VarNet
Name of software | VarNet |
Purpose | Somatic mutation caller using weakly supervised deep learning |
Name of Contact | Kiran Krishnamachari & Anders Skanderup |
Email of technical contact | kiran_krishnamachari@gis.a-star.edu.sg skanderupamj@gis.a-star.edu.sg |
Summary of software function | Identification of somatic mutations in tumor samples is commonly based on statistical methods in combination with heuristic filters. Here we develop VarNet, an end-to-end deep learning approach for identification of somatic variants from aligned tumor and matched normal DNA reads. VarNet is trained using image representations of 4.6 million high-confidence somatic variants annotated in 356 tumor whole genomes. We benchmark VarNet across a range of publicly available datasets, demonstrating performance often exceeding current state-of-the-art methods. Overall, our results demonstrate how a scalable deep learning approach could augment and potentially supplant human engineered features and heuristic filters in somatic variant calling. VarNet is freely available for academic use. Commercial usage requires a license. |
Publications describing software & its application | Krishnamachari, K., Lu, D., Swift-Scott, A. et al. Accurate somatic variant detection using weakly supervised deep learning. Nat Commun 13, 4248 (2022).https://doi.org/10.1038/s41467-022-31765-8
|
A*STAR celebrates International Women's Day
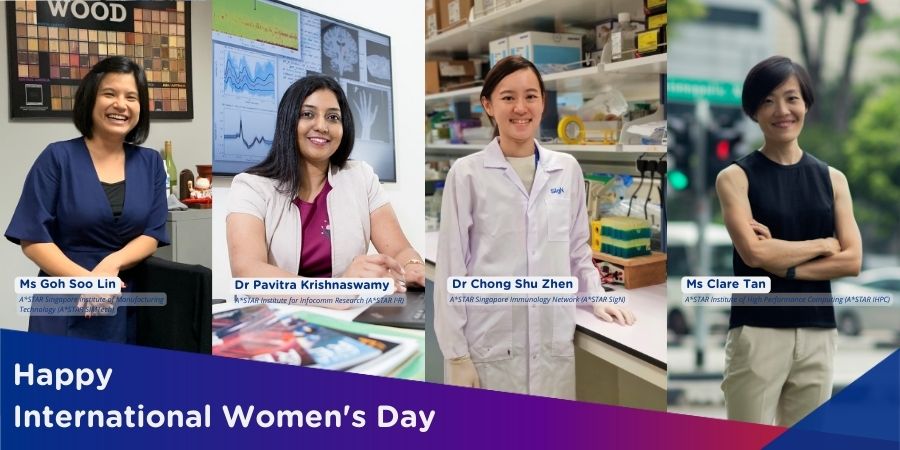
From groundbreaking discoveries to cutting-edge research, our researchers are empowering the next generation of female science, technology, engineering and mathematics (STEM) leaders.
Get inspired by our #WomeninSTEM