Digital Healthcare and MedTech
Background / Motivation
Health and Medical Technology is an important sector in Singapore that supports more than 9,000 jobs with 50 Regional Headquarters, 25 MNCs with R&D presence, over 250 MedTech start-ups and small-medium enterprises3. Increasingly, new approaches to innovation in this sector will require sustained R&D efforts to help medtech companies move up the value chain. The COVID-19 pandemic has also accelerated the shift of healthcare systems to the digitalised paradigm and is rapidly transforming how healthcare professionals and stakeholders will work together for enhanced resilience.
Capabilities
To deliver scientific and technological advancements that impact the Health and Medical Technology sector, IHPC has set up the Health and Medical Technology Innovation Technology Area (ITA) group to harness the strengths of multiple disciplines across different departments.
Health and Medical Technology is an important sector in Singapore that supports more than 9,000 jobs with 50 Regional Headquarters, 25 MNCs with R&D presence, over 250 MedTech start-ups and small-medium enterprises3. Increasingly, new approaches to innovation in this sector will require sustained R&D efforts to help medtech companies move up the value chain. The COVID-19 pandemic has also accelerated the shift of healthcare systems to the digitalised paradigm and is rapidly transforming how healthcare professionals and stakeholders will work together for enhanced resilience.
To deliver scientific and technological advancements that impact the Health and Medical Technology sector, IHPC has set up the Health and Medical Technology Innovation Technology Area (ITA) group to harness the strengths of multiple disciplines across different departments.
IHPC’s digital healthcare and medtech efforts include:
- Digital healthcare, especially in ophthalmology
- Health-tech for wellness and pre-disease screening
- Geriatric care
- Modelling and simulation for production and design of medical devices
Collaboration Opportunities
Leverage the span of technical expertise at IHPC for technology advancement to help your business move up the value chain.
We welcome interested party to collaborate in research and development relating to Digital Healthcare and MedTech in identifying new technology areas to develop new applications, multi-disciplinary projects or commercialisation outcomes.
For more info or collaboration opportunities, please write to enquiry@ihpc.a-star.edu.sg.
Leverage the span of technical expertise at IHPC for technology advancement to help your business move up the value chain.
We welcome interested party to collaborate in research and development relating to Digital Healthcare and MedTech in identifying new technology areas to develop new applications, multi-disciplinary projects or commercialisation outcomes.
For more info or collaboration opportunities, please write to enquiry@ihpc.a-star.edu.sg.
A*STAR celebrates International Women's Day
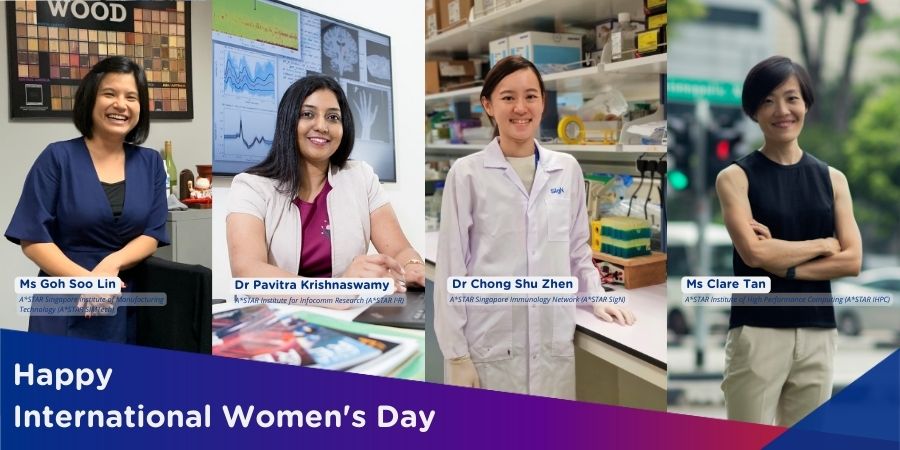
From groundbreaking discoveries to cutting-edge research, our researchers are empowering the next generation of female science, technology, engineering and mathematics (STEM) leaders.
Get inspired by our #WomeninSTEM