Data Analysis Tool Empowers the People
Data production doubles each year, but data scientists, who wrangle insights from reams of data, are in short supply. To bridge this gap, a team at A*STAR has developed a fully automatic, web-based system that puts the power of big data analysis in the hands of laypeople.
Uncovering patterns and relationships hidden in vast data sets requires a machine learning pipeline or ‘workflow’ — a string of algorithms and processes called operators. But not every workflow is appropriate for every situation. So how does the non-expert know which to use? To help, Theint Theint Aye, from the A*STAR Institute of High Performance Computing and her colleagues have produced an analytics system (called the Layman Analytics System, or ‘LAS’) for the novice.
Say you have a data set to analyse. The first part of the LAS — the workflow recommender — compares your data set’s metadata to that of existing data sets in a repository. It then selects the best-performing workflows based on those similar repository data sets and passes them to the second part: the workflow optimizer.
Here, ‘genetic programming’ refines the workflow. Operators are randomly replaced, analogous to random genetic mutations in DNA. Mutated workflows are then crossed with each other, which involves swapping pairs of operators between them.
The process then repeats — ‘fittest’ workflows are selected, mutated and crossed — for a predefined number of generations (based on empirical experience). The result: an automatically generated tailor-made workflow.
The system is web based using cloud infrastructure, so there is no need to install special software or use dedicated computing power.
To evaluate whether the LAS generated appropriate workflows, Aye’s team tested it on 114 data sets from the University of California’s Irvine Machine Learning Repository and benchmarked against OpenML, an open-source, online machine learning platform.
For 87 data sets (about 76 per cent of the total), LAS-produced workflow accuracy was above the 50th percentile of OpenML’s performance. This figure could improve over time too, Aye says. Users can plug their data sets and workflows back into the repository, providing a richer stock from which the workflow recommender can later draw.
Non-experts usually take days to generate a good workflow, however, in LAS, the average time to produce a workflow in 15 generations was just over 3 hours. In the future, implementing a faster search technique, or heuristic, could further cut processing time. “Obviously, we would want to run it as efficiently as possible and also have good accuracy values,” Aye says, adding that a graphics processing unit might also boost the LAS’s speed.
Uncovering patterns and relationships hidden in vast data sets requires a machine learning pipeline or ‘workflow’ — a string of algorithms and processes called operators. But not every workflow is appropriate for every situation. So how does the non-expert know which to use? To help, Theint Theint Aye, from the A*STAR Institute of High Performance Computing and her colleagues have produced an analytics system (called the Layman Analytics System, or ‘LAS’) for the novice.
Say you have a data set to analyse. The first part of the LAS — the workflow recommender — compares your data set’s metadata to that of existing data sets in a repository. It then selects the best-performing workflows based on those similar repository data sets and passes them to the second part: the workflow optimizer.
Here, ‘genetic programming’ refines the workflow. Operators are randomly replaced, analogous to random genetic mutations in DNA. Mutated workflows are then crossed with each other, which involves swapping pairs of operators between them.
The process then repeats — ‘fittest’ workflows are selected, mutated and crossed — for a predefined number of generations (based on empirical experience). The result: an automatically generated tailor-made workflow.
The system is web based using cloud infrastructure, so there is no need to install special software or use dedicated computing power.
To evaluate whether the LAS generated appropriate workflows, Aye’s team tested it on 114 data sets from the University of California’s Irvine Machine Learning Repository and benchmarked against OpenML, an open-source, online machine learning platform.
For 87 data sets (about 76 per cent of the total), LAS-produced workflow accuracy was above the 50th percentile of OpenML’s performance. This figure could improve over time too, Aye says. Users can plug their data sets and workflows back into the repository, providing a richer stock from which the workflow recommender can later draw.
Non-experts usually take days to generate a good workflow, however, in LAS, the average time to produce a workflow in 15 generations was just over 3 hours. In the future, implementing a faster search technique, or heuristic, could further cut processing time. “Obviously, we would want to run it as efficiently as possible and also have good accuracy values,” Aye says, adding that a graphics processing unit might also boost the LAS’s speed.
A*STAR celebrates International Women's Day
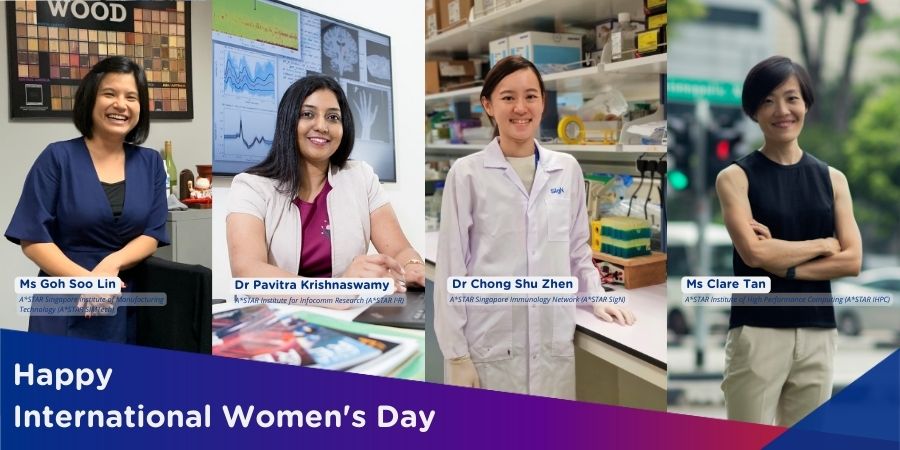
From groundbreaking discoveries to cutting-edge research, our researchers are empowering the next generation of female science, technology, engineering and mathematics (STEM) leaders.
Get inspired by our #WomeninSTEM