Advanced Spatial Omics with Quantum and Generative AI
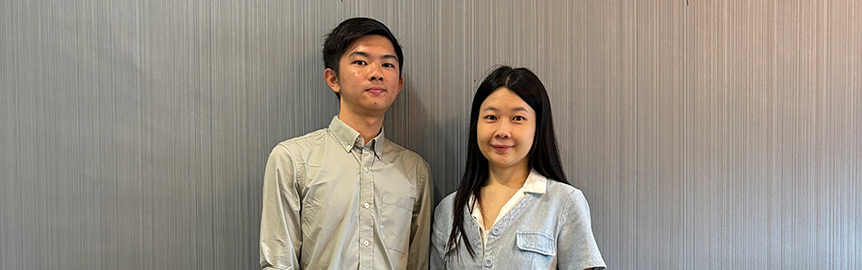
LAU MAI CHAN
![]() | LAU Mai Chan Senior Scientist Email: Lau_Mai_Chan@bii.a-star.edu.sg Research Group: Advanced Spatial Omics with Quantum and Generative AI |
Mai Chan Lau earned her PhD from the National University of Singapore in 2015, focusing on high-performance GPU research. She began her postdoctoral training at the Singapore Immunology Network (ASTAR), focusing on single-cell immunology bioinformatics. Following this, she moved to Boston, USA, where she leveraged AI and machine learning for Molecular Pathological Epidemiology (MPE) studies, using tissue-based multi-marker technology at Dana-Farber Cancer Institute, Brigham and Women’s Hospital, and Harvard Medical School. In 2021, she returned to Singapore and joined the Institute of Molecular and Cell Biology (ASTAR), expanding her research to spatial transcriptomics and AI. In November 2022, she established her own laboratory at the Bioinformatics Institute (ASTAR), concentrating on advanced spatial multi-omics studies using AI and quantum computing. Concurrently, she leads the Computational Immunology Platform at the Singapore Immunology Network (ASTAR). She served as a program committee member for Clinical Translation of Medical Image Computing & Computer-Assisted Intervention (CLINICCAI) during 2022-2024. She holds an editorial board position for the World Scientific Annual Review of Cancer Immunology journal.
GROUP MEMBERS
Research officer | TAN Wei Kit |
A*STAR celebrates International Women's Day
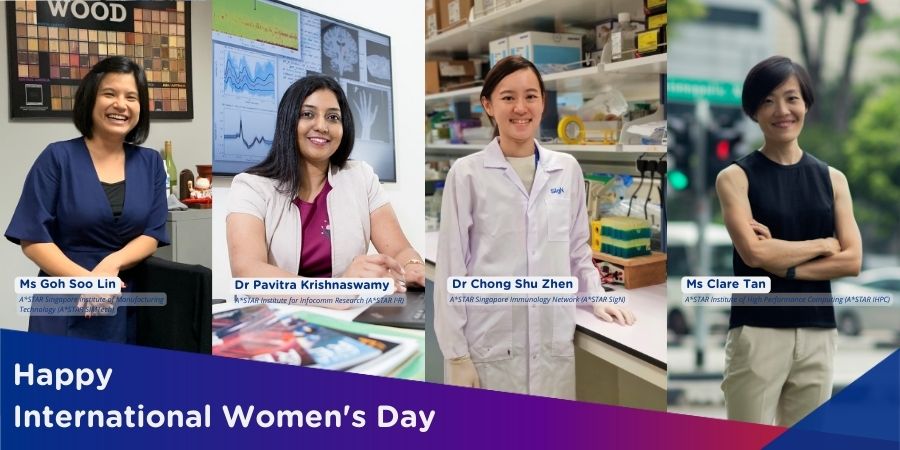
From groundbreaking discoveries to cutting-edge research, our researchers are empowering the next generation of female science, technology, engineering and mathematics (STEM) leaders.
Get inspired by our #WomeninSTEM