Artificial Intelligence (AI) at GIS
Advancements in AI technology and their application to comprehensive datasets for data prediction, classification, simulation, or modeling, represent the next frontier of genomics. With the nature of biological data which can be large, complex, heterogenous, and ever-changing due to evolution, AI bioinformatics tools have the potential to enhance analysis speed and generate valuable insights for the development of research.
At GIS, we complement our computational research in genomics with AI. This research includes, but is not limited to:
- Analysis of DNA/RNA sequencing data and biomolecular structures for applications on read type detection, de novo assembly, DNA/RNA modifications, RNA folding, etc
- Application on cancer research to improve treatments, discovery of new drugs and biomarkers
- Metagenomic identification and analysis of novel species, virulence resistance determinants
- Cancer drug response prediction, microbial surveillance in healthcare environments
- Microbial surveillance in healthcare environments and antimicrobial resistance prediction
- Analysis of transcriptional regulation using epigenomics data and identification of prognostic makers and drug targets
- Development of machine-learning open-source software that improves the analysis of third generation RNA-sequencing data
- Generative modeling of underlying biological processes
- Image-integrated analysis of spatial transcriptomics data
- Graph-based methods for learning and inference on spatial-omics data (eg, spatial cell clustering)
- Mathematical optimisation and feature selection for transcriptomics (eg, DUBStepR)
- Uncertainty quantification and predictive modeling of genetic networks
- R Vaser, M Šikić. "Time-and memory-efficient genome assembly with Raven" Nature Computational Science, 1-5. 2021
- G Zhu, et al. "Tissue-specific cell-free DNA degradation quantifies circulating tumor DNA burden." Nature communications 12.1 (2021): 1-11.
- Yeo, G.H.T., Saksena, S. D., & Gifford, D. K. (2021). “Generative modeling of single-cell time series with PRESCIENT enables prediction of cell trajectories with interventions.” Nature Communications, 12(1), 3222.
- B Ranjan, WJ Sun, JY Park, K Mishra, R Xie, F Alipour, V Singhal, F Schmidt, I Joanito, NA Rayan, M.G.L Lim, S Prabhakar. (2021) “DUBStepR: correlation-based feature selection for clustering single-cell RNA sequencing data.” bioRxiv 2020.10.07.330563; doi: https://doi.org/10.1101/2020.10.07.330563
- N Baumgarten*, D Hecker*, S Karunanithi*, F Schmidt, M List, MH Schulz “EpiRegio: analysis and retrieval of regulatory elements linked to genes” Nucleic Acids Research, May 2020
- L Vrček, P Veličković, M Šikić. "A step towards neural genome assembly" arXiv preprint arXiv:2011.05013. 2020
- MJ Mason, C Schinke, CLP Eng, et al. “Multiple Myeloma DREAM Challenge reveals epigenetic regulator PHF19 as marker of aggressive disease.” Leukemia. 2020;34(7):1866-1874. doi:10.1038/s41375-020-0742-z
- PN Pratanwanich, F Yao, Y Chen, CWQ Koh, C Hendra, P Poon, YT Goh, et al. “Detection of differential RNA modifications from direct RNA sequencing of human cell lines” 2020. bioRxiv
- W Huang, et al. "SMuRF: portable and accurate ensemble prediction of somatic mutations." Bioinformatics 35.17 (2019): 3157-3159.
- C Suphavilai, D Bertrand, N Nagarajan. "Predicting cancer drug response using a recommender system." Bioinformatics 34.22 (2018): 3907-3914.
- KMK Lim, et al. "@ MInter: automated text-mining of microbial interactions." Bioinformatics 32.19 (2016): 2981-2987.
A*STAR celebrates International Women's Day
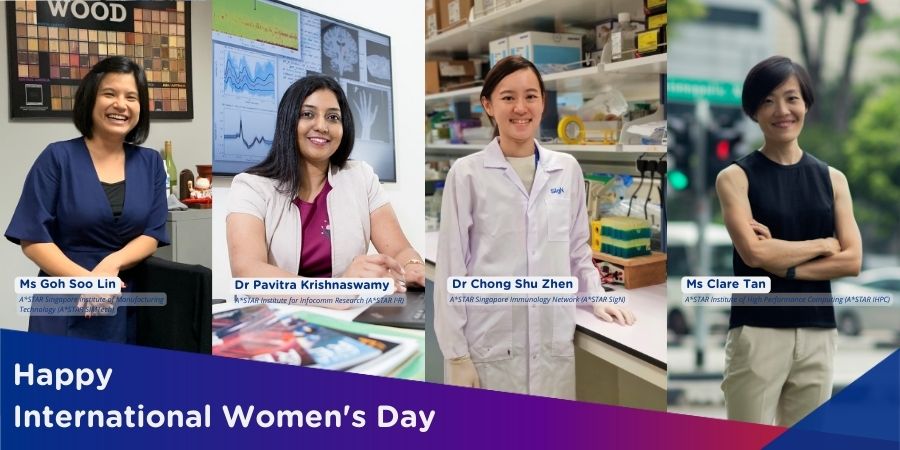
From groundbreaking discoveries to cutting-edge research, our researchers are empowering the next generation of female science, technology, engineering and mathematics (STEM) leaders.
Get inspired by our #WomeninSTEM