Reducing Commuters’ Wait Time
Waiting endlessly for a bus to arrive may soon be a thing of the past — a method for analyzing the flow of passengers on public bus networks, developed by researchers at A*STAR, could enable schedule optimization and minimize waiting times.
Global positioning systems have long enabled real-time estimations of the arrival time of buses on many of the world’s public transport networks. When this automated vehicle location (AVL) data is combined with passenger information — known as automated passenger collection (APC) data and usually collected as the passenger scans their tickets — an intricate picture of human motion across a metropolitan area can be compiled. This information, in turn, can be harnessed to improve public transport.
One useful metric by which public transport systems are assessed is the average time a commuter must wait for their bus. Waiting times can be recorded manually or by video, but these methods are intrinsically very limited in scale. Instead, researchers have developed mathematical formulae that try to model average waiting times from the so-called ‘headway time’, the time until the next bus, based on AVL data. But this approach requires several unverified assumptions. For example, it assumes that for high frequency services, commuters arrive at the bus stop uniformly.
Muhamad Azfar Ramli from A*STAR’s Institute of High Performance Computing and collaborators from a local bus company have analysed AVL and APC data from ten bus routes in Singapore over one month. They showed that the correlation between the number of commuters boarding and the corresponding headway is poor, thus indicating that the uniform arrival assumption is incorrect in Singapore. The team then used this data to build an accurate simulation, which was able to accurately reproduce the empirical data gathered in Singapore in August 2014.
With the accuracy and efficiency of their model confirmed, the researchers were able to use the technique to propose schedules that would minimize the average commuter waiting time without necessarily imposing more buses on the network.
“We hope that our work lays the foundation for more dynamic and responsive transport operations that can be reactive not only to changes in bus locations and movements but also to commuting demand,” says Ramli. At present, the analysis can only be done a day or two after data collection, but the team believes this can be done faster. “We hope that when both our estimation techniques and the available real-time data streaming technologies have improved, our methodologies can be applied in real-time and enable operators to react more accurately to dynamic changes in service quality.”
A*STAR celebrates International Women's Day
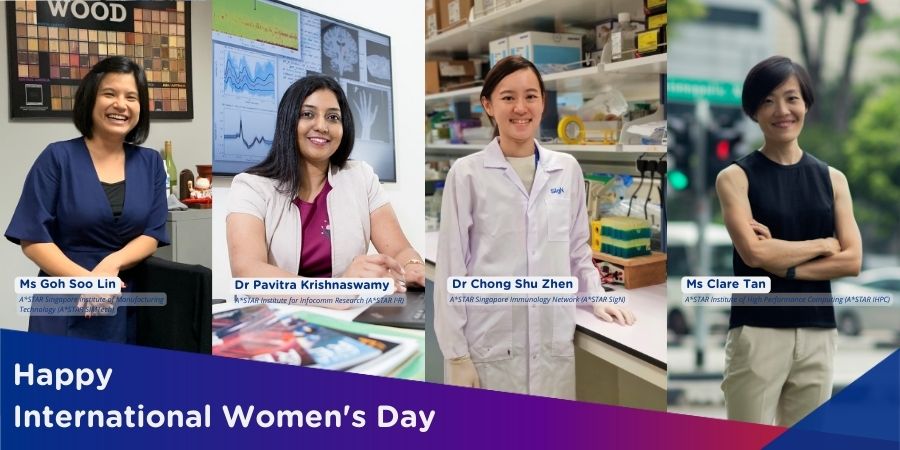
From groundbreaking discoveries to cutting-edge research, our researchers are empowering the next generation of female science, technology, engineering and mathematics (STEM) leaders.
Get inspired by our #WomeninSTEM