Mile SIKIC
RESEARCH
LinkedIn: https://www.linkedin.com/in/milesikic/X: https://twitter.com/msikic
We are a group of computer scientists dedicated to advancing the field of genomics through the development of cutting-edge AI and classical algorithms. Our primary objective is to decode the language of DNA and RNA and leverage this knowledge to pioneer new AI innovations inspired by genomics. Our focus areas include:
A) DNA/RNA Foundation Models
Our mission is to craft robust DNA and RNA foundation models, meticulously fine-tuning them to excel in various downstream tasks. These tasks include predicting RNA structural features, detecting modifications in DNA or RNA nucleotides, and classifying species within metagenomics samples. To achieve these goals, we harness a wide range of data inputs, including DNA and RNA sequences, as well as raw sequenced signals.
B) De Novo Assembly of Complex Genomes
We take pride in developing AI models and classical algorithms for strings and graphs related to de novo assembly. We aim to revolutionize and expedite the de novo assembly methods for both single genomes and metagenomes. Notably, we are pioneers in the utilization of graph neural networks for de novo assembly. Our ambitions extend to creating innovative methodologies for assembling polyploid and cancer genomes.
C) RNA Structure Prediction
We aim to accurately predict RNA structures and assess their druggability by harnessing state-of-the-art AI techniques. Our toolkit includes models inspired by Alphafold, large language models, and diffusion models, all combined with high-throughput experimental data.
D) Genomics Analytics
Beyond our innovative method development, we collaborate closely with experts in genomics to undertake critical genomics analyses. These collaborations involve de novo assembly of reference genomes, constructing pangenomes, and in-depth analysis of metagenomes, epigenomes, and RNA-sequenced data.
Selected Publications
- Šikić, M “Facilitating genome structural variation analysis Nature Methods” 20 (4), 491-492, 2023 Abstract
- Stanojević D, Li Z, Foo R, Šikić, M “Rockfish: A Transformer-based Model for Accurate 5-Methylcytosine Prediction from Nanopore Sequencing” bioRxiv, 2022.11. 11.513492 ” Abstract
- Vaser R, Šikić M “Time-and memory-efficient genome assembly with Raven” Nature Computational Science 1 (5), 332-336, 2021 Abstract
- Vrček L, Veličković P, Šikić M “A step towards neural genome assembly”, NeuroIPS 2020 Abstract
- Šošić M, Šikić M "Edlib: a C/C ++ library for fast, exact sequence alignment using edit distance." Bioinformatics 2017 05 01 ; 33(9) : 1394-1395 Abstract
- Vaser R, Sović I, Nagarajan N, Šikić M "Fast and accurate de novo genome assembly from long uncorrected reads." Genome Res 2017 05 ; 27(5) : 737-746 Abstract
- Sović I*, Šikić M*, Wilm A, Fenlon SN, Chen S, Nagarajan N "Fast and sensitive mapping of nanopore sequencing reads with GraphMap." Nat Commun 2016 04 15 ; 7 : 11307 *These authors contributed equally Abstract
- Vaser R, Adusumalli S, Leng SN, Sikic M, Ng PC "SIFT missense predictions for genomes." Nat Protoc 2016 01 ; 11(1) : 1-9 Abstract
- Šikić M, Tomić S, Vlahovicek K "Prediction of protein-protein interaction sites in sequences and 3D structures by random forests." PLoS Comput Biol 2009 01 ; 5(1) : e1000278 Abstract
A*STAR celebrates International Women's Day
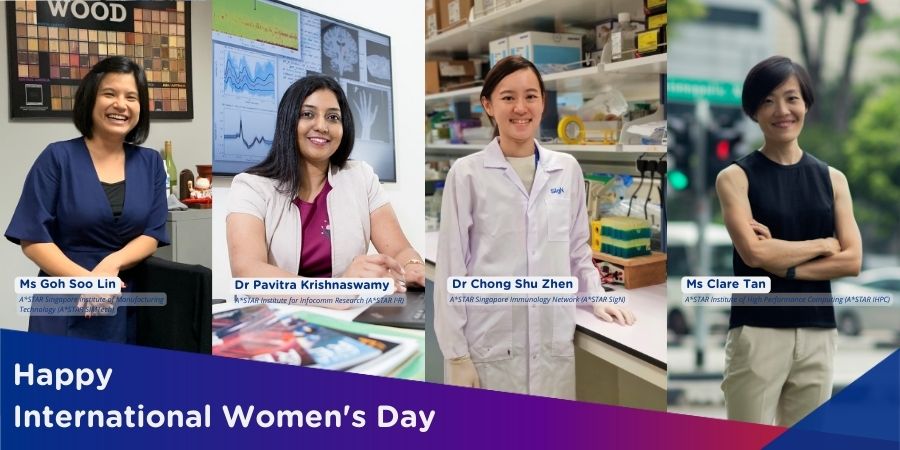
From groundbreaking discoveries to cutting-edge research, our researchers are empowering the next generation of female science, technology, engineering and mathematics (STEM) leaders.
Get inspired by our #WomeninSTEM