Early dengue outbreak detection modeling based on dengue incidences in Singapore during 2012 to 2017
Dengue is endemic in Singapore, and there were several severe outbreaks of dengue in 2012 to 2017; during dengue outbreaks, there is rapid and consecutive increases of dengue incidences that deviate from the normal endemic pattern. Reliable early warnings of impending dengue outbreaks provide windows of opportunity to adopt or ramp up intervention measures mitigating against disease outbreak and any consequent challenge to public health resources, without wasting public resources due to false alarms. Other than outbreaks during which the spread of dengue was rapid and large-scale, MOH's historical data of weekly dengue counts also show the presence of transient large weekly case counts, i.e. spikes in weekly case count that rapidly decreases to a low case count.
A commonly-used quantitative definition of an outbreak is dengue incidence exceeding a threshold value computed from the mean and standard deviation of the number of dengue cases over a constant number of years immediately preceding the point in time of interest. Superimposing such a computed threshold on MOH's historical data showed that using the computed threshold as an outbreak prediction method would erroneously flag some of the transient large case counts as outbreaks.
This work innovatively proposed to predict dengue outbreaks by using statistical control charts which are extensively used within manufacturing to detect defective products; statistical control charts detect "out of control" events using both the absolute value of the data point (e.g. whether the data point falls outside of a threshold), as well as the trend indicated by the immediate preceding data points (e.g. a group of data points showing rapid and consecutive increases).
A key breakthrough in this work is the proposal of a two-step framework to accommodate the differing characteristics of disease surveillance data relative to industrial data. Analysis of Singapore's weekly dengue cases in 2012 to 2017 using statistical control charts successfully identified the actual dengue outbreaks, but did not flag the transient high case counts as impending outbreaks. To the best of our knowledge, this is the first time statistical control charts is proposed for prediction of dengue outbreaks. The proposed two-step framework is quite general, and if there is sufficient historical data available to construct the statistical control charts, this two-step framework should work well in predicting outbreaks of other diseases such as salmonellosis, malaria, and influenza.
This work documents the successful application of IHPC's predictive modelling capabilities outside of the traditional manufacturing-related use cases to support the national agenda. MOH had expressed interest to further evaluate this early outbreak detection model for practical use, though unfortunately these plans have been delayed due to COVID-19. After the completion of the initial framework, the team has further verified the proposed early outbreak detection model with real-time data (versus the historical data studied in the initial phase), and is developing an online version of the early outbreak detection model. The early outbreak detection model will be integrated with at-risk spatial-temporal locations forecasting for targeted and proactive dengue control; research for at-risk location forecasting is currently ongoing. IHPC also plans to demonstrate the use cases of our models on the Virtual Singapore platform.
A*STAR celebrates International Women's Day
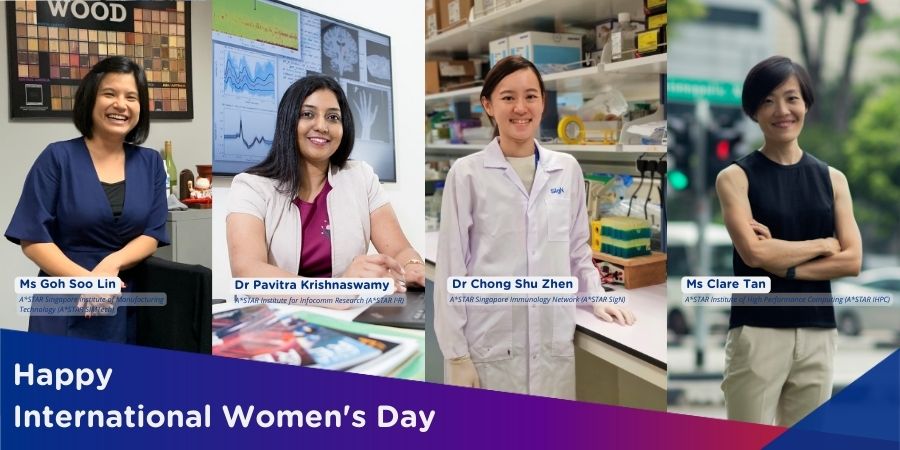
From groundbreaking discoveries to cutting-edge research, our researchers are empowering the next generation of female science, technology, engineering and mathematics (STEM) leaders.
Get inspired by our #WomeninSTEM