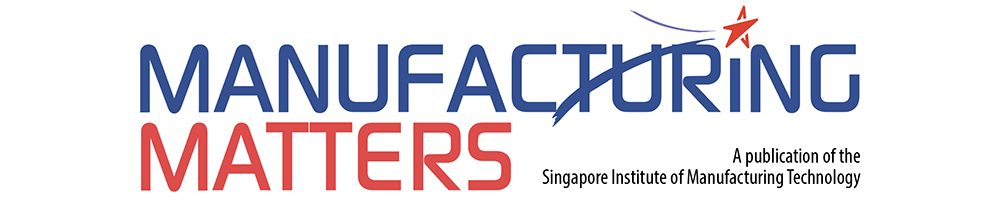
- Press Releases
- Industry Updates
- SIMTech Manufacturing Matters
- Manufacturing Matters
- SIMTech 30th Anniversary
- Events
- ASPEN2022
- BCA Green Mark (Platinum)for Healthier Workplaces and Laboratories
Is Your Artificial Intelligence Model Safe for Deployment?
A black-box optimisation software library for rigorous testing of AI
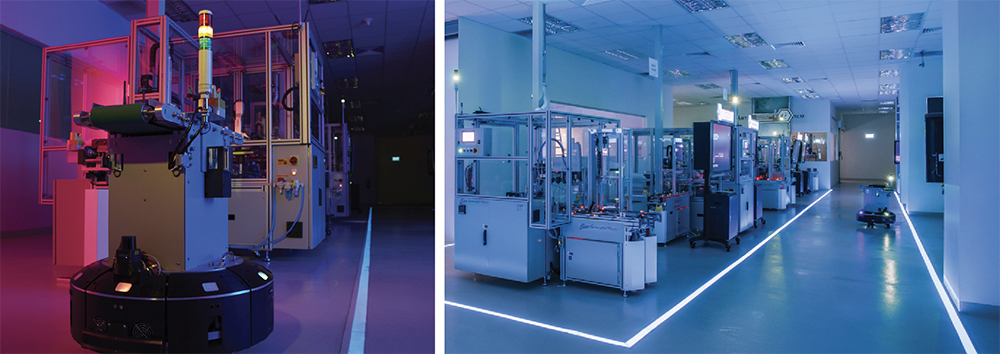
Having transformed industries in retail and advertising, the potential of artificial intelligence (AI) in boosting productivity of manufacturing processes is gaining attention worldwide. From cyber-physical production systems to smart robotics, AI-enabled digital technologies have begun to proliferate all stages of the manufacturing pipeline – spanning automated shop floor operations, enterprise planning and supply chain decision support.
Advances in deep reinforcement learning (DRL) has been one of the key drivers stimulating the widespread adoption of AI, making it possible to train artificial agents to independently act in uncertain environments. However – despite many success stories in controlled laboratory experiments – DRL models are yet to be rigorously tested against the unknowns of the real-world. Anticipatory analyses of AI’s decisions in unforeseen circumstances are thus necessary to ensure trustworthy deployment of such models in safety-critical applications. Of particular relevance to ongoing research are scenarios where an intelligent adversary may calculatedly alter an AI agent’s inputs with the deliberate intent of causing harm (see Figure 1).
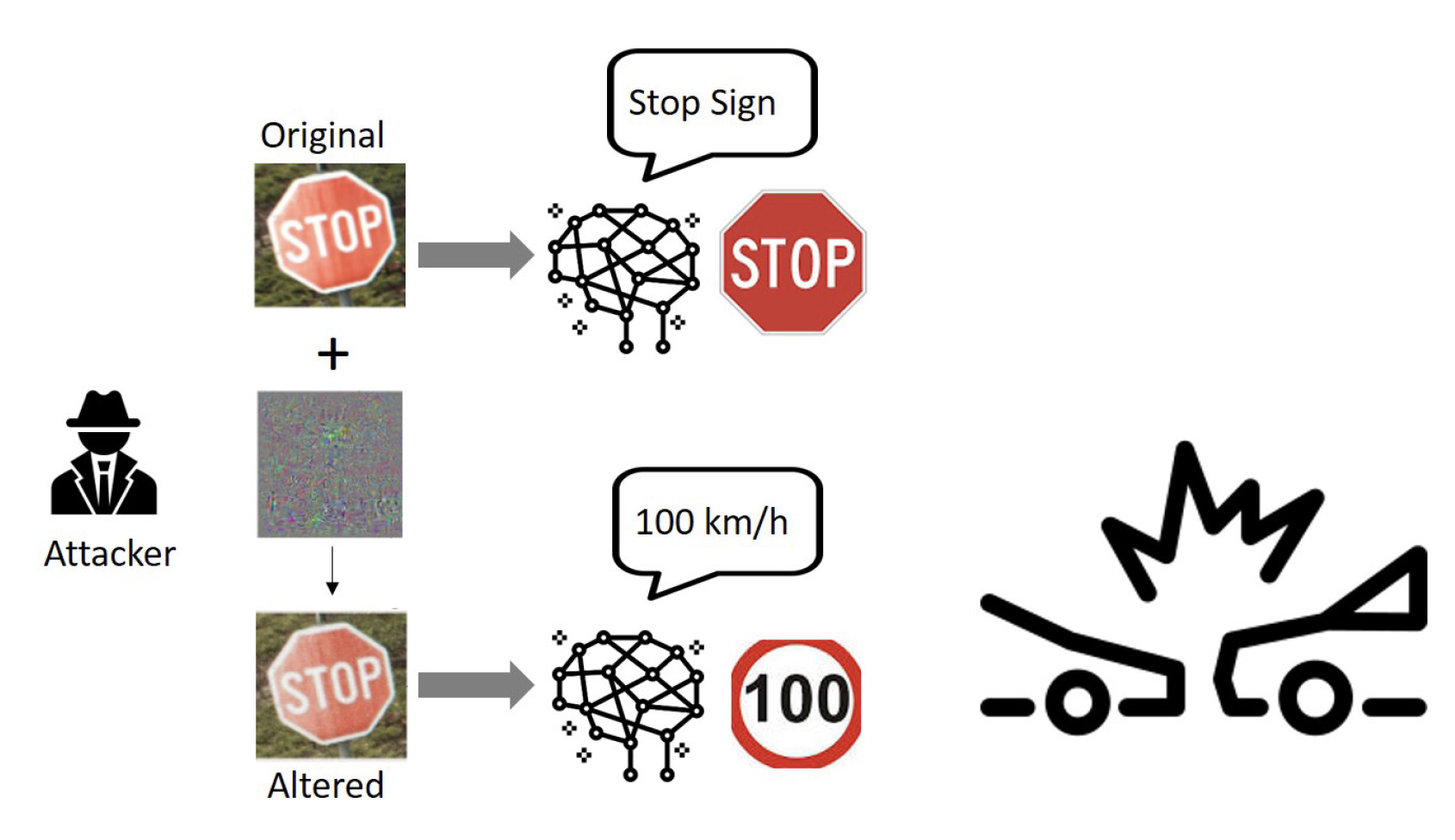
Although cutting-edge AI has focused on achieving models of high accuracy, the robustness of these models must be tested before they are put into operational use, so as to ensure the integrity & reliability of AI deployment.
Through a collaboration between NTU and SIMTech, it has recently been revealed that many state-of-the-art DRL algorithms are not as reliable as widely expected. The study focused on assessing the vulnerability of deep learning techniques in prescribing sequences of actions to be taken by vision-based AI agents, such as robots or autonomous vehicles with on-board camera sensors. The analysis was formulated as a black-box optimisation problem, with the objective of quickly identifying sensitive pixels that have the most significant impact on an agent’s performance. Stunningly, the team found that a mere one-pixel change to an agent’s sensory inputs was often sufficient to severely degrade its performance. What is more, the AI’s sensitivity to changes in certain pixels was found to persist across similar input frames. The result indicated that even though DRL seems to thrive in familiar, standardised environments, there are inherent weaknesses in its performance against external variability – potentially to the detriment of safety in the presence of an adversary.
The computational studies carried out during the course of the research have led to a suite of software libraries and capabilities for rigorous testing of AI across applications.
As an attestation of the scientific novelty and impact of the work, articles reporting the aforementioned findings have been published in leading journals such as the IEEE Transactions on Cybernetics and the IEEE Transactions on Cognitive and Developmental Systems. The work has also been recognised as an A*STAR Research Highlight in June 2020.
For more information, please contact Dr Abhishek Gupta,
Planning & Operations Management Group at abhishek_gupta@SIMTech.a-star.edu.sg
A*STAR celebrates International Women's Day
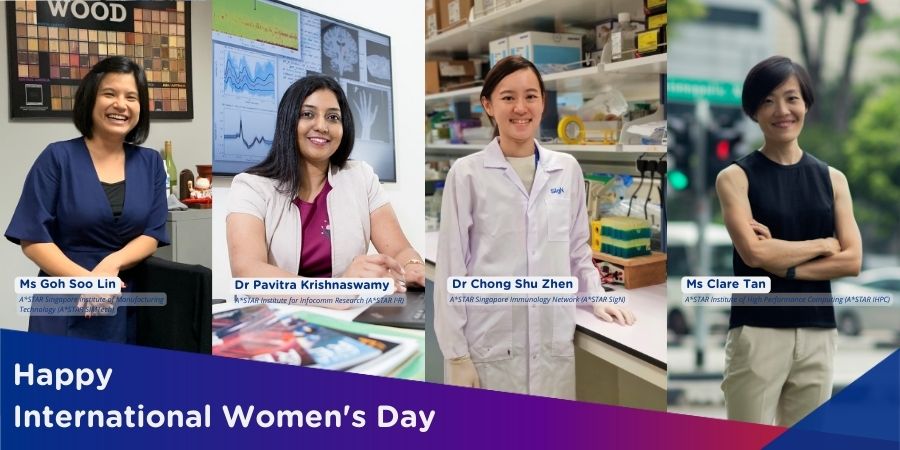
From groundbreaking discoveries to cutting-edge research, our researchers are empowering the next generation of female science, technology, engineering and mathematics (STEM) leaders.
Get inspired by our #WomeninSTEM