Knowledge-driven AI
Background / Motivation
The past decade has seen tremendous advances in machine learning (ML) and artificial intelligence (AI), especially in the application of deep learning to natural language processing and computer vision. This data revolution has led to massive productivity in ways such as accelerated materials development cycles, improved predictive maintenance in industries such as aerospace, and enhanced process optimisation in advance manufacturing.
However, perennial concerns remain over some fundamental limitations in conventional ML: (i) the requirement for large and typically expensive-to-acquire datasets; and (ii) a lack of generalisability and interpretability. A potential solution to alleviate these limitations is to incorporate knowledge with AI. Such knowledge-driven AI (K-AI) can comprise both scientific and human-centric knowledge.
IHPC, with its expertise in engineering and numerical theory-based simulation, cognitive and social sciences, and machine learning, is in a unique position to combine deep domain knowledge in areas such as physics, chemistry and social sciences with novel AI models and methods to create greater impact across different domains.
Capabilities
The past decade has seen tremendous advances in machine learning (ML) and artificial intelligence (AI), especially in the application of deep learning to natural language processing and computer vision. This data revolution has led to massive productivity in ways such as accelerated materials development cycles, improved predictive maintenance in industries such as aerospace, and enhanced process optimisation in advance manufacturing.
However, perennial concerns remain over some fundamental limitations in conventional ML: (i) the requirement for large and typically expensive-to-acquire datasets; and (ii) a lack of generalisability and interpretability. A potential solution to alleviate these limitations is to incorporate knowledge with AI. Such knowledge-driven AI (K-AI) can comprise both scientific and human-centric knowledge.
IHPC, with its expertise in engineering and numerical theory-based simulation, cognitive and social sciences, and machine learning, is in a unique position to combine deep domain knowledge in areas such as physics, chemistry and social sciences with novel AI models and methods to create greater impact across different domains.
IHPC’s K-AI efforts (Fig 1) include:
Knowledge-Guided Forward Modelling - Integrating domain knowledge with AI for forward prediction
- Hybridisation of AI and Simulation
- Knowledge-guided design of AI model architecture
- Knowledge-constrained training of AI models
Knowledge-Guided Inverse Design - Utilising domain knowledge during optimisation to achieve optimal design solutions and/or to accelerate the design process
- Knowledge-guided design of algorithms for more optimal search space exploration
- Knowledge-guided reduction of search space for faster optimisation
Knowledge-Guided Representation Learning - Utilising domain knowledge to better acquire and represent information for incorporation into the AI models for both forward modelling and inverse design
- Knowledge-guided data representation for improved feature construction and selection for AI models
- Strategies for knowledge acquisition and extraction from domain experts or indirect sources of information (e.g., reasoning for general expertise)
Fig 1. IHPC's Research Foci within K-AI
Collaboration Opportunities
IHPC welcomes interested parties to collaborate in research and development relating to Artificial Intelligence and Quantum Computing, especially in identifying new technology areas to develop new applications, multi-disciplinary projects or commercialisation outcomes. Leverage our domain expertise and K-AI framework to solve interesting problem statements.
For more info or collaboration opportunities, please write to enquiry@ihpc.a-star.edu.sg.
IHPC welcomes interested parties to collaborate in research and development relating to Artificial Intelligence and Quantum Computing, especially in identifying new technology areas to develop new applications, multi-disciplinary projects or commercialisation outcomes. Leverage our domain expertise and K-AI framework to solve interesting problem statements.
For more info or collaboration opportunities, please write to enquiry@ihpc.a-star.edu.sg.
A*STAR celebrates International Women's Day
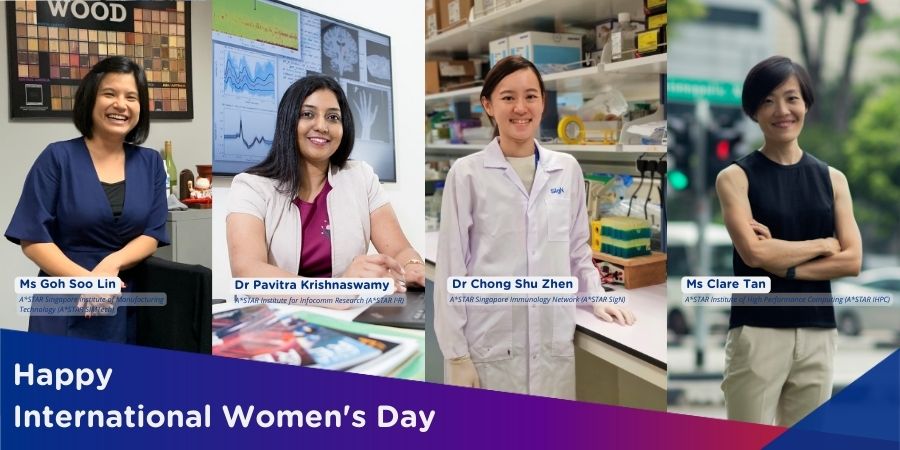
From groundbreaking discoveries to cutting-edge research, our researchers are empowering the next generation of female science, technology, engineering and mathematics (STEM) leaders.
Get inspired by our #WomeninSTEM