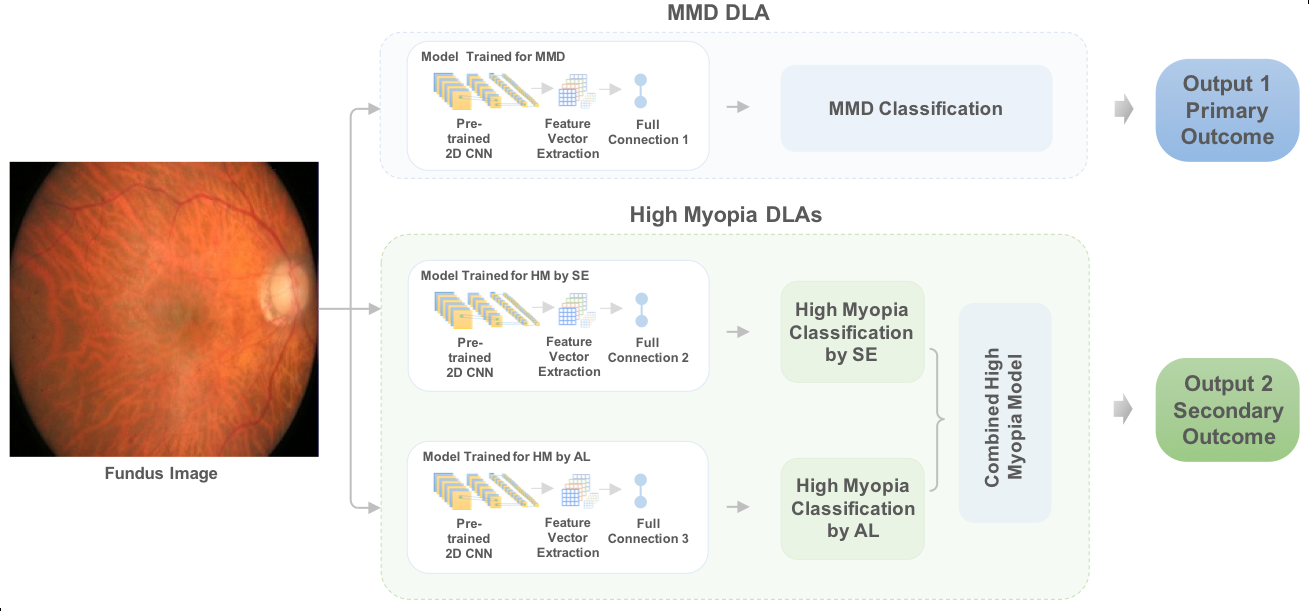
The architecture of AI model for MMD and High Myopia
c) Papilledema: Our system consisted of a segmentation network (U-Net) to detect the location of the optic disk from fundus photographs and a classification network (DenseNet) to classify the optic disk into one of the three classes: normal disk, disk with papilledema, and disk with other abnormalities.
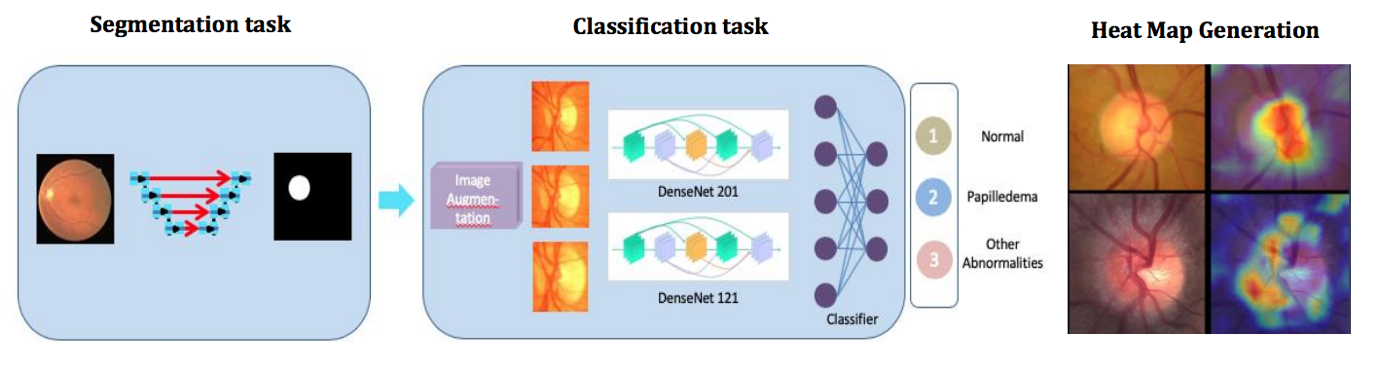
The architecture of AI model with segmentation and classification networks for Papilledema Detection
Industry Applications
With further validation, the deep learning algorithm for disease-related visual impairment, MMD and Papilledema detection could be deployed as an automated tool for identifying referable disease-related visual impairment in the community, allowing potentially timelier and more accurate referral of such cases to tertiary eye centres or as an aid for tertiary eye centres to speed up diagnosis. These could be effective screening tools for identifying the individuals with the highest risk of future complications, and such a strategy is likely to be important in tackling the global healthcare burden.
References
[1] Half the world to be short-sighted by 2050
For more info or collaboration opportunities, please write to enquiry@ihpc.a-star.edu.sg.
A*STAR celebrates International Women's Day
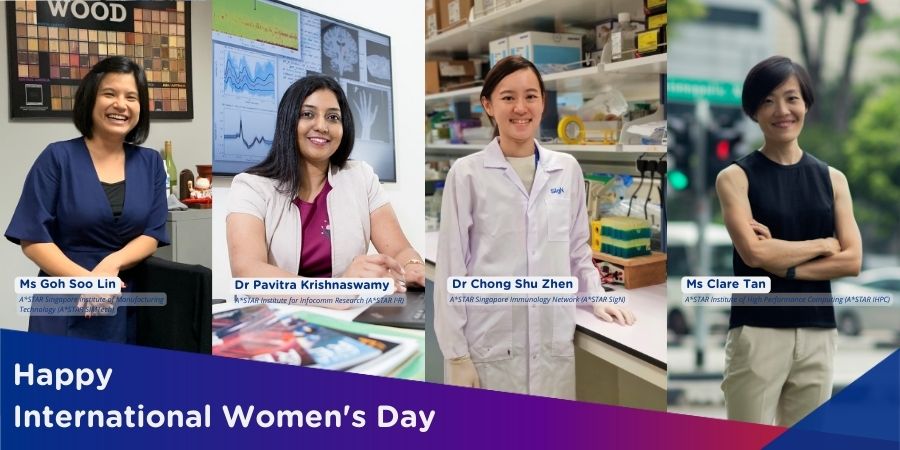
From groundbreaking discoveries to cutting-edge research, our researchers are empowering the next generation of female science, technology, engineering and mathematics (STEM) leaders.
Get inspired by our #WomeninSTEM